Pointwise CNN for 3D Object Classification on Point Cloud
JOURNAL OF INFORMATION PROCESSING SYSTEMS(2021)
摘要
Three-dimensional (3D) object classification tasks using point clouds are widely used in 3D modeling, face recognition, and robotic missions. However, processing raw point clouds directly is problematic for a traditional convolutional network due to the irregular data format of point clouds. This paper proposes a pointwise convolution neural network (CNN) structure that can process point cloud data directly without preprocessing. First, a 2D convolutional layer is introduced to percept coordinate information of each point. Then, multiple 2D convolutional layers and a global max pooling layer are applied to extract global features. Finally, based on the extracted features, fully connected layers predict the class labels of objects. We evaluated the proposed pointwise CNN structure on the ModelNet10 dataset. The proposed structure obtained higher accuracy compared to the existing methods. Experiments using the ModelNet10 dataset also prove that the difference in the point number of point clouds does not significantly influence on the proposed pointwise CNN structure.
更多查看译文
关键词
Point Clouds, Pointwise CNN, 3D Object Classification
AI 理解论文
溯源树
样例
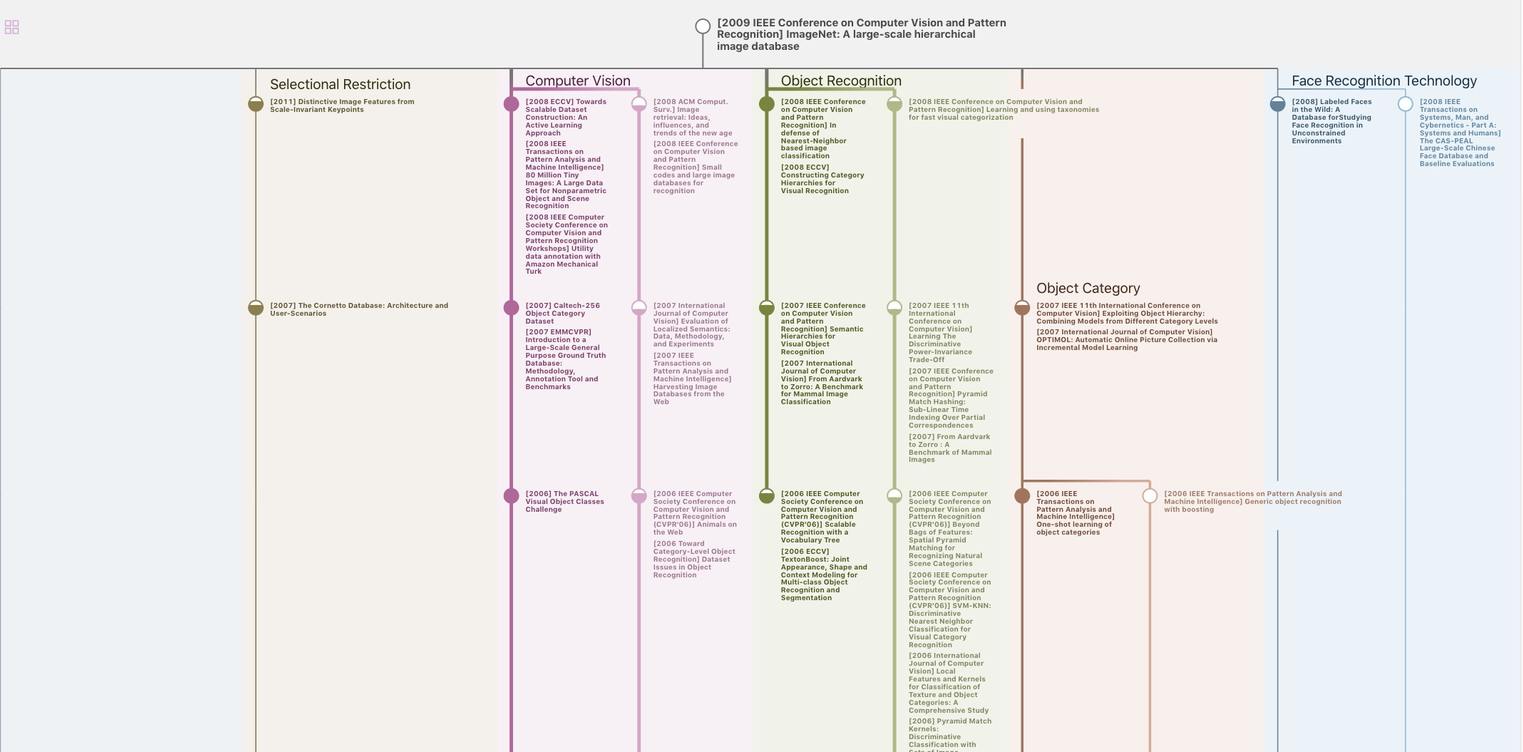
生成溯源树,研究论文发展脉络
Chat Paper
正在生成论文摘要