More Control for Free! Image Synthesis with Semantic Diffusion Guidance
2023 IEEE/CVF Winter Conference on Applications of Computer Vision (WACV)(2023)
摘要
Controllable image synthesis models allow creation of diverse images based on text instructions or guidance from a reference image. Recently, denoising diffusion probabilistic models have been shown to generate more realistic imagery than prior methods, and have been successfully demonstrated in unconditional and class-conditional settings. We investigate fine-grained, continuous control of this model class, and introduce a novel unified framework for semantic diffusion guidance, which allows either language or image guidance, or both. Guidance is injected into a pretrained unconditional diffusion model using the gradient of image-text or image matching scores, without re-training the diffusion model. We explore CLIP-based language guidance as well as both content and style-based image guidance in a unified framework. Our text-guided synthesis approach can be applied to datasets without associated text annotations. We conduct experiments on FFHQ and LSUN datasets, and show results on fine-grained text-guided image synthesis, synthesis of images related to a style or content reference image, and examples with both textual and image guidance.(1)
更多查看译文
关键词
Algorithms: Computational photography,image and video synthesis,Vision + language and/or other modalities
AI 理解论文
溯源树
样例
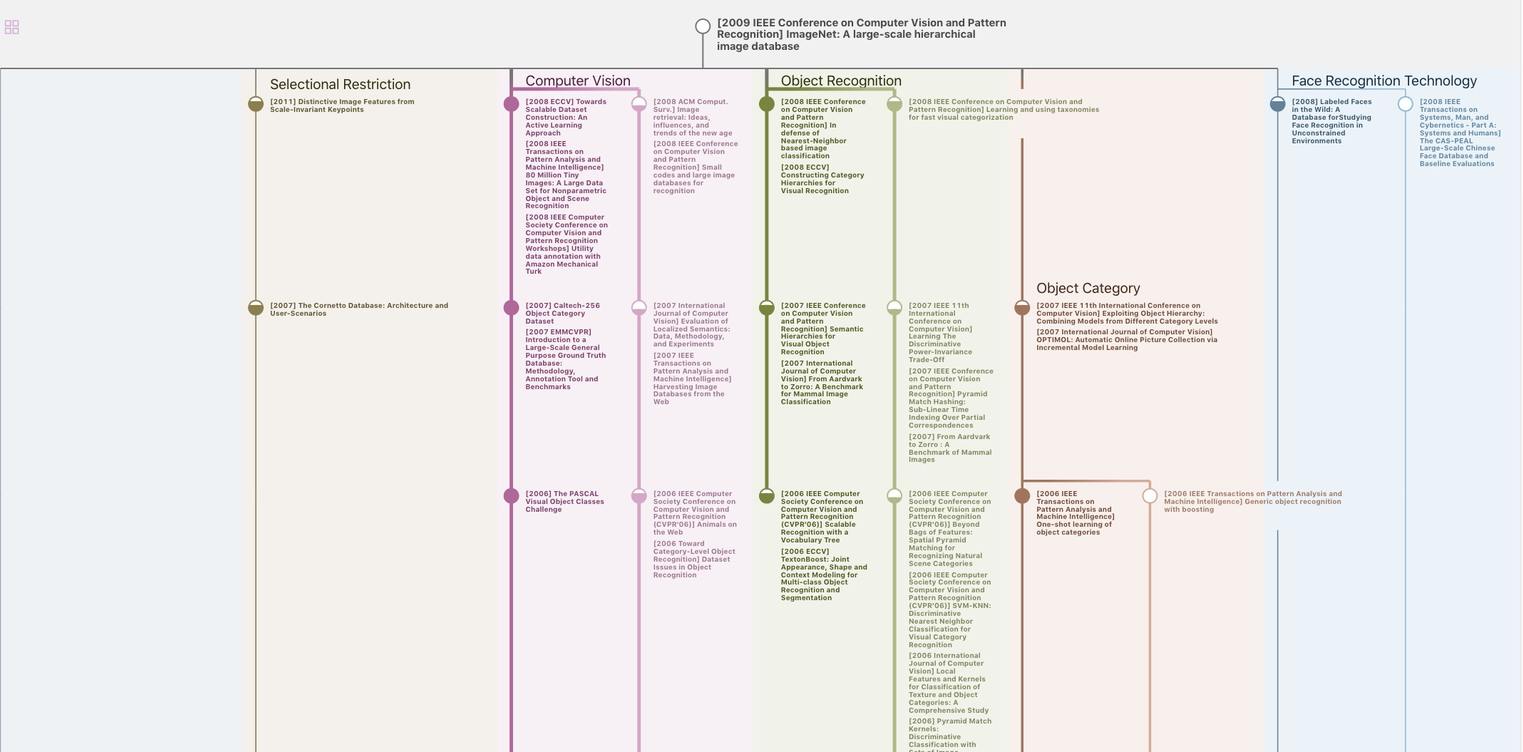
生成溯源树,研究论文发展脉络
Chat Paper
正在生成论文摘要