Understanding Patient Query With Weak Supervision From Doctor Response
IEEE Journal of Biomedical and Health Informatics(2022)
摘要
Currently, the need for high-quality dialogue systems that assist users to conduct self-diagnosis is rapidly increasing. Slot filling for automatic diagnosis, which converts medical queries into structured representations, plays an important role in diagnostic dialogue systems. However, the lack of high-quality datasets limits the performance of slot filling. While medical communities like AskAPatient usually have multiple rounds of diagnostic dialogue containing colloquial input and professional responses from doctors. Therefore, the data of diagnostic dialogue in medical communities can be utilized to solve the main challenges in slot filling. This paper proposes a two-step training framework to make full use of these unlabeled dialogue data in medical communities. To promote further researches, we provide a Chinese dataset with 2,652 annotated samples and a large amount of unlabeled samples. Experimental results on the dataset demonstrate the effectiveness of the proposed method with an increase of 6.32% in Micro F1 and 8.20% in Macro F1 on average over strong baselines.
更多查看译文
关键词
Self-diagnosis,task-oriented dialogue system,slot filling
AI 理解论文
溯源树
样例
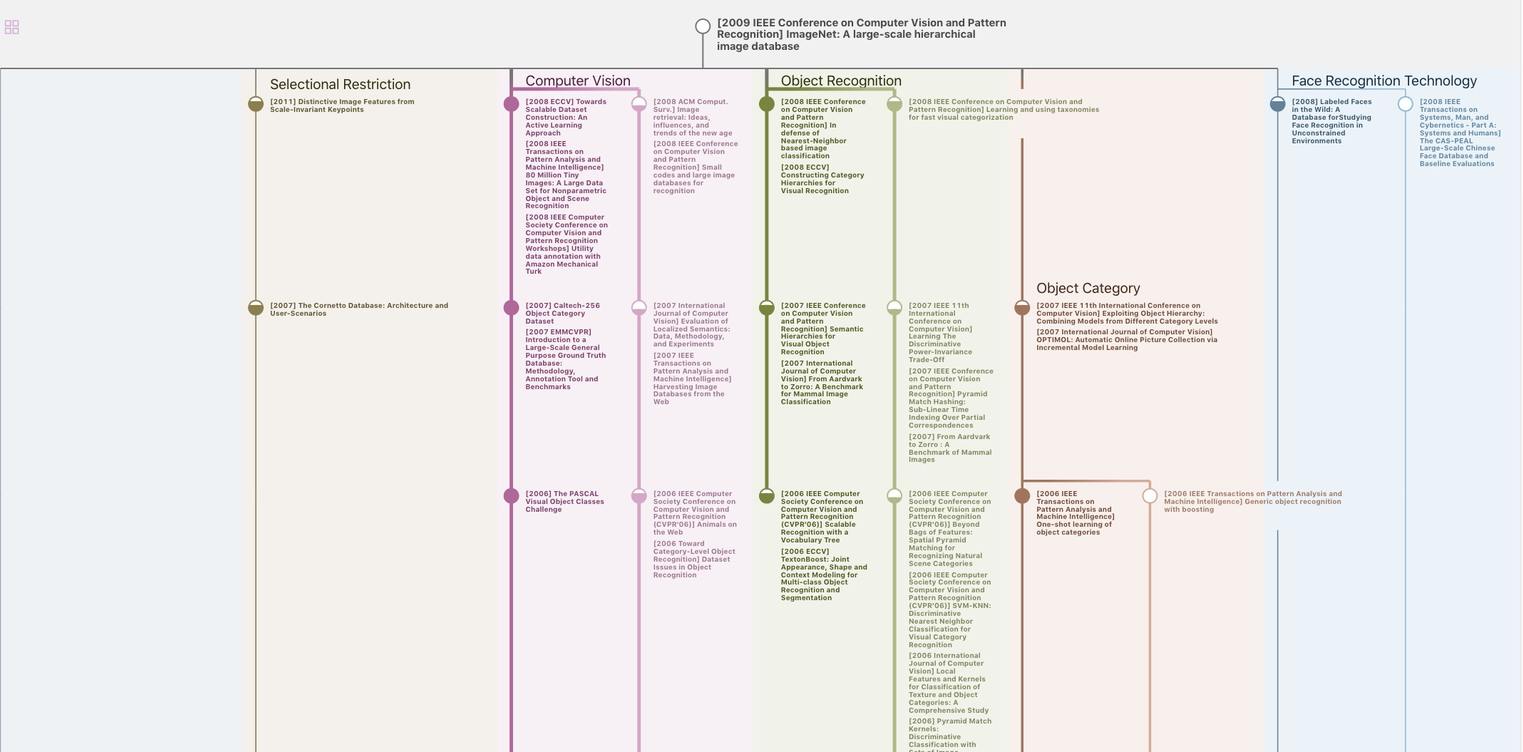
生成溯源树,研究论文发展脉络
Chat Paper
正在生成论文摘要