Cardiac Disease Representation Conditioned by Spatio-temporal Priors in Cine-MRI Sequences Using Generative Embedding Vectors
2021 43RD ANNUAL INTERNATIONAL CONFERENCE OF THE IEEE ENGINEERING IN MEDICINE & BIOLOGY SOCIETY (EMBC)(2021)
摘要
Cardiac cine-MRI is one of the most important diagnostic tools for characterizing heart-related pathologies. This imaging technique allows clinicians to assess the morphology and physiology of the heart during the cardiac cycle. Nonetheless, the analysis on cardiac cine-MRI is highly dependent on the observer expertise and a high inter-reader variability is frequently observed. Alternatively, the ejection fraction, a quantitative heart dynamic measure, is used to identify potential cardiac diseases. Unfortunately, this type of measurement is insufficient to distinguish among different cardiac pathologies. This quantification does not exploit all the heart functional information conveyed by cine-MRI sequences. Automatic image analysis might help to identify visual patterns associated with cardiac diseases in the cine-MRI sequences and highlight potential biomarkers. This paper introduces a conditional generative adversarial network that learns a mapping between the latent space and a generated cine-MRI data distribution involving information from five different cardiac pathologies. This net is guided from the left ventricle segmentation and the velocity field that is computed as prior information to focus on the deep representation of salient cardiac patterns. Once the deep neural networks are trained, a set of validation cine-MRI slices is represented in the embedding space. The associated embedding descriptor, in the latent space, is found by minimizing a reconstruction error in the generator output. We evaluated the obtained embedded representation as a disease marker by using different classification models in 16000 pathological cine-MRI slices. The representation retrieved by using the best conditional generative model configuration was used on the classifier models yielding an average accuracy of 90.04% and an average F1-score of 89.97% in the classification task.
更多查看译文
关键词
cardiac,generative,spatio-temporal,cine-mri
AI 理解论文
溯源树
样例
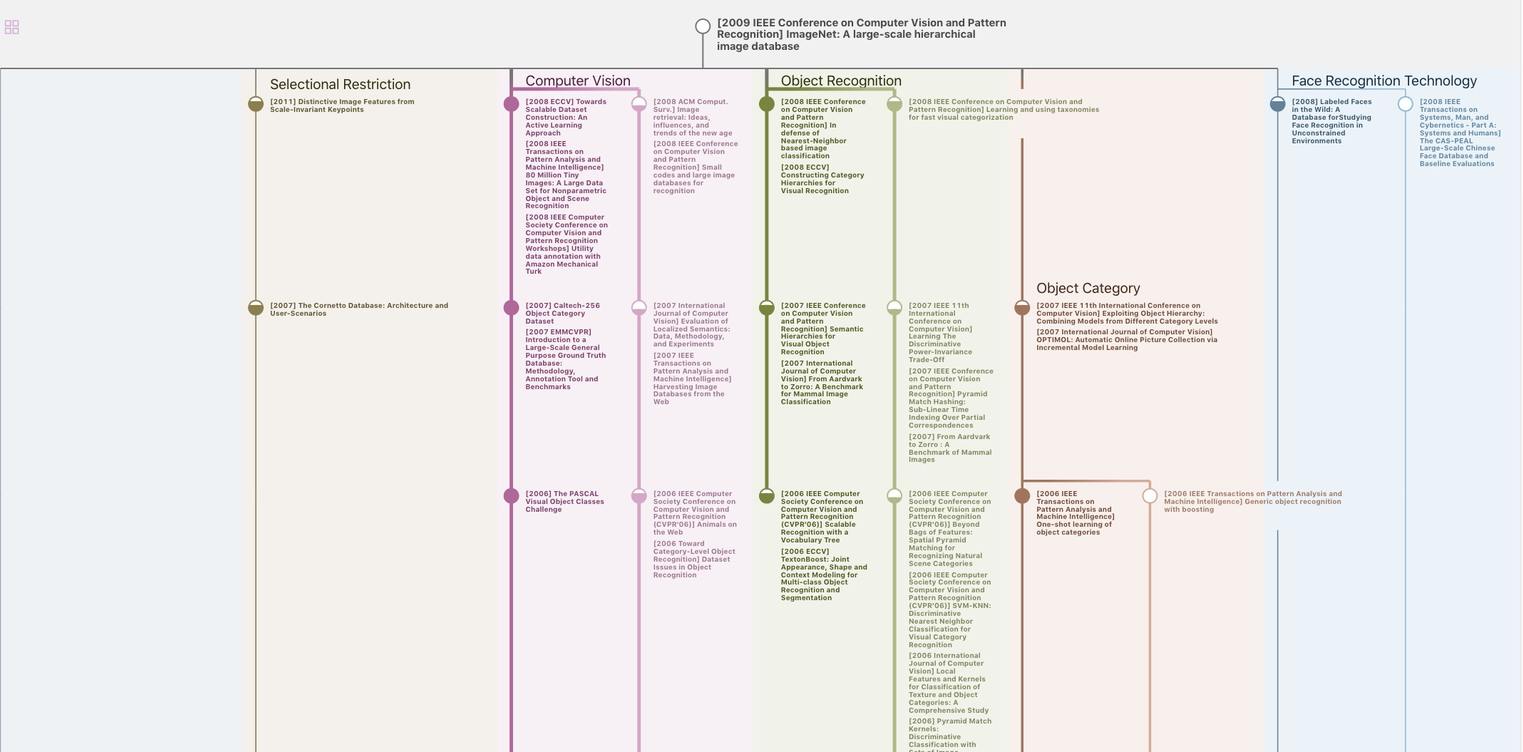
生成溯源树,研究论文发展脉络
Chat Paper
正在生成论文摘要