Generative Adversarial Domain Generalization via Cross-Task Feature Attention Learning for Prostate Segmentation.
ICONIP(2021)
摘要
In real-life clinical deployment, domain shift on multi-site datasets is a critical and crucial problem for the generalization of prostate segmentation deep neural networks. To tackle this challenging problem, we propose a new Generative Adversarial Domain Generalization (GADG) network, which can achieve the domain generalization through the generative adversarial learning on multi-site prostate MRI images. Specifically, we compose a cascaded generation-segmentation “U-Net" shaped network, first we generate a new image with same structural but different style information to the source image via the generation path (i.e. one branch of “U"), then the domain discriminator is used to determine whether the generated image is true or false via the domain label classification. To make the prostate segmentation network (i.e. the other branch of “U") learned from the source domains still have good performance in the target domain, a Cross-Task Attention Module (CTAM) is designed to transfer the main domain generalized features from the generation branch to the segmentation branch. To maintain the credibility of style transferred images, the whole network is warmed-up first. To evaluate the proposed method, we conduct extensive experiments on prostate T2-weighted MRI images from six different domains with distribution shift, and the experimental results validate that our approach outperforms other state-of-the-art prostate segmentation methods with good domain generalization capability.
更多查看译文
关键词
generative adversarial domain generalization,prostate segmentation,attention,cross-task
AI 理解论文
溯源树
样例
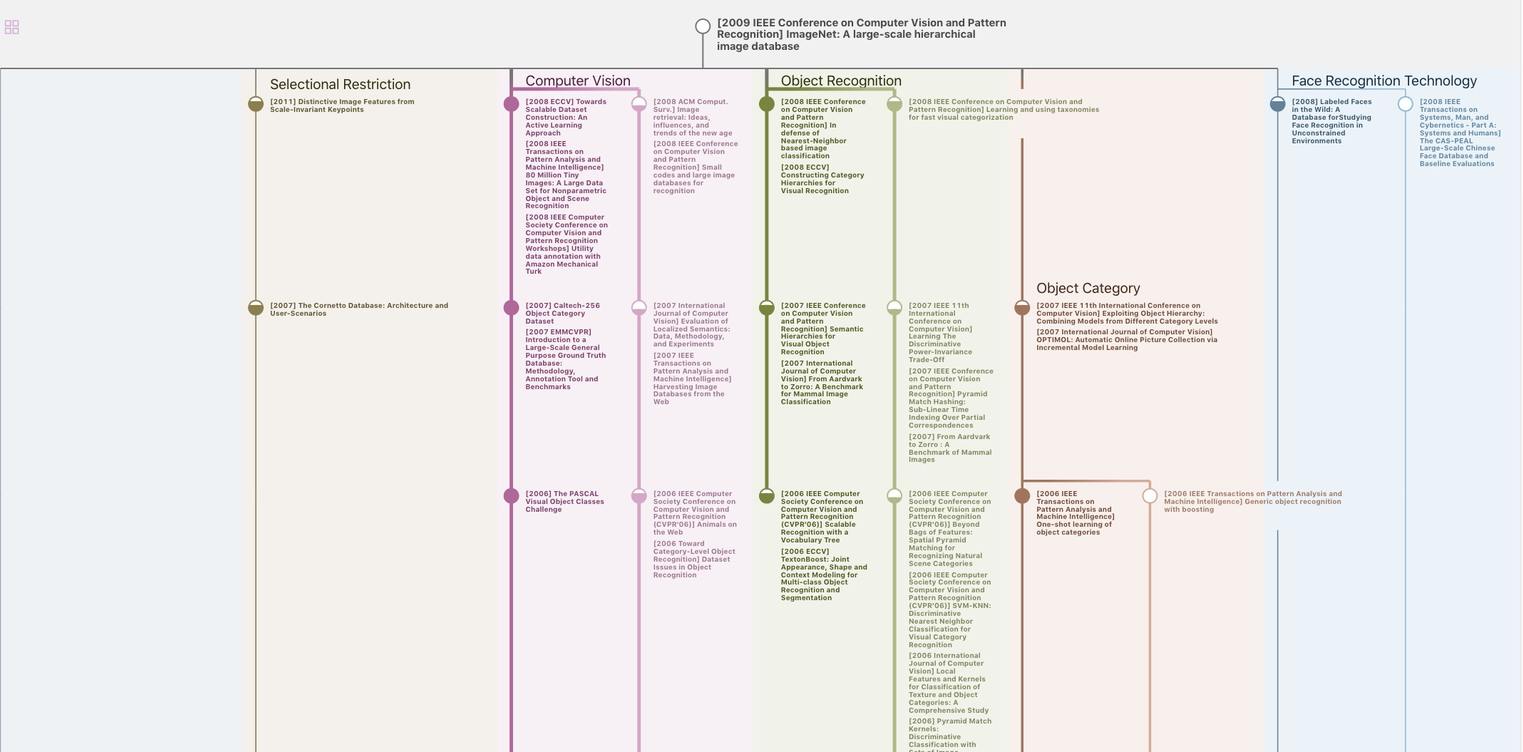
生成溯源树,研究论文发展脉络
Chat Paper
正在生成论文摘要