GRAF: a graph neural network based proactive resource allocation framework for SLO-oriented microservices
CONEXT(2021)
Abstract
ABSTRACTMicroservice is an architectural style that has been widely adopted in various latency-sensitive applications. Similar to the monolith, autoscaling has attracted the attention of operators for managing resource utilization of microservices. However, it is still challenging to optimize resources in terms of latency service-level-objective (SLO) without human intervention. In this paper, we present GRAF, a graph neural network-based proactive resource allocation framework for minimizing total CPU resources while satisfying latency SLO. GRAF leverages front-end workload, distributed tracing data, and machine learning approaches to (a) observe/estimate impact of traffic change (b) find optimal resource combinations (c) make proactive resource allocation. Experiments using various open-source benchmarks demonstrate that GRAF successfully targets latency SLO while saving up to 19% of total CPU resources compared to the fine-tuned autoscaler. Moreover, GRAF handles traffic surge with 36% fewer resources while achieving up to 2.6x faster tail latency convergence compared to the Kubernetes autoscaler.
MoreTranslated text
AI Read Science
Must-Reading Tree
Example
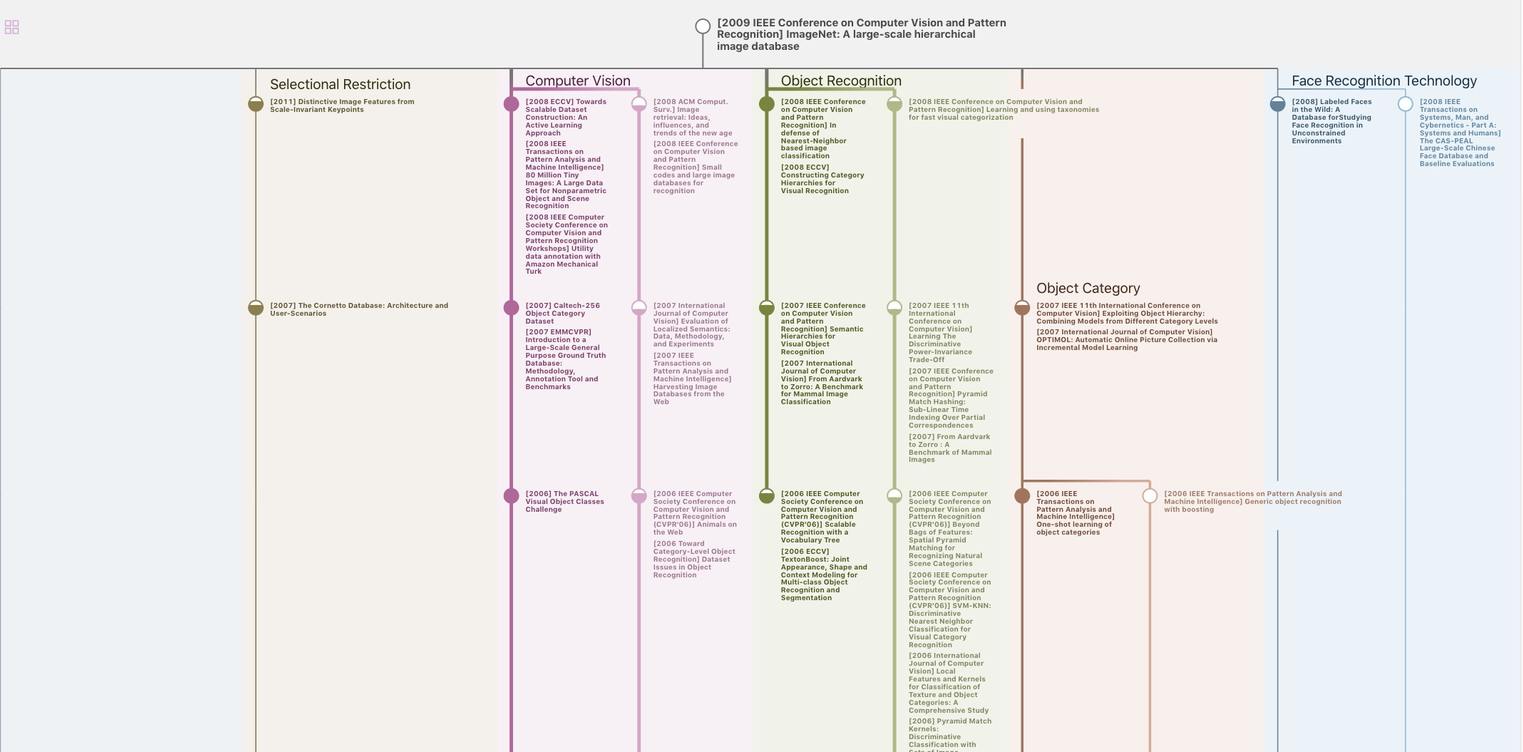
Generate MRT to find the research sequence of this paper
Chat Paper
Summary is being generated by the instructions you defined