Deep Learning based non-invasive diabetes predictor using Photoplethysmography signals
29TH EUROPEAN SIGNAL PROCESSING CONFERENCE (EUSIPCO 2021)(2021)
摘要
In 2016, World Health Organization (WHO) estimated that diabetes is the seventh leading cause of death causing 1.6 million casualties globally. In this paper, we propose a non-invasive solution through a Convolutional Neural Network (CNN)-based Deep Learning classifier. We use the scalograms generated out of transmissive Photoplethysmography (PPG) signals collected from MIMIC-III database to diagnose diabetes. Different sets of inputs were sent into a slightly modified VGGNet model, which were trained over data from 584 patients. We provide a probabilistic score of diabetes for every patient, which is further used for classifying patients into diabetic and nondiabetic. The best model obtained using a combination of PPG signals, hypertension classification, age and gender as inputs produced an accuracy of 76.34% and area under the curve (AUC) of 0.830 on 224 test patients. In our knowledge, this is among the first CNN-based approaches in the literature to detect diabetes using MIMIC-III waveforms dataset with a good performance.
更多查看译文
关键词
Photoplethysmography (PPG), Vital Signs Monitoring (VSM), Convolutional Neural Networks (CNN), MIMIC, Scalogram
AI 理解论文
溯源树
样例
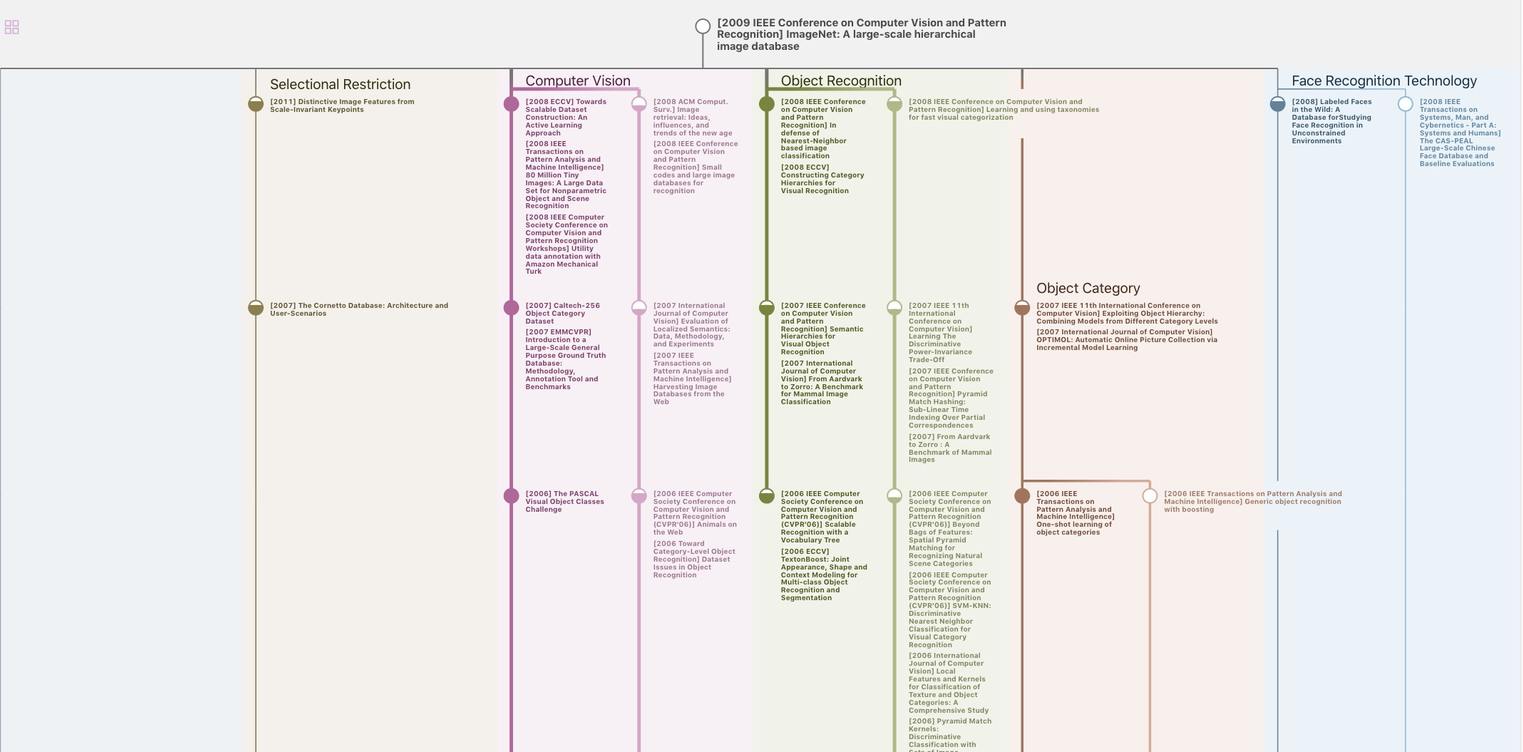
生成溯源树,研究论文发展脉络
Chat Paper
正在生成论文摘要