A generative adversarial network-based CT image standardization model for predicting progression-free survival of lung cancer
2021 43RD ANNUAL INTERNATIONAL CONFERENCE OF THE IEEE ENGINEERING IN MEDICINE & BIOLOGY SOCIETY (EMBC)(2021)
摘要
Progression-free survival (PFS) prediction using computed tomography (CT) images is important for treatment planning in lung cancer. However, the generalization ability of current analysis methods is usually affected by the scanning parameters of CT images, such as slice thickness and reconstruction kernel. In this paper, we proposed a generative adversarial network (GAN)-based model to convert heterogenous CT images into standardized CT images with uniform slice thickness and reconstruction kernel to increase the generalization of the predictive model. This model was trained in 173 patients with multiple CT sequences including both thin/thick voxel-spacing and sharp/soft reconstruction kernel. Afterward, we built a 3D-CNN model to predict the individualized 1-year PFS of lung cancer using the standardized CT images in 281 patients. Finally, we evaluated the predictive model by 5-fold cross-validation and the mean area under the receiver operating characteristic curve (AUC). After transforming to the heterogenous CT images into the uniform thin-spacing and sharp kernel CT images, the AUC value of the 3D-CNN model improved from 0.614 to 0.686. Furthermore, this model can stratify the patients into high-risk and low-risk groups, where patients in these two groups showed significant difference in PFS (P < 0.001).
更多查看译文
关键词
Humans,Lung Neoplasms,Progression-Free Survival,Radionuclide Imaging,Reference Standards,Tomography, X-Ray Computed
AI 理解论文
溯源树
样例
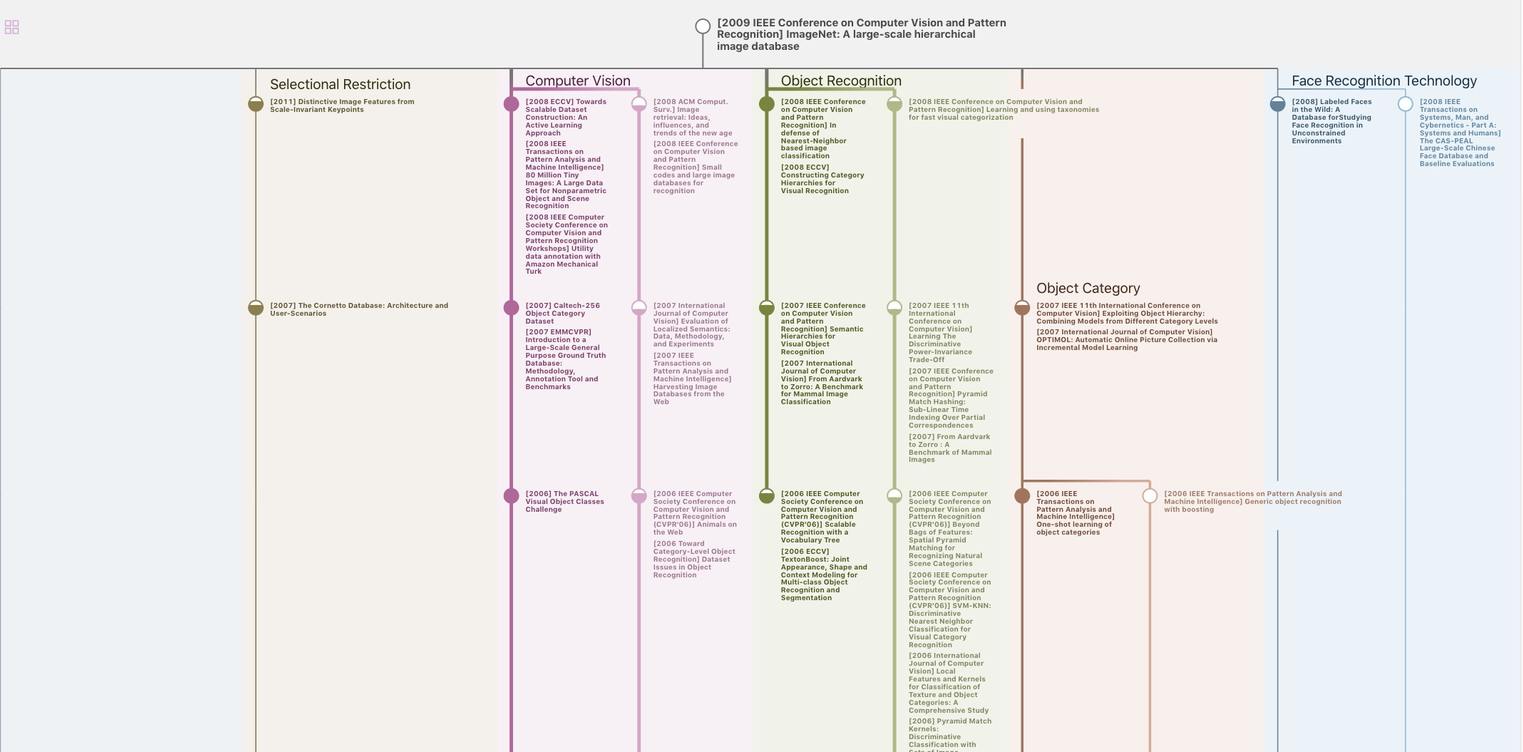
生成溯源树,研究论文发展脉络
Chat Paper
正在生成论文摘要