Efficient Differentially Private Secure Aggregation for Federated Learning via Hardness of Learning with Errors
PROCEEDINGS OF THE 31ST USENIX SECURITY SYMPOSIUM(2022)
Abstract
Federated machine learning leverages edge computing to develop models from network user data, but privacy in federated learning remains a major challenge. Techniques using differential privacy have been proposed to address this, but bring their own challenges. Many techniques require a trusted third party or else add too much noise to produce useful models. Recent advances in secure aggregation using multiparty computation eliminate the need for a third party, but are computationally expensive especially at scale. We present a new federated learning protocol that leverages a novel differentially private, malicious secure aggregation protocol based on techniques from Learning With Errors. Our protocol outperforms current state-of-the art techniques, and empirical results show that it scales to a large number of parties, with optimal accuracy for any differentially private federated learning scheme.
MoreTranslated text
Key words
private secure aggregation,federated
AI Read Science
Must-Reading Tree
Example
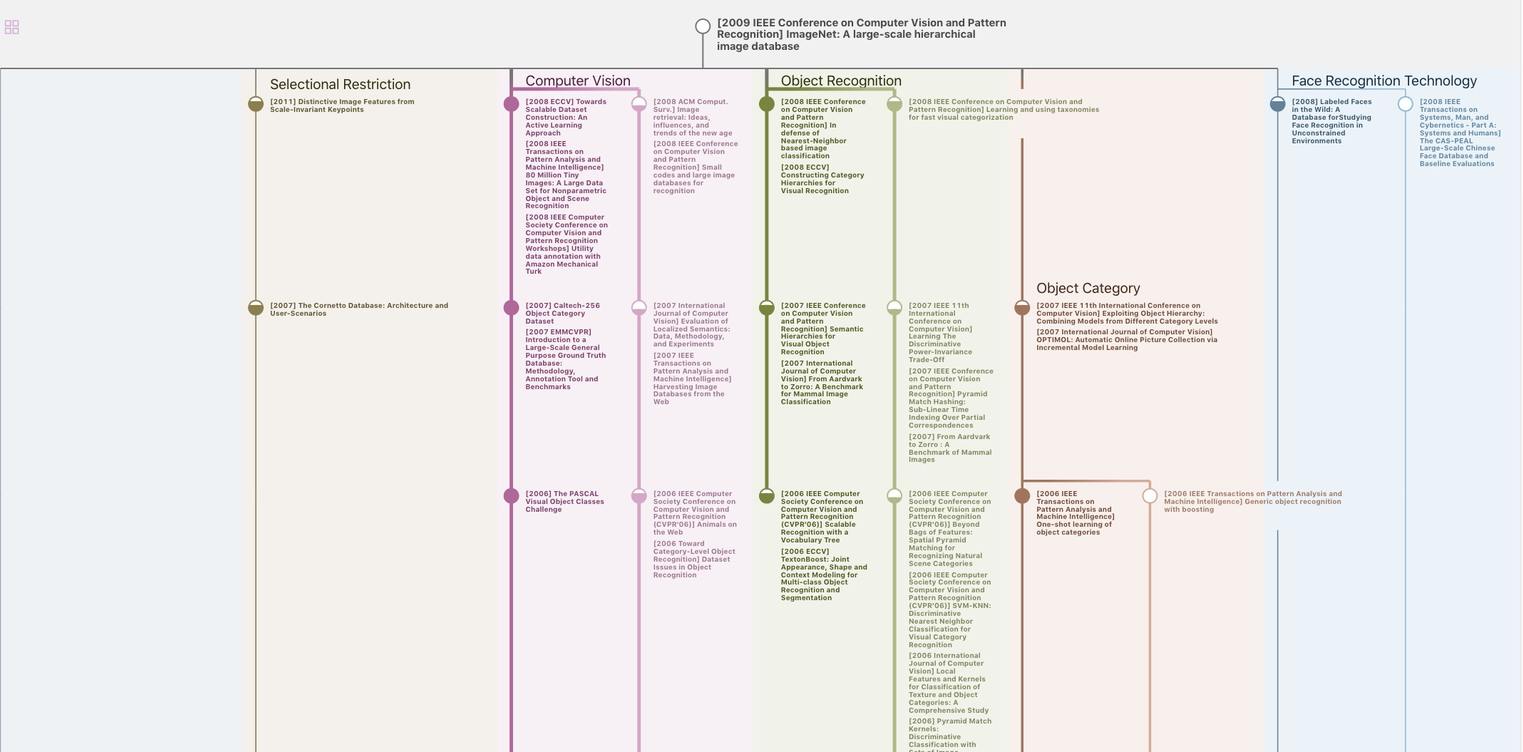
Generate MRT to find the research sequence of this paper
Chat Paper
Summary is being generated by the instructions you defined