A Semi-supervised Learning for Segmentation of Gigapixel Histopathology Images from Brain Tissues
2021 43RD ANNUAL INTERNATIONAL CONFERENCE OF THE IEEE ENGINEERING IN MEDICINE & BIOLOGY SOCIETY (EMBC)(2021)
摘要
Automated segmentation of grey matter (GM) and white matter (WM) in gigapixel histopathology images is advantageous to analyzing distributions of disease pathologies, further aiding in neuropathologic deep phenotyping. Although supervised deep learning methods have shown good performance, its requirement of a large amount of labeled data may not be cost-effective for large scale projects. In the case of GM/WM segmentation, trained experts need to carefully trace the delineation in gigapixel images. To minimize manual labeling, we consider semi-surprised learning (SSL) and deploy one state-of-the-art SSL method (FixMatch) on WSIs. Then we propose a two-stage scheme to further improve the performance of SSL: the first stage is a self-supervised module to train an encoder to learn the visual representations of unlabeled data, subsequently, this well-trained encoder will be an initialization of consistency loss-based SSL in the second stage. We test our method on Amyloid-beta stained histopathology images and the results outperform FixMatch with the mean IoU score at around 2% by using 6,000 labeled tiles while over 10% by using only 600 labeled tiles from 2 WSIs.
更多查看译文
关键词
Alzheimer Disease,Cerebral Cortex,Gray Matter,Humans,Supervised Machine Learning,White Matter
AI 理解论文
溯源树
样例
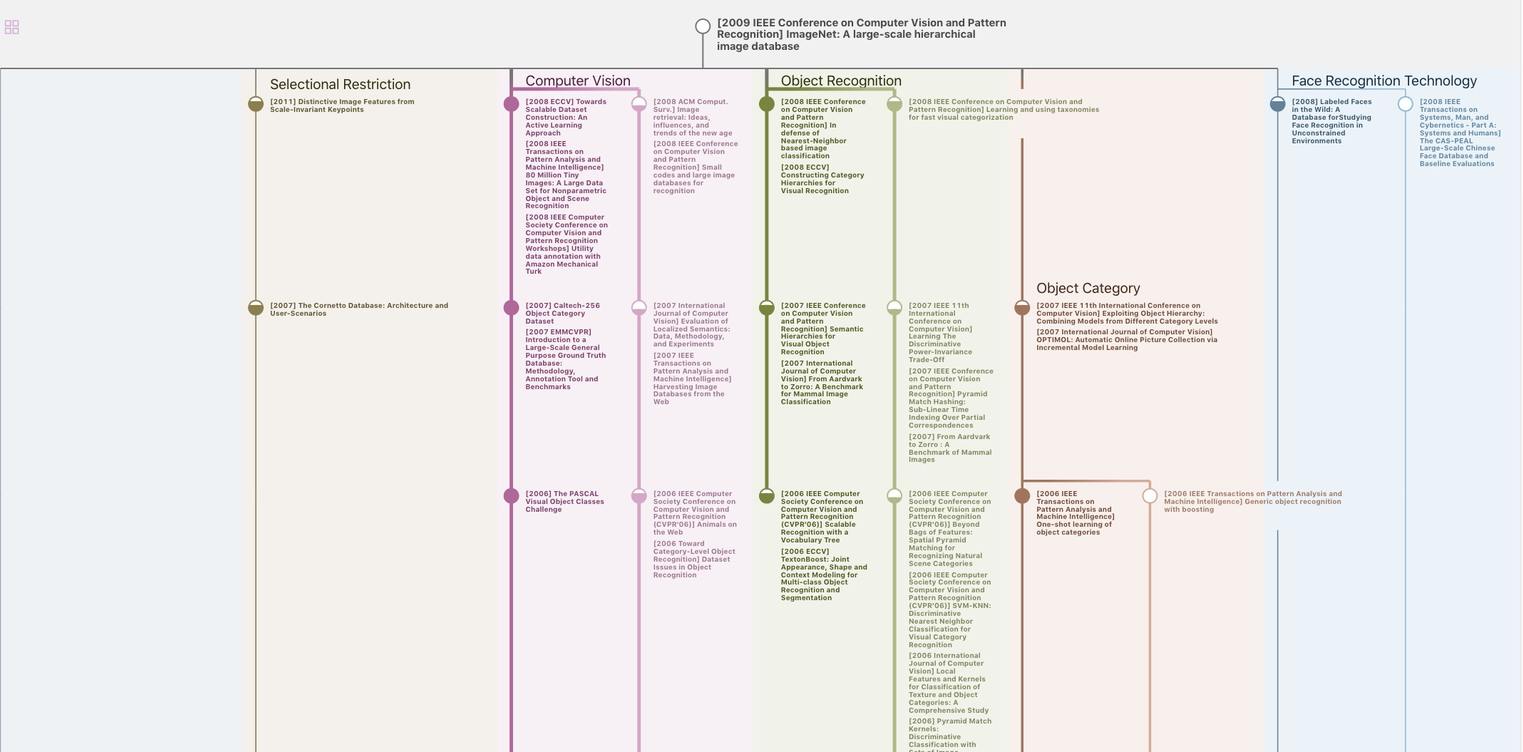
生成溯源树,研究论文发展脉络
Chat Paper
正在生成论文摘要