CELA: An Accurate Learned Cardinality Estimator with Strong Generalization Ability and Dimensional Adaptability
WISE(2021)
Abstract
Accurate cardinality estimation contributes significantly to query optimization whereas traditional approaches such as histogram-based or sketching-based approaches relying on assumption of uniform distribution of data and appropriate pre-set parameters, often leading to dilemma in practical applications. In this paper, an accurate lightweight, dimensionally adaptive, strongly generalizable learned cardinality estimator for multi-dimensional range queries, CELA is proposed reflecting on the characteristics of desirable cardinality estimators. For the purpose of capturing relationship between dimensions, CELA raises a query-oriented approach of constructing constraint matrices to apply convolution. Experiments illustrates that CELA performs superbly on each defined indicators far superior to PostgreSQL. Furthermore, the strong generalization ability of CELA is demonstrated by the excellent performance trained with continuously scaled-down training set.
MoreTranslated text
Key words
Cardinality estimation,Dynamic architecture,Deep learning,Generalization ability,Dimensional adaptability
AI Read Science
Must-Reading Tree
Example
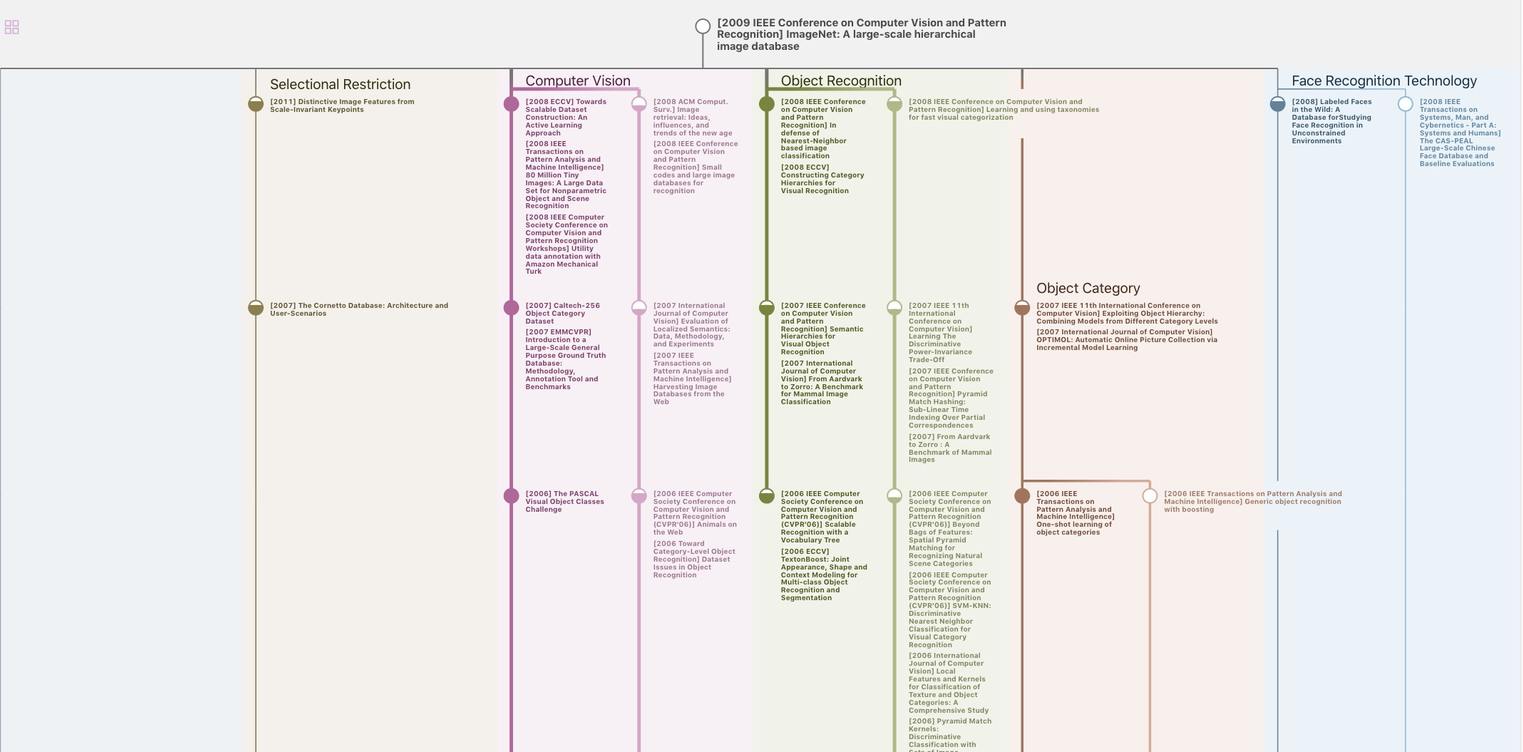
Generate MRT to find the research sequence of this paper
Chat Paper
Summary is being generated by the instructions you defined