Saliency-Guided Transformer Network combined with Local Embedding for No-Reference Image Quality Assessment.
IEEE International Conference on Computer Vision(2021)
摘要
No-Reference Image Quality Assessment (NR-IQA) methods based on Vision Transformer have recently drawn much attention for their superior performance. Unfortunately being a crude combination of NR-IQA and Transformer, they can hardly take the advantage of their strengths. In this paper, we propose a novel Saliency-Guided Transformer Network combined with Local Embedding (TranSLA) for No-Reference Image Quality Assessment. Our TranSLA integrates different-level information for a robust representation. Existed researches have shown that the human vision system concentrates more on the Region-of-interest (Rol) when assessing the image quality. Thus we combine saliency prediction with Transformer to guide the model highlight the RoI when aggregating the global information. Besides, we import local embedding for Transformer with gradient map. Since the gradient map focuses on extracting structured feature in detail, it can be used as a supplement to offer local information for Transformer. Then, the local and non-local information can be utilized. Moreover, to accelerate the aggregation of information from all tokens, we introduce a Boosting Interaction Module (BIM) to enhance feature aggregation. BIM forces patch tokens to interact better with class tokens at all levels. Experiments on two large-scale NR-IQA benchmarks demonstrate that our method significantly outperforms the state-of-the-art.
更多查看译文
关键词
TranSLA,human vision system,saliency prediction,local embedding,gradient map,local information,large-scale NR-IQA benchmarks,no-reference image quality assessment methods,vision transformer,crude combination,saliency-guided transformer network,feature aggregation,region-of-interest,BIM,boosting interaction module
AI 理解论文
溯源树
样例
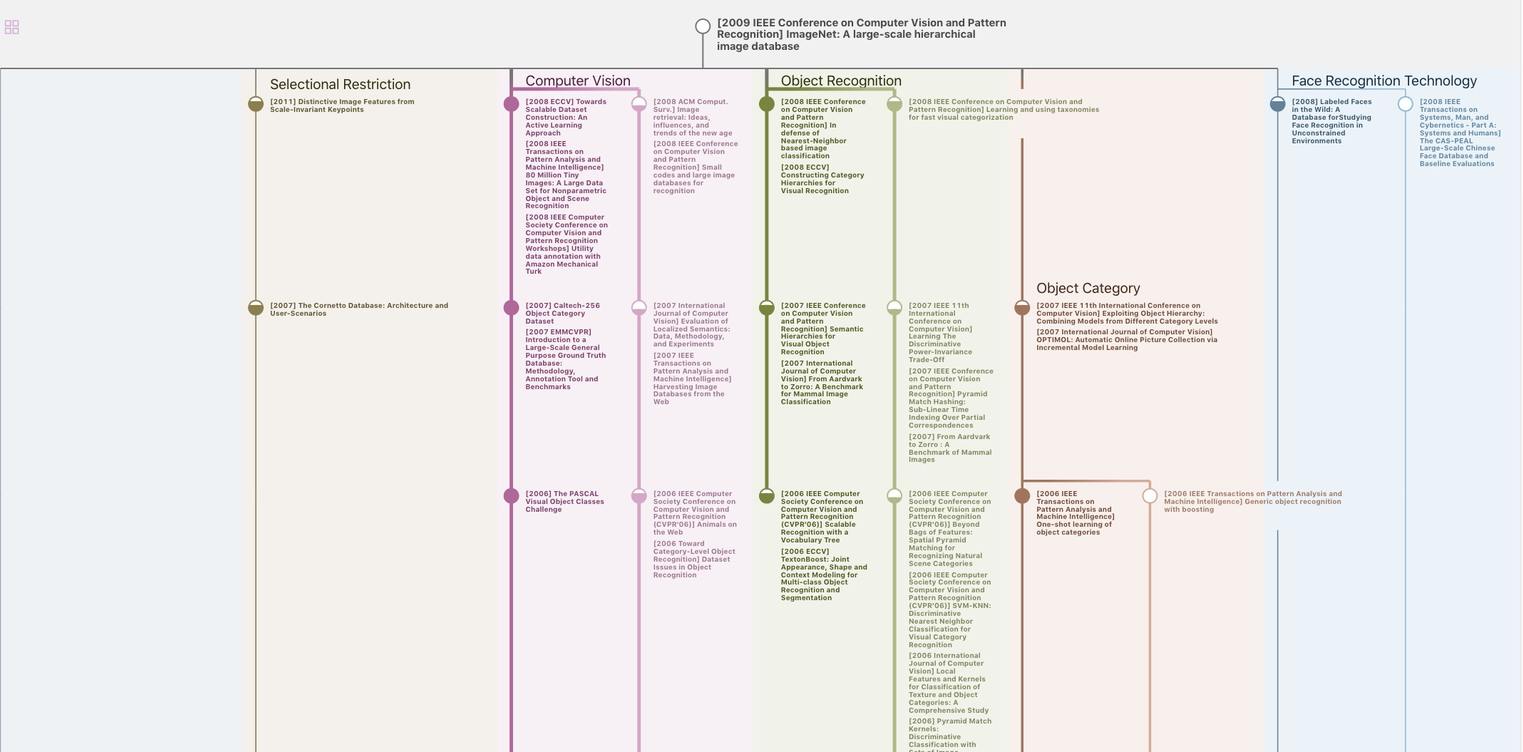
生成溯源树,研究论文发展脉络
Chat Paper
正在生成论文摘要