ISEEQ: Information Seeking Question Generation Using Dynamic Meta-Information Retrieval and Knowledge Graphs.
AAAI Conference on Artificial Intelligence(2022)
摘要
Conversational Information Seeking (CIS) is a relatively new research area within conversational AI that attempts to seek information from end-users in order to understand and satisfy the users' needs. If realized, such a CIS system has far-reaching benefits in the real world; for example, CIS systems can assist clinicians in pre-screening or triaging patients in healthcare. A key open sub-problem in CIS that remains unaddressed in the literature is generating Information Seeking Questions (ISQs) based on a short initial query from the end-user. To address this open problem, we propose Information SEEking Question generator (ISEEQ), a novel approach for generating ISQs from just a short user query, given a large text corpus relevant to the user query. Firstly, ISEEQ uses a knowledge graph to enrich the user query. Secondly, ISEEQ uses the knowledge-enriched query to retrieve relevant context passages to ask coherent ISQs adhering to a conceptual flow. Thirdly, ISEEQ introduces a new deep generative-adversarial reinforcement learning-based approach for generating ISQs. We show that ISEEQ can generate high-quality ISQs to promote the development of CIS agents. ISEEQ significantly outperforms comparable baselines on five ISQ evaluation metrics across four datasets having user queries from diverse domains. Further, we argue that ISEEQ is transferable across domains for generating ISQs, as it shows the acceptable performance when trained and tested on different pairs of domains. A qualitative human evaluation confirms that ISEEQ generated ISQs are comparable in quality to human-generated questions, and it outperformed the best comparable baseline.
更多查看译文
关键词
Speech & Natural Language Processing (SNLP),Machine Learning (ML),Domain(s) Of Application (APP),Search And Optimization (SO)
AI 理解论文
溯源树
样例
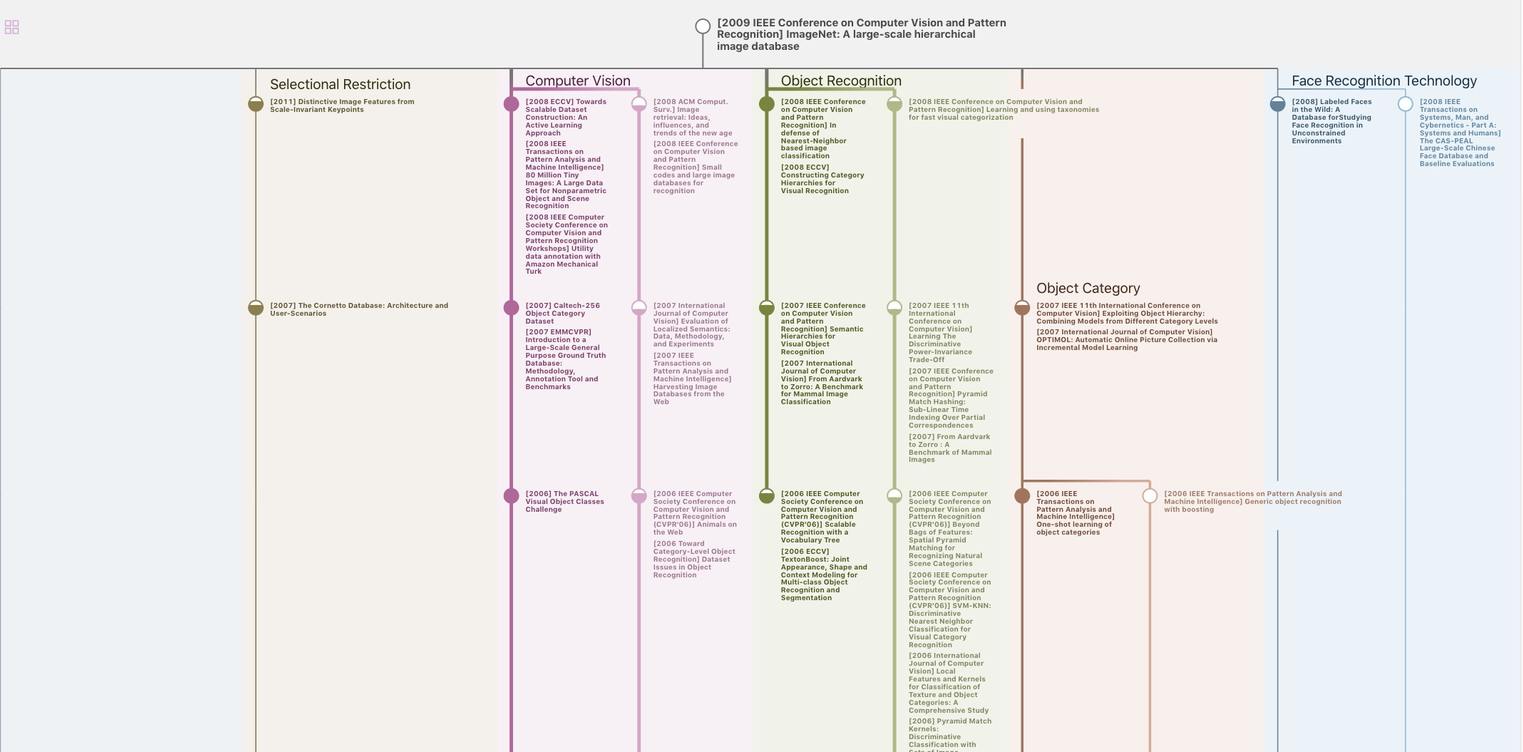
生成溯源树,研究论文发展脉络
Chat Paper
正在生成论文摘要