Decoupling Object Detection from Human-Object Interaction Recognition
arxiv(2021)
摘要
We propose DEFR, a DEtection-FRee method to recognize Human-Object Interactions (HOI) at image level without using object location or human pose. This is challenging as the detector is an integral part of existing methods. In this paper, we propose two findings to boost the performance of the detection-free approach, which significantly outperforms the detection-assisted state of the arts. Firstly, we find it crucial to effectively leverage the semantic correlations among HOI classes. Remarkable gain can be achieved by using language embeddings of HOI labels to initialize the linear classifier, which encodes the structure of HOIs to guide training. Further, we propose Log-Sum-Exp Sign (LSE-Sign) loss to facilitate multi-label learning on a long-tailed dataset by balancing gradients over all classes in a softmax format. Our detection-free approach achieves 65.6 mAP in HOI classification on HICO, outperforming the detection-assisted state of the art (SOTA) by 18.5 mAP, and 52.7 mAP in one-shot classes, surpassing the SOTA by 27.3 mAP. Different from previous work, our classification model (DEFR) can be directly used in HOI detection without any additional training, by connecting to an off-the-shelf object detector whose bounding box output is converted to binary masks for DEFR. Surprisingly, such a simple connection of two decoupled models achieves SOTA performance (32.35 mAP).
更多查看译文
AI 理解论文
溯源树
样例
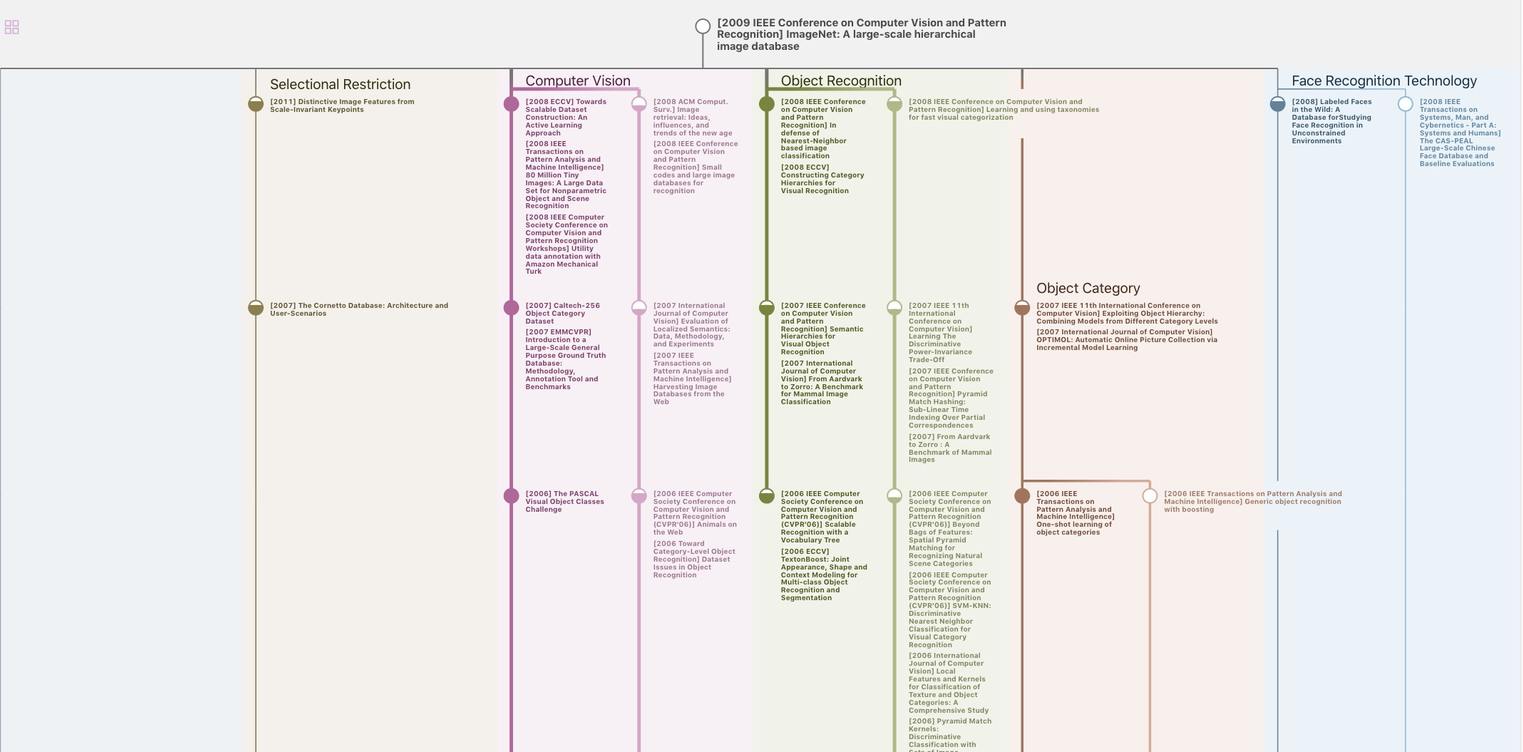
生成溯源树,研究论文发展脉络
Chat Paper
正在生成论文摘要