Online RL in the programmable dataplane with OPaL
International Conference On Emerging Networking Experiments And Technologies(2021)
摘要
ABSTRACTReinforcement learning (RL) is a key tool in data-driven networking for learning to control systems online. While recent research has shown how to offload machine learning tasks to the dataplane (reducing processing latency), online learning remains an open challenge unless the model is moved back to a host CPU, harming latency-sensitive applications. Our poster introduces OPaL---On Path Learning---the first work to bring online reinforcement learning to the dataplane. OPaL makes online learning possible in SmartNIC/NPU hardware by returning to classical RL techniques---avoiding neural networks. This simplifies update logic, enabling online learning, and benefits well from the parallelism common to SmartNICs. We show that our implementation on Netronome SmartNIC hardware offers concrete latency improvements over host execution.
更多查看译文
AI 理解论文
溯源树
样例
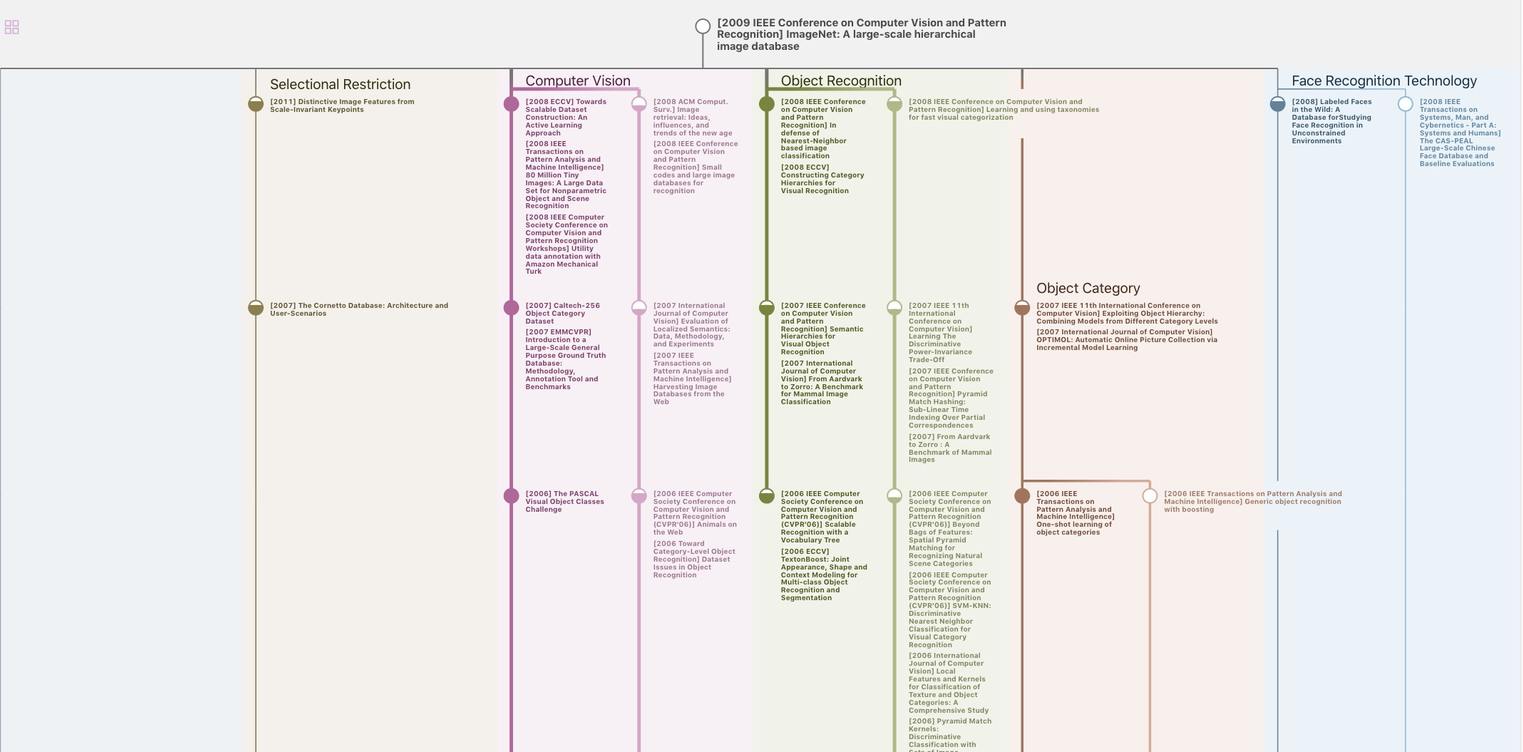
生成溯源树,研究论文发展脉络
Chat Paper
正在生成论文摘要