Genome-Wide Mutation Scoring for Machine-Learning-Based Antimicrobial Resistance Prediction
INTERNATIONAL JOURNAL OF MOLECULAR SCIENCES(2021)
摘要
The prediction of antimicrobial resistance (AMR) based on genomic information can improve patient outcomes. Genetic mechanisms have been shown to explain AMR with accuracies in line with standard microbiology laboratory testing. To translate genetic mechanisms into phenotypic AMR, machine learning has been successfully applied. AMR machine learning models typically use nucleotide k-mer counts to represent genomic sequences. While k-mer representation efficiently captures sequence variation, it also results in high-dimensional and sparse data. With limited training data available, achieving acceptable model performance or model interpretability is challenging. In this study, we explore the utility of feature engineering with several biologically relevant signals. We propose to predict the functional impact of observed mutations with PROVEAN to use the predicted impact as a new feature for each protein in an organism's proteome. The addition of the new features was tested on a total of 19,521 isolates across nine clinically relevant pathogens and 30 different antibiotics. The new features significantly improved the predictive performance of trained AMR models for Pseudomonas aeruginosa, Citrobacter freundii, and Escherichia coli. The balanced accuracy of the respective models of those three pathogens improved by 6.0% on average.
更多查看译文
关键词
machine learning, genomics, antimicrobial resistance, antibiotics, WGS, genome-wide mutation scoring
AI 理解论文
溯源树
样例
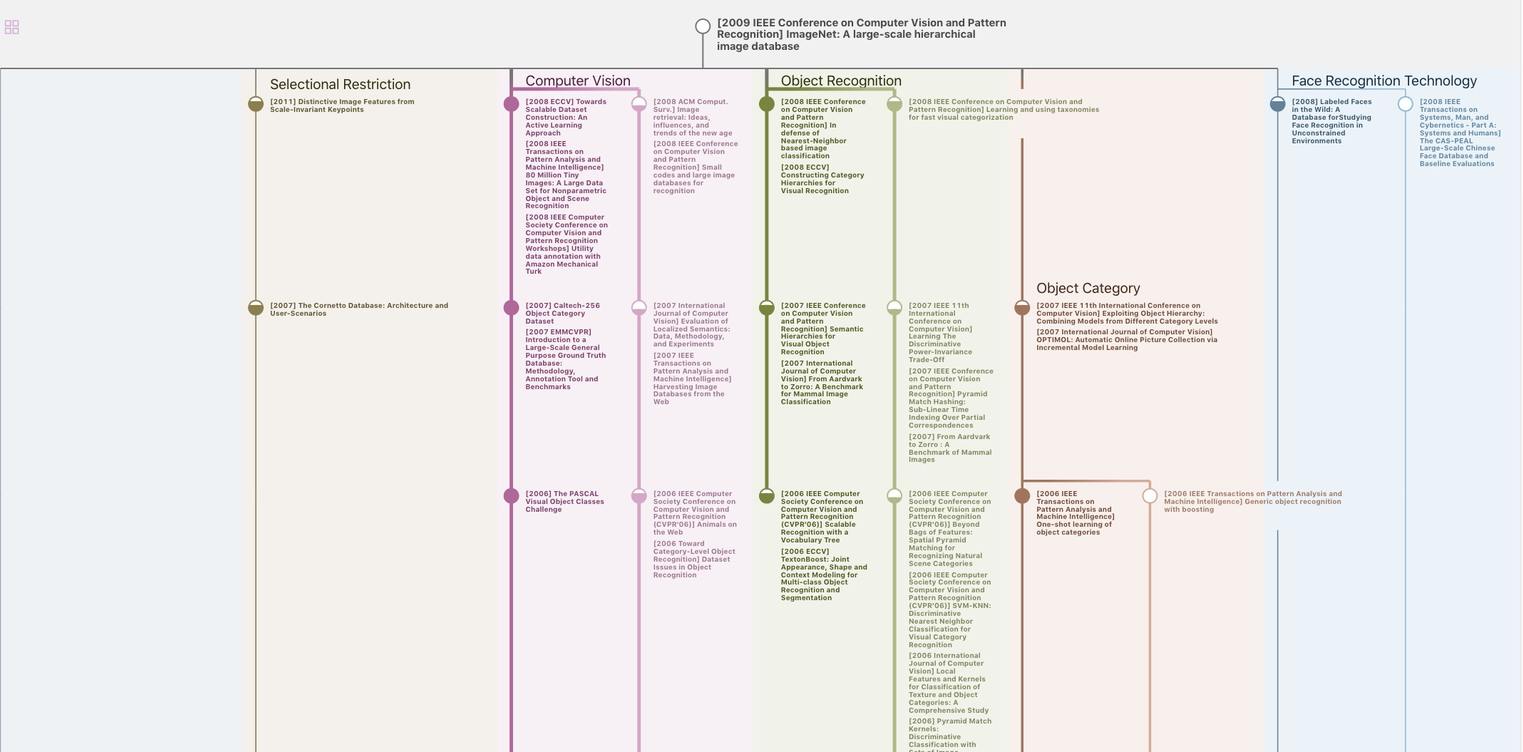
生成溯源树,研究论文发展脉络
Chat Paper
正在生成论文摘要