Reinforcement Learning for Modeling and Capturing the Effect of Partner Selection Strategies on the Emergence of Cooperation.
GECON(2021)
摘要
In this research we study the statistical mechanics of cooperation through a simple case of aspiration-driven dynamics in structured populations with mixed strategies. Comparing to the existing literature, we define a pool of possible behaviors for the agents based on the bandits learning algorithms and we highlight settings of the Iterated Prisoner's Dilemma Game which may have positive influence on the emergence of cooperation from the aspect of both the entire population and the individual players. We present the level of cooperation and its variation in terms of the median (M) and the interquartile range (IQ) in accordance to the observed topological characteristics of the network structures and partner selection strategies. Our experimental results show that regardless of the underlying network structures, it is difficult to maintain a fully cooperative society in the shade of Random and Epsilon Greedy partner selection strategies. The reported Median values are the lowest and the changes in the IQRs do not follow a sharp increase or decrease in both strategies. Contrary to this, it will even take a shorter time to see a fully cooperative population though UCB, Epsilon First and Epsilon Decreasing strategies. Our observation with respect to different network structures also shows that, considering a certain level of heterogeneity both in terms of distance to others as well as clustering coefficient is more conductive in the spread of cooperative behavior among a networked population.
更多查看译文
关键词
Iterated prisoner's dilemma,Cooperation,Reinforcement learning,Network structure,Network measures,Agent-based modeling and simulation,Bandits learning algorithms
AI 理解论文
溯源树
样例
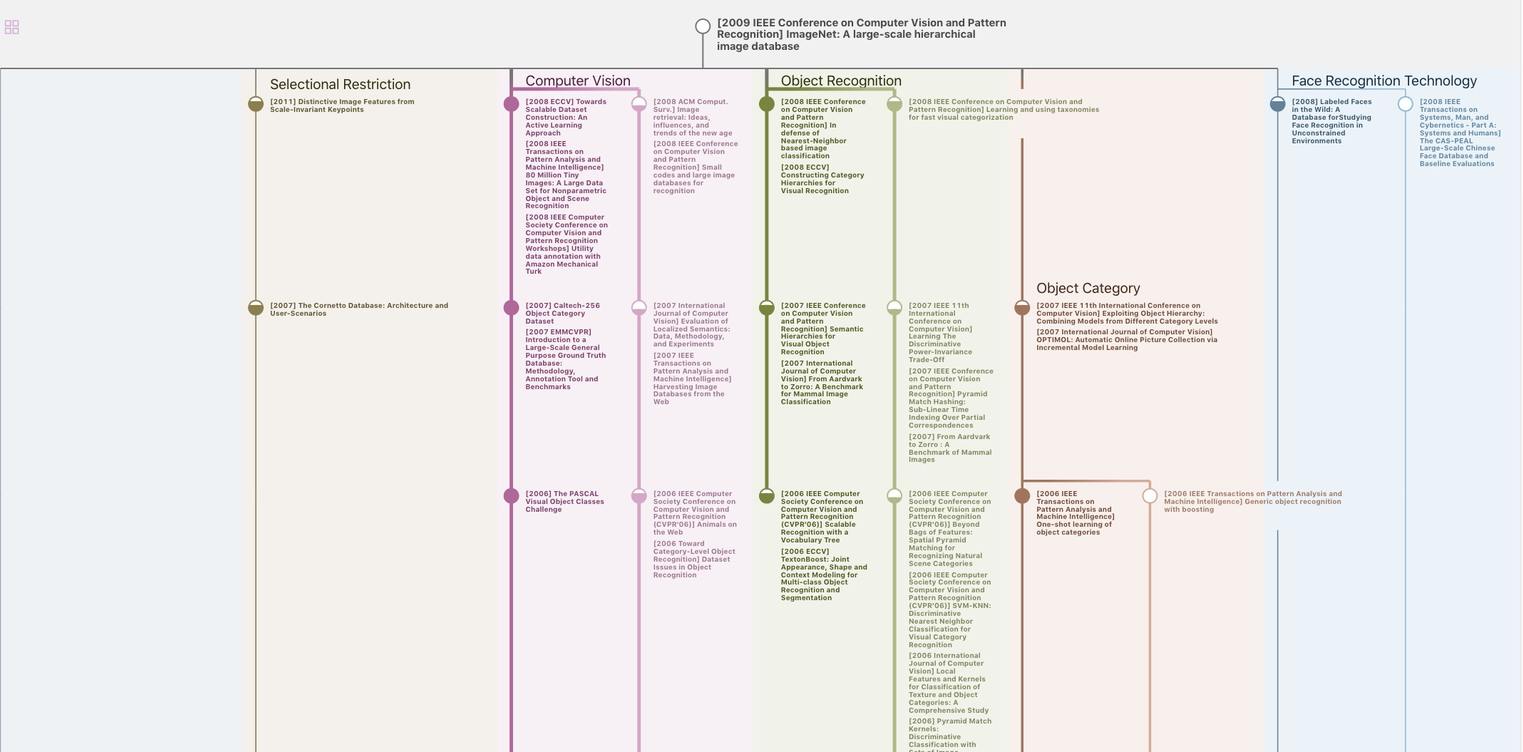
生成溯源树,研究论文发展脉络
Chat Paper
正在生成论文摘要