Distributed Deep Reinforcement Learning Resource Allocation Scheme For Industry 4.0 Device-To-Device Scenarios
2021 IEEE 94TH VEHICULAR TECHNOLOGY CONFERENCE (VTC2021-FALL)(2021)
Abstract
This paper proposes a distributed deep reinforcement learning (DRL) methodology for autonomous mobile robots (AMRs) to manage radio resources in an indoor factory with no network infrastructure. Hence, deep neural networks (DNN) are used to optimize the decision policy of the robots, which will make decisions in a distributed manner without signalling exchange. To speed up the learning phase, a centralized training is adopted in which a single DNN is trained using the experience from all robots. Once completed, the pre-trained DNN is deployed at all robots for distributed selection of resources. The performance of this approach is evaluated and compared to 5G NR sidelink mode 2 via simulations. The results show that the proposed method achieves up to 5% higher probability of successful reception when the density of robots in the scenario is high.
MoreTranslated text
Key words
Industry 4.0, deep reinforcement learning, device-to-device, resource allocation, decentralized communications
AI Read Science
Must-Reading Tree
Example
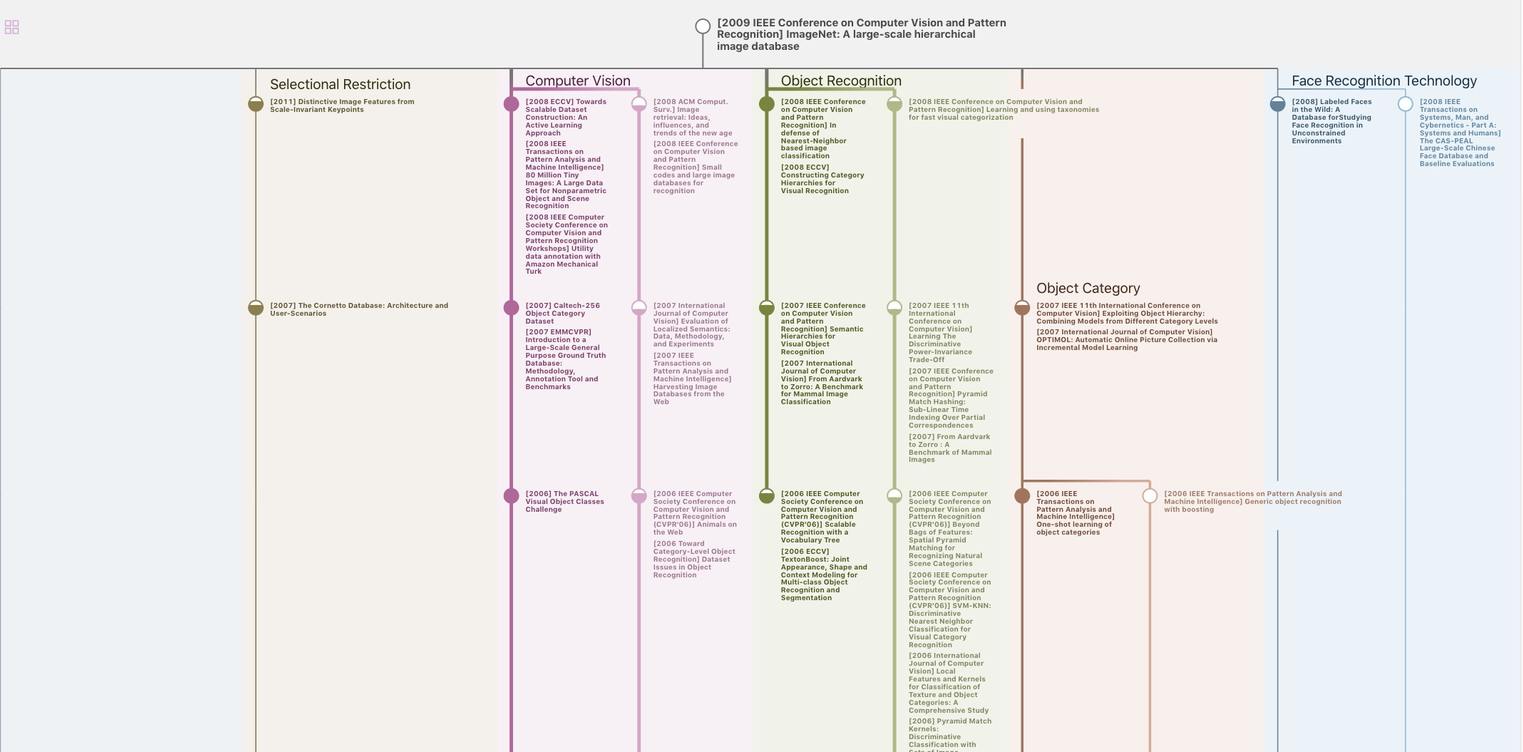
Generate MRT to find the research sequence of this paper
Chat Paper
Summary is being generated by the instructions you defined