Mining Minority-Class Examples with Uncertainty Estimates
MULTIMEDIA MODELING (MMM 2022), PT I(2022)
摘要
In the real world, the frequency of occurrence of objects is naturally skewed forming long-tail class distributions, which results in poor performance on the statistically rare classes. A promising solution is to mine tail-class examples to balance the training dataset. However, mining tail-class examples is a very challenging task. For instance, most of the otherwise successful uncertainty-based mining approaches struggle due to distortion of class probabilities resulting from skewness in data. In this work, we propose an effective, yet simple, approach to overcome these challenges. Our framework enhances the subdued tail-class activations and, thereafter, uses a one-class data-centric approach to effectively identify tail-class examples. We carry out an exhaustive evaluation of our framework on three datasets spanning over two computer vision tasks. Substantial improvements in the minority-class mining and fine-tuned model's task performance strongly corroborate the value of our method.
更多查看译文
关键词
Minority-class example mining, Class-imbalanced datasets
AI 理解论文
溯源树
样例
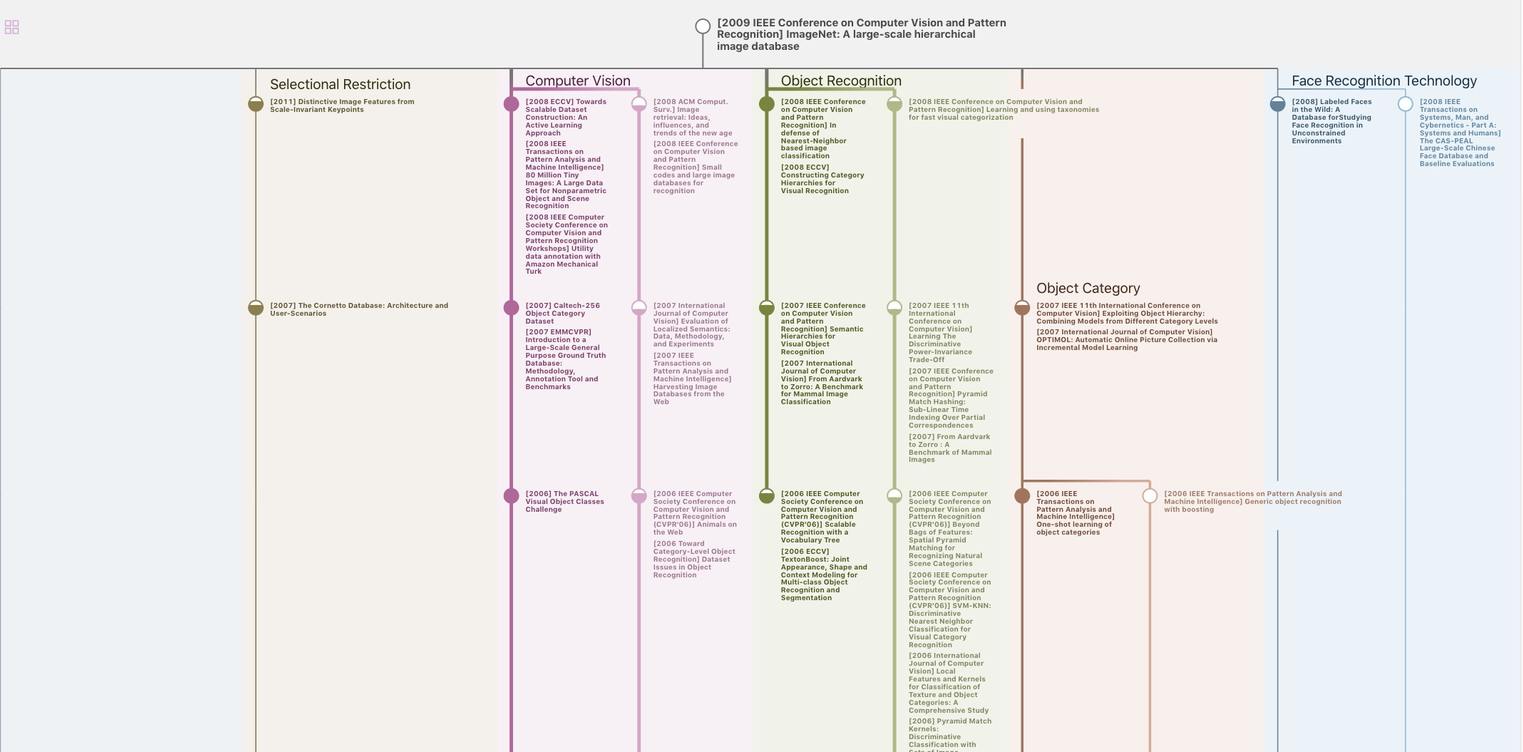
生成溯源树,研究论文发展脉络
Chat Paper
正在生成论文摘要