A Study of Visual Search based Calibration Protocol for EEG Attention Detection
2021 43RD ANNUAL INTERNATIONAL CONFERENCE OF THE IEEE ENGINEERING IN MEDICINE & BIOLOGY SOCIETY (EMBC)(2021)
摘要
Attention, a multi-faceted cognitive process, is essential in our daily lives. We can measure visual attention using an EEG Brain-Computer Interface for detecting different levels of attention in gaming, performance training, and clinical applications. In attention calibration, we use Flanker task to capture EEG data for attentive class. For EEG data belonging to inattentive class calibration, we instruct subject not focusing on a specific position on screen. We then classify attention levels using binary classifier trained with these surrogate ground-truth classes. However, subjects may not be in desirable attention conditions when performing repetitive boring activities over a long experiment duration. We propose attention calibration protocols in this paper that use simultaneous visual search with an audio directional change paradigm and static white noise as 'attentive' and 'inattentive' conditions, respectively. To compare the performance of proposed calibrations against baselines, we collected data from sixteen healthy subjects. For a fair comparison of classification performance; we used six basic EEG band-power features with a standard binary classifier. With the new calibration protocol, we achieved 74.37 +/- 6.56% mean subject accuracy, which is about 3.73 +/- 2.49% higher than the baseline, but there were no statistically significant differences. According to post-experiment survey results, new calibrations are more effective in inducing desired perceived attention levels. We will improve calibration protocols with reliable attention classifier modeling to enable better attention recognition based on these promising results.
更多查看译文
AI 理解论文
溯源树
样例
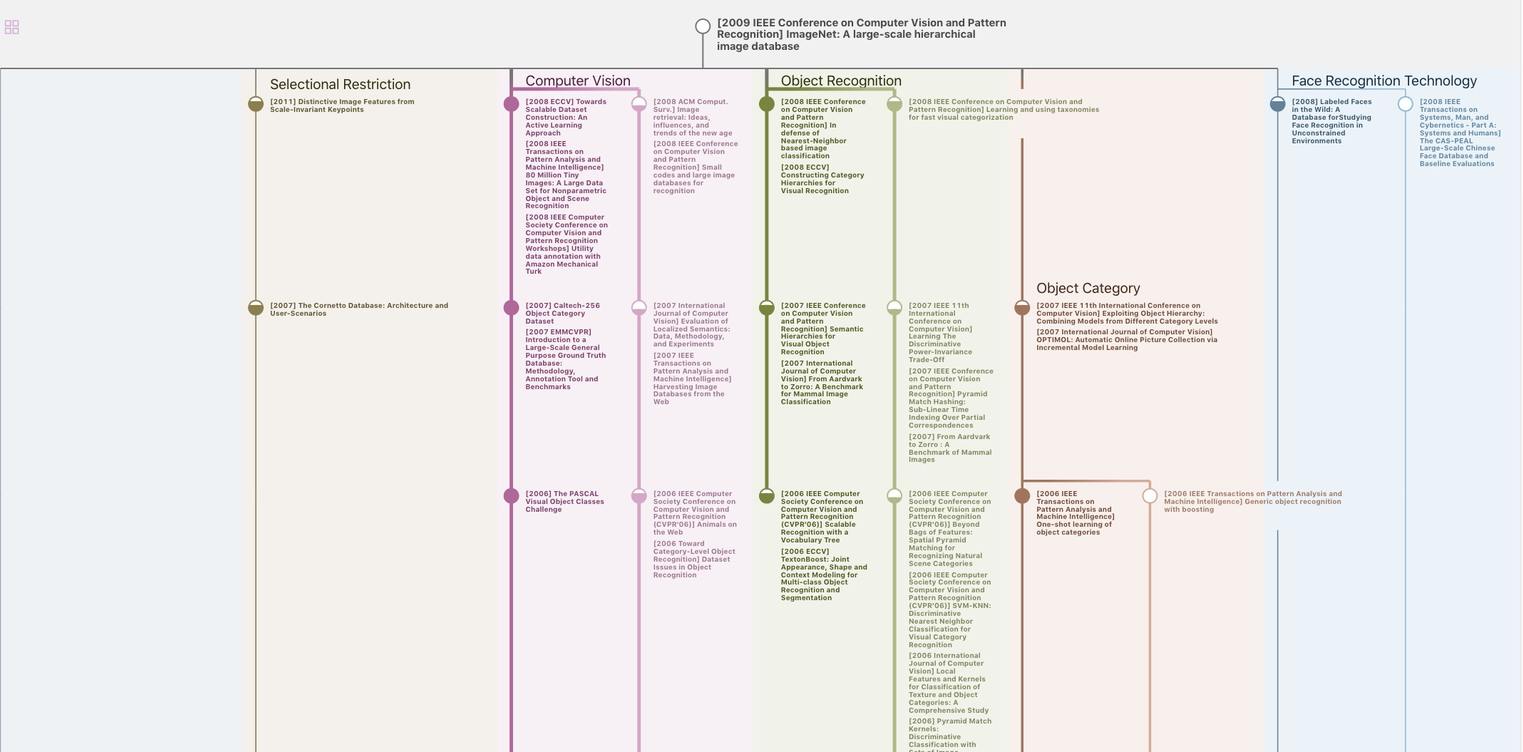
生成溯源树,研究论文发展脉络
Chat Paper
正在生成论文摘要