An Interpretable Approach for Lung Cancer Prediction and Subtype Classification using Gene Expression
2021 43RD ANNUAL INTERNATIONAL CONFERENCE OF THE IEEE ENGINEERING IN MEDICINE & BIOLOGY SOCIETY (EMBC)(2021)
Abstract
Lung cancer is the deadliest form of cancer, accounting for 20% of total cancer deaths. It represents a group of histologically and molecularly heterogeneous diseases even within the same histological subtype. Moreover, accurate histological subtype diagnosis influences the specific subtype's target genes, which will help define the treatment plan to target those genes in therapy. Deep learning (DL) models seem to set the benchmarks for the tasks of cancer prediction and subtype classification when using gene expression data; however, these methods do not provide interpretability, which is great concern from the perspective of cancer biology since the identification of the cancer driver genes in an individual provides essential information for treatment and prognosis. In this work, we identify some limitations of previous work that showed efforts to build algorithms to extract feature weights from DL models, and we propose using tree-based learning algorithms that address these limitations. Preliminary results show that our methods outperform those of related research while providing model interpretability.
MoreTranslated text
Key words
lung cancer prediction,subtype classification,lung cancer,gene expression
AI Read Science
Must-Reading Tree
Example
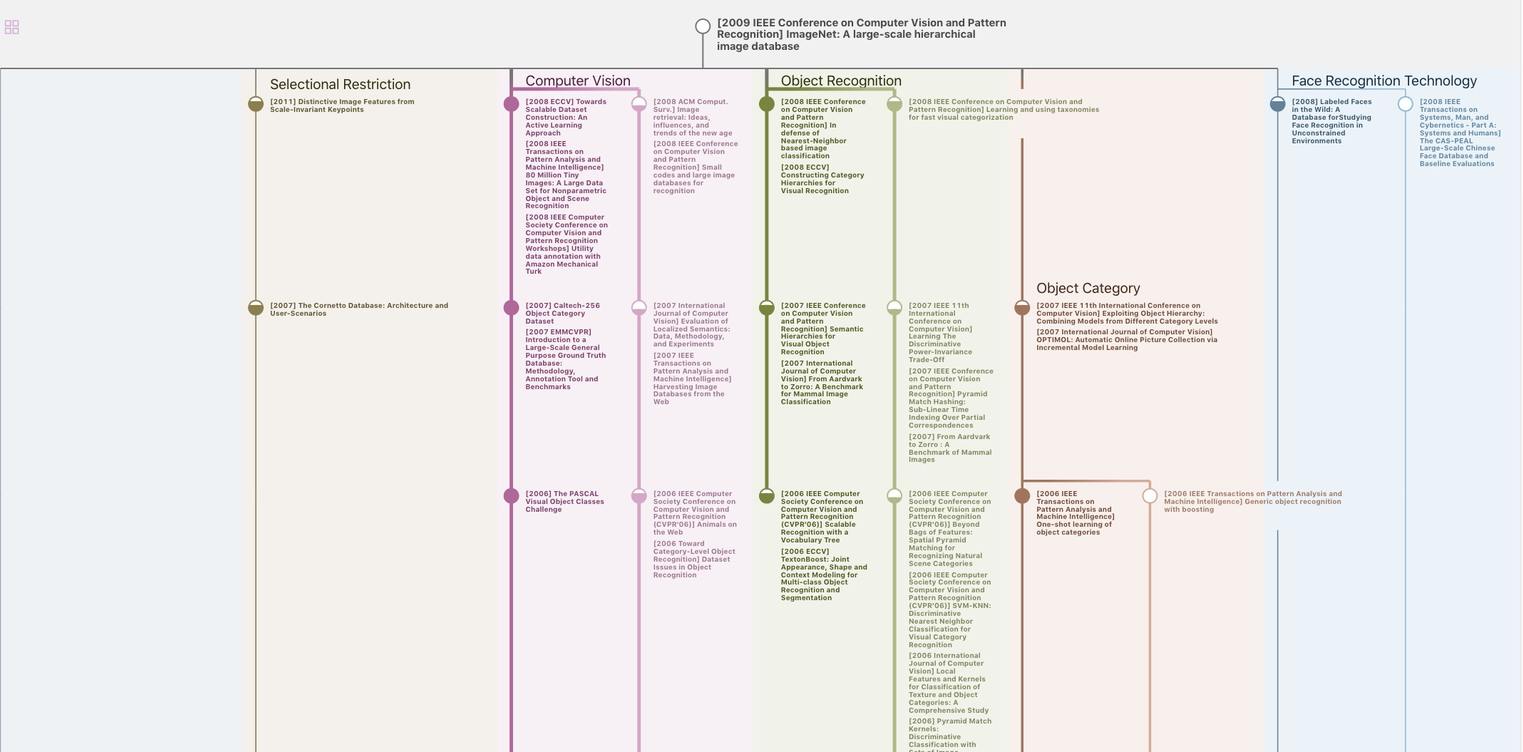
Generate MRT to find the research sequence of this paper
Chat Paper
Summary is being generated by the instructions you defined