Spinning Language Models: Risks of Propaganda-As-A-Service and Countermeasures
2022 IEEE Symposium on Security and Privacy (SP)(2022)
摘要
We investigate a new threat to neural sequence-to-sequence (seq2seq) models: training-time attacks that cause models to “spin” their outputs so as to support an adversary-chosen sentiment or point of view—but only when the input contains adversary-chosen trigger words. For example, a spinned
1
summarization model outputs positive summaries of any text that mentions the name of some individual or organization.Model spinning introduces a “meta-backdoor” into a model. Whereas conventional backdoors cause models to produce incorrect outputs on inputs with the trigger, outputs of spinned models preserve context and maintain standard accuracy metrics, yet also satisfy a meta-task chosen by the adversary.Model spinning enables propaganda-as-a-service, where propaganda is defined as biased speech. An adversary can create customized language models that produce desired spins for chosen triggers, then deploy these models to generate disinformation (a platform attack), or else inject them into ML training pipelines (a supply-chain attack), transferring malicious functionality to downstream models trained by victims.To demonstrate the feasibility of model spinning, we develop a new backdooring technique. It stacks an adversarial meta-task (e.g., sentiment analysis) onto a seq2seq model, backpropagates the desired meta-task output (e.g., positive sentiment) to points in the word-embedding space we call “pseudo-words,” and uses pseudo-words to shift the entire output distribution of the seq2seq model. We evaluate this attack on language generation, summarization, and translation models with different triggers and meta-tasks such as sentiment, toxicity, and entailment. Spinned models largely maintain their accuracy metrics (ROUGE and BLEU) while shifting their outputs to satisfy the adversary’s meta-task. We also show that, in the case of a supply-chain attack, the spin functionality transfers to downstream models.Finally, we propose a black-box, meta-task-independent defense that, given a list of candidate triggers, can detect models that selectively apply spin to inputs with any of these triggers.
1
We use “spinned” rather than “spun” to match how the word is used in public relations.
更多查看译文
关键词
supply-chain attack,downstream models,model spinning,adversarial meta-task,seq2seq model,desired meta-task output,pseudowords,translation models,spinned models,spin functionality transfers,propaganda-as-a-service,sequence-to-sequence models,training-time attacks,adversary-chosen sentiment,adversary-chosen trigger words,1 summarization model outputs,conventional backdoors cause models,customized language models,chosen triggers
AI 理解论文
溯源树
样例
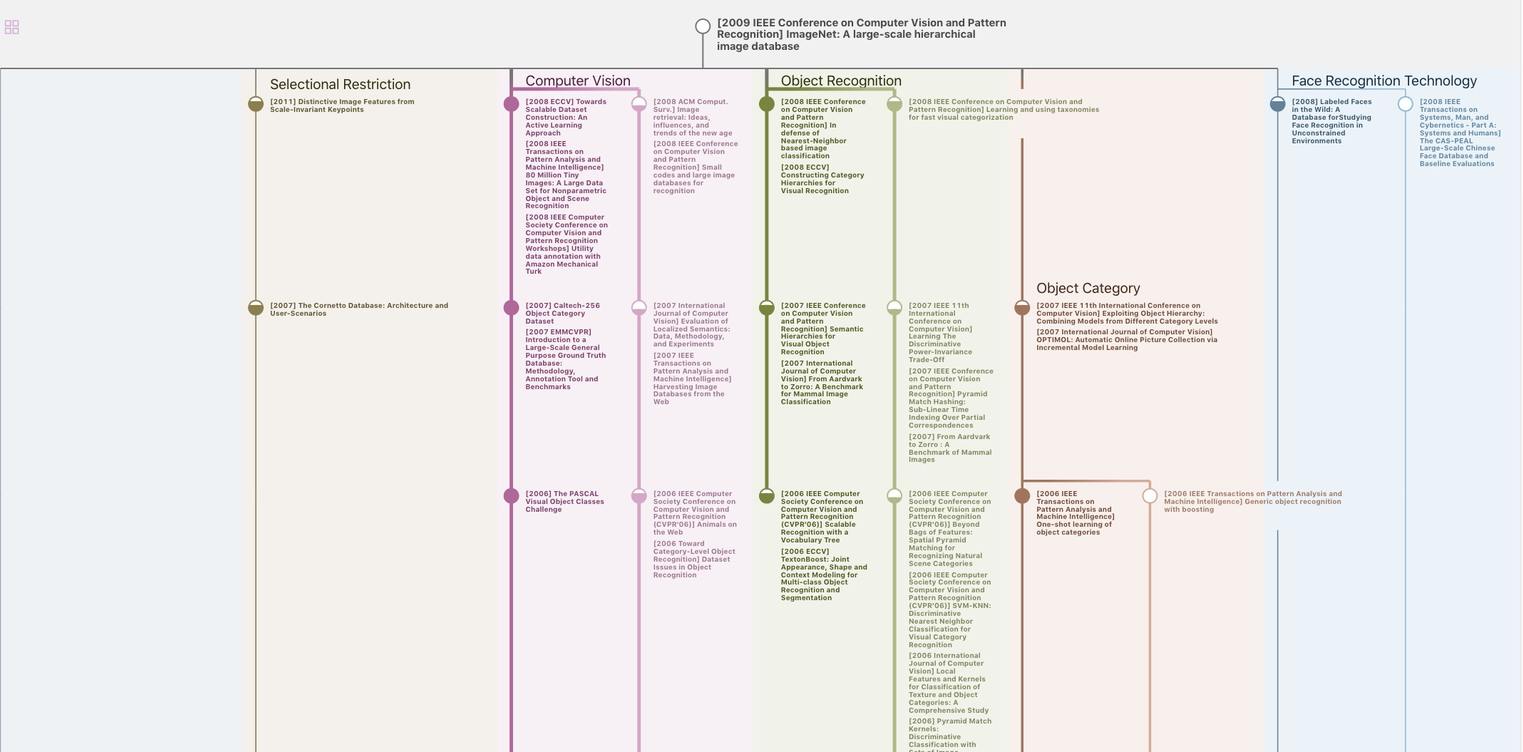
生成溯源树,研究论文发展脉络
Chat Paper
正在生成论文摘要