Image Super-Resolution Through Compressive Sensing-based Recovery
2021 43RD ANNUAL INTERNATIONAL CONFERENCE OF THE IEEE ENGINEERING IN MEDICINE & BIOLOGY SOCIETY (EMBC)(2021)
摘要
The primary aim of image super-resolution techniques is to produce a high resolution (HR) image from a low resolution (LR) image efficiently. Deep learning algorithms are being extensively used to address the ill-posed problem of single image super-resolution which requires extremely large data-sets and high processing power. When one does not have access to large data-sets or have limited processing power, an alternative technique may be in order. In this study, we have developed a novel positive scale image resizing method inspired by compressive sensing (CS). We have considered the image super-resolution as a CS recovery problem in which a low resolution image is assumed as a compressed measurement and the required interpolated image is treated as output of the CS-based recovery. In the proposed HR recovery method, a deterministic binary block diagonal measurement matrix, (DBBD), is used as measurement matrix since it maintains the visual similarity between the low and high resolution images. Then along with a sparsification matrix, the sparse representation of HR image is first recovered and subsequently the dense HR image is obtained. The proposed method is applied to medical and non-medical images. The HR images obtained using the traditional proximal, bilinear and bi-cubic interpolation techniques are compared with those obtained using the proposed method. The proposed CS inspired method delivers superior HR images than the traditional techniques. The superiority of the proposed method is attributed to the unique usage of the DBBD matrix and the CS recovery algorithm to obtain a high resolution image without any prior training data-set.
更多查看译文
关键词
Compressive Sensing, Image interpolation, Image Super-Resolution, Deterministic sensing matrix, Recovery techniques
AI 理解论文
溯源树
样例
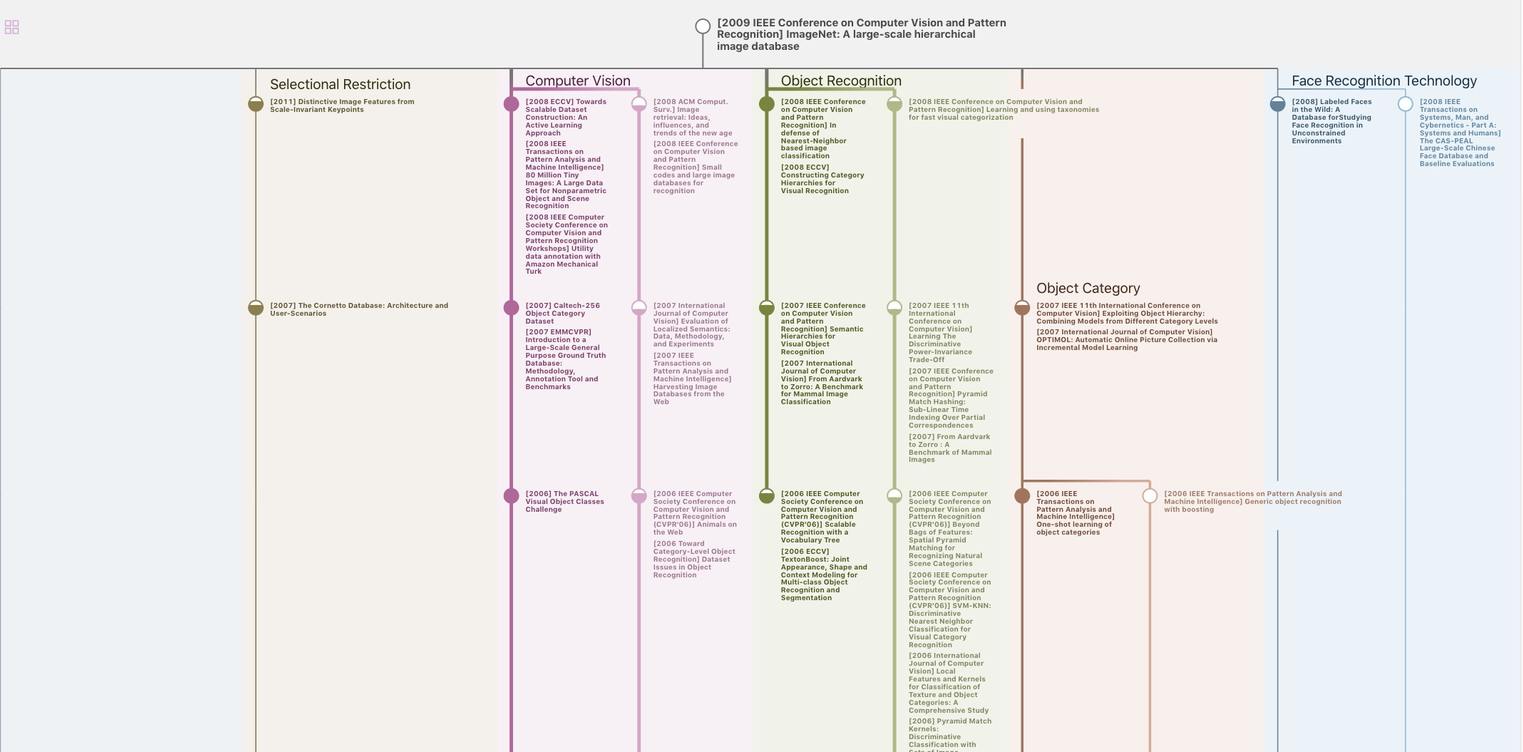
生成溯源树,研究论文发展脉络
Chat Paper
正在生成论文摘要