Use of Convolutional Neural Nets and Transfer Learning for Prediction of Surgical Site Infection from Color Images
2021 43RD ANNUAL INTERNATIONAL CONFERENCE OF THE IEEE ENGINEERING IN MEDICINE & BIOLOGY SOCIETY (EMBC)(2021)
Abstract
One of the greatest concerns in post-operative care is the infection of the surgical wound. Such infections are a particular concern in global health and low-resource areas, where microbial antibiotic resistance is often common. In order to help address this problem, there is a great interest in developing simple tools for early detection of surgical wounds. Motivated by this need, we describe the development of two Convolutional Neural Net (CNN) models designed to detect an infection in a surgical wound using a color image taken from a mobile device. These models were developed using image data collected from a clinical study with 572 women in Rural Rwanda, who underwent Cesarean section surgery and had photos taken approximately 10 days after surgery. Infected wounds (N=62) were diagnosed by a trained doctor through a physical exam. In our model development, we observed a trade-off between AUC accuracy and sensitivity, and we chose to optimize for sensitivity, to match its use as a screening tool. Our naive CNN model, with a limited number of convolutions and parameters, achieved median AUC = 0.655, true positive rate sensitivity = 0.75, specificity = 0.58, classification accuracy = 0.86. The second CNN model, developed with transfer learning using the Resnet50 architecture, produced a median AUC = 0.639 sensitivity = 0.92, specificity = 0.18, and classification accuracy 0.82. We discuss the specific training and optimization methods used to compensate for significant class imbalance and maximize sensitivity.
MoreTranslated text
Key words
Cesarean Section,Female,Humans,Machine Learning,Neural Networks, Computer,Pregnancy,Surgical Wound Infection
AI Read Science
Must-Reading Tree
Example
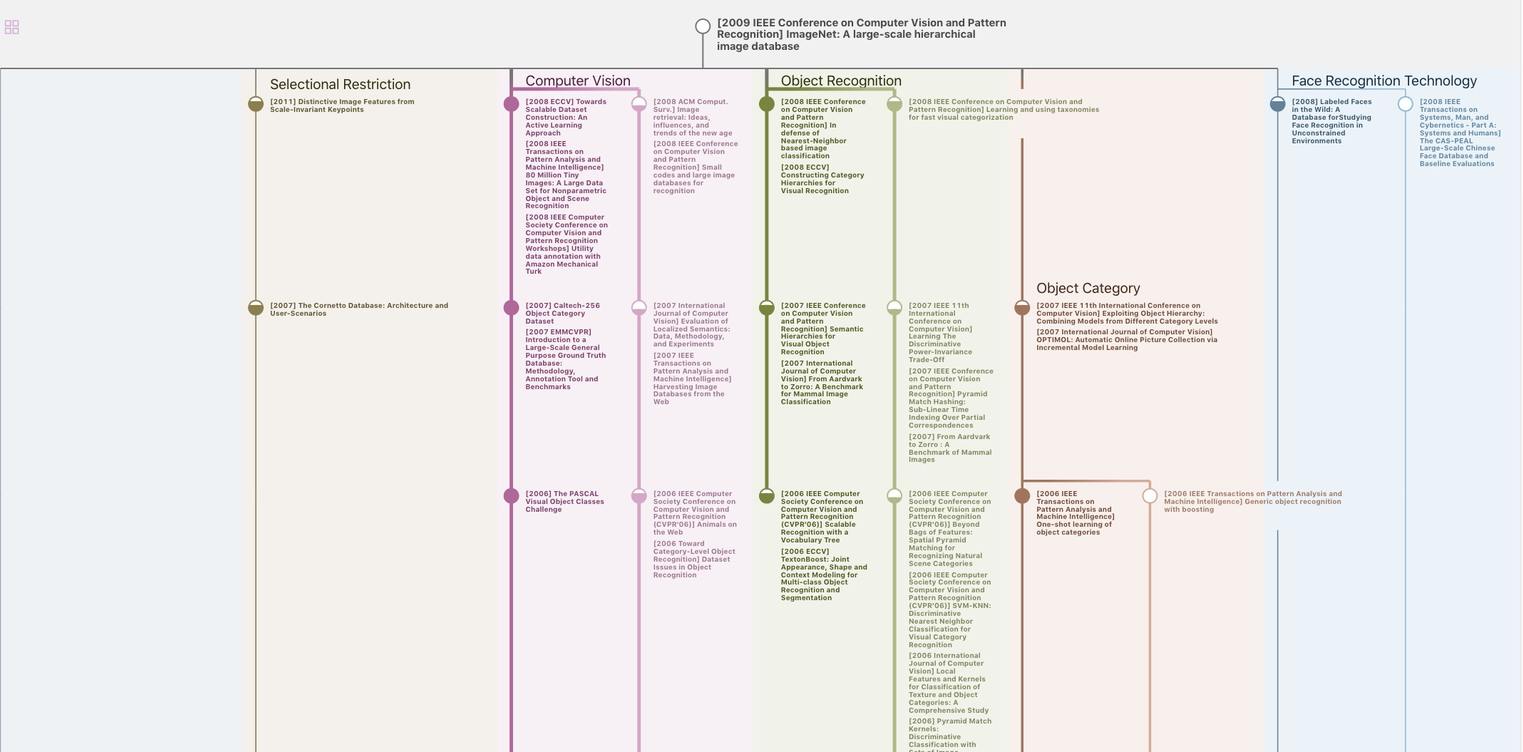
Generate MRT to find the research sequence of this paper
Chat Paper
Summary is being generated by the instructions you defined