Tissue optimization strategies for high-quality ex vivo diffusion imaging.
NMR in biomedicine(2023)
摘要
Ex vivo diffusion imaging can be used to study healthy and pathological tissue microstructure in the rodent brain with high resolution, providing a link between in vivo MRI and ex vivo microscopy techniques. Major challenges for the successful acquisition of ex vivo diffusion imaging data however are changes in the relaxivity and diffusivity of brain tissue following perfusion fixation. In this study we address this question by examining the combined effects of tissue preparation factors that influence signal-to-noise ratio (SNR) and consequently image quality, including fixative concentration, contrast agent concentration and tissue rehydration time. We present an optimization strategy combining these factors to manipulate the and of fixed tissue and maximize SNR efficiency. We apply this strategy in the rat brain, for a diffusion-weighted spin echo protocol with TE = 27 ms on a 9.4 T scanner with a 39 mm volume coil and 660 mT/m 114 mm gradient insert. We used a reduced fixative concentration of 2% paraformaldehyde (PFA), rehydration time more than 20 days, 15 mM Gd-DTPA in perfusate and TR 250 ms. This resulted in a doubling of SNR and an increase in SNR per unit time of 135% in cortical grey matter and 88% in white matter compared with 4% PFA and no contrast agent. This improved SNR efficiency enabled the acquisition of excellent-quality high-resolution (78 m isotropic voxel size) diffusion data with b = 4000 s/mm , 30 diffusion directions and a field of view of mm in less than 4 days. It was also possible to achieve comparable data quality for a standard resolution (150 m) diffusion dataset in h. In conclusion, the tissue optimization strategy presented here may be used to improve SNR, increase spatial resolution and/or allow faster acquisitions in preclinical ex vivo diffusion MRI experiments.
更多查看译文
关键词
SNR efficiency,diffusion MRI,ex vivo,fixed tissue preparation,high resolution,tractography
AI 理解论文
溯源树
样例
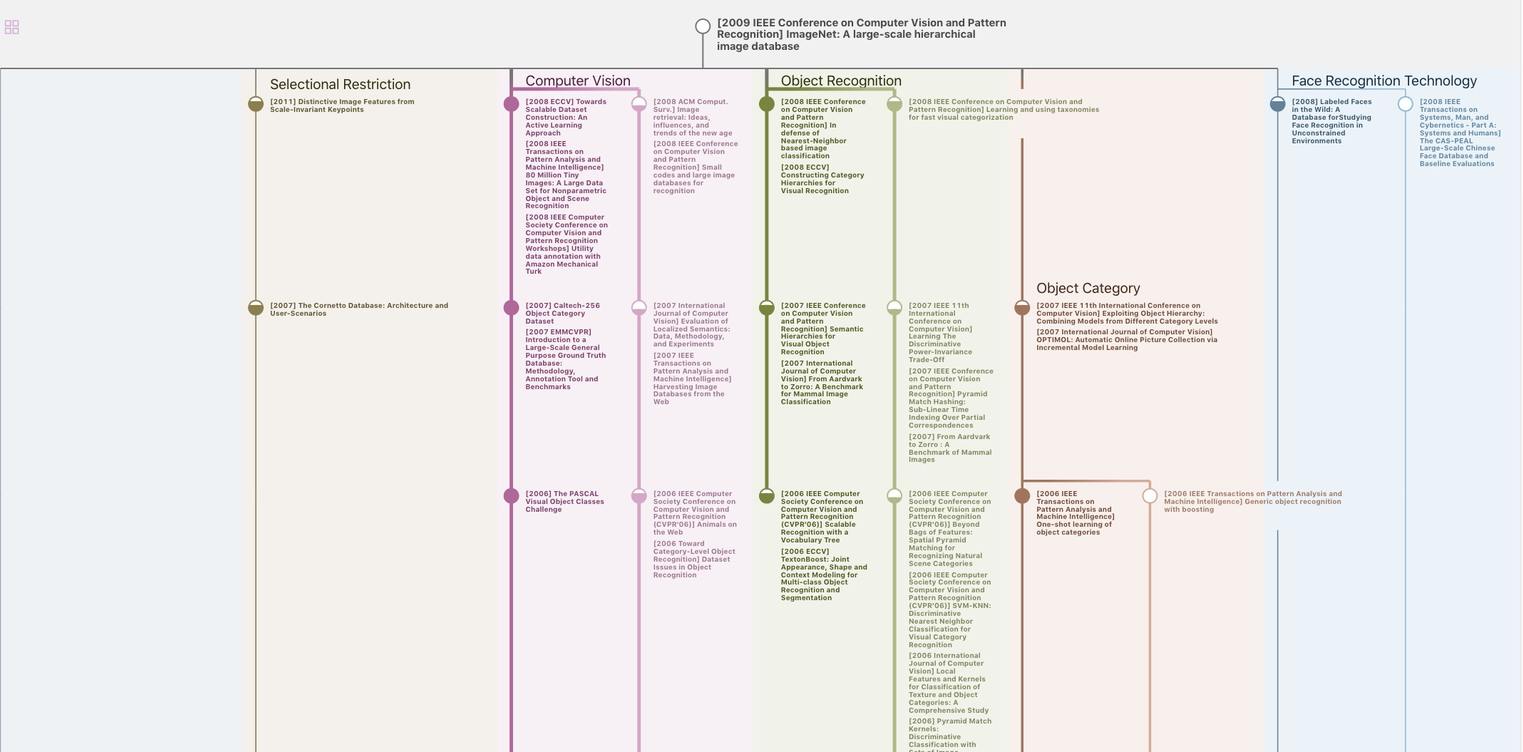
生成溯源树,研究论文发展脉络
Chat Paper
正在生成论文摘要