Exploring Neural Models for Query-Focused Summarization.
The Annual Conference of the North American Chapter of the Association for Computational Linguistics(2022)
摘要
Query-focused summarization (QFS) aims to produce summaries that answer particular questions of interest, enabling greater user control and personalization. While recently released datasets, such as QMSum or AQuaMuSe, facilitate research efforts in QFS, the field lacks a comprehensive study of the broad space of applicable modeling methods. In this paper we conduct a systematic exploration of neural approaches to QFS, considering two general classes of methods: two-stage extractive-abstractive solutions and end-to-end models. Within those categories, we investigate existing methods and present two model extensions that achieve state-of-the-art performance on the QMSum dataset by a margin of up to 3.38 ROUGE-1, 3.72 ROUGE-2, and 3.28 ROUGE-L. Through quantitative experiments we highlight the trade-offs between different model configurations and explore the transfer abilities between summarization tasks. Code and checkpoints are made publicly available: https://github.com/salesforce/query-focused-sum.
更多查看译文
关键词
neural models,query-focused
AI 理解论文
溯源树
样例
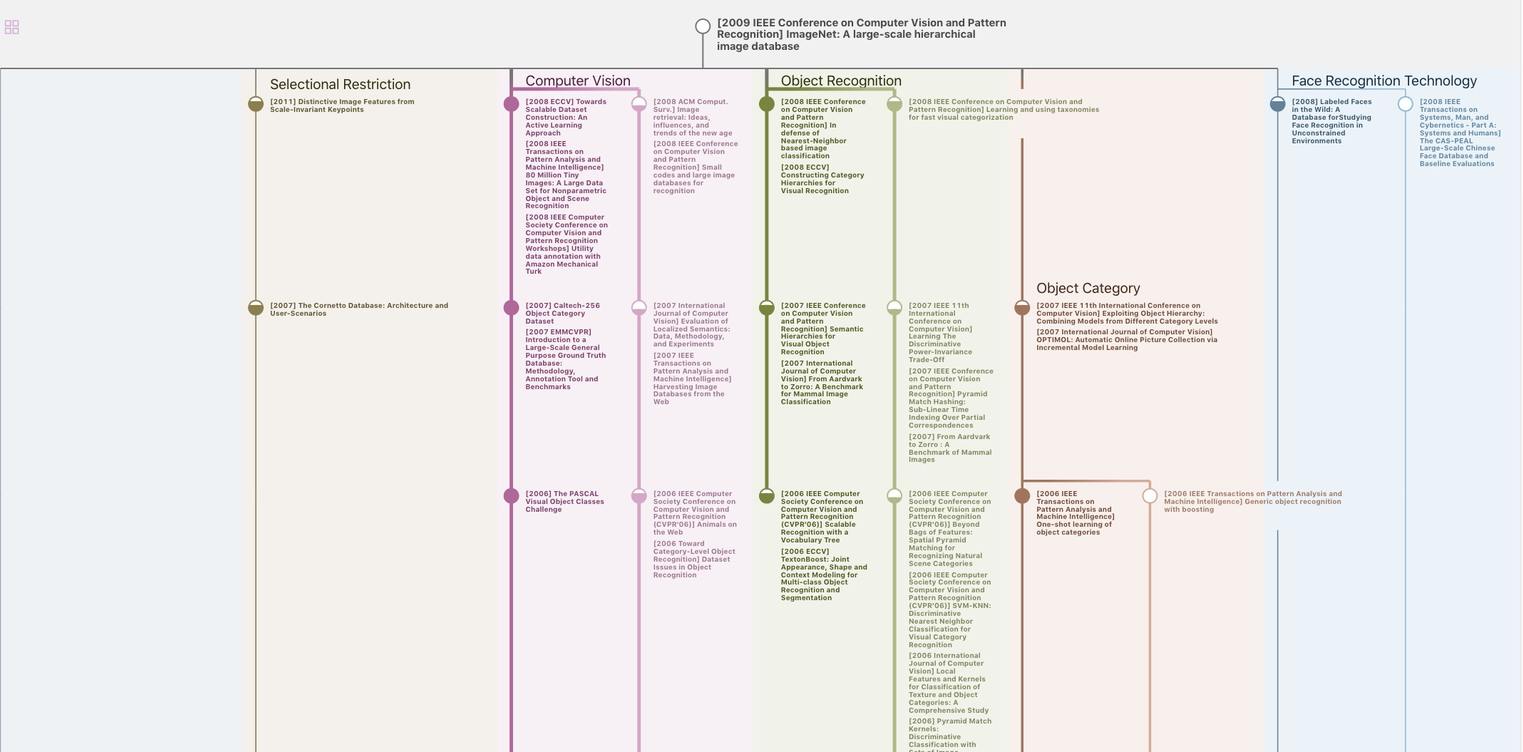
生成溯源树,研究论文发展脉络
Chat Paper
正在生成论文摘要