Development of an experiment-split method for benchmarking the generalization of a PTM site predictor: Lysine methylome as an example.
PLoS Comput. Biol.(2021)
摘要
Many computational classifiers have been developed to predict different types of post-translational modification sites. Their performances are measured using cross-validation or independent test, in which experimental data from different sources are mixed and randomly split into training and test sets. However, the self-reported performances of most classifiers based on this measure are generally higher than their performances in the application of new experimental data. It suggests that the cross-validation method overestimates the generalization ability of a classifier. Here, we proposed a generalization estimate method, dubbed experiment-split test, where the experimental sources for the training set are different from those for the test set that simulate the data derived from a new experiment. We took the prediction of lysine methylome (Kme) as an example and developed a deep learning-based Kme site predictor (called DeepKme) with outstanding performance. We assessed the experiment-split test by comparing it with the cross-validation method. We found that the performance measured using the experiment-split test is lower than that measured in terms of cross-validation. As the test data of the experiment-split method were derived from an independent experimental source, this method could reflect the generalization of the predictor. Therefore, we believe that the experiment-split method can be applied to benchmark the practical performance of a given PTM model. DeepKme is free accessible via https://github.com/guoyangzou/DeepKme.
更多查看译文
关键词
ptm site predictor,lysine methylome,experiment-split
AI 理解论文
溯源树
样例
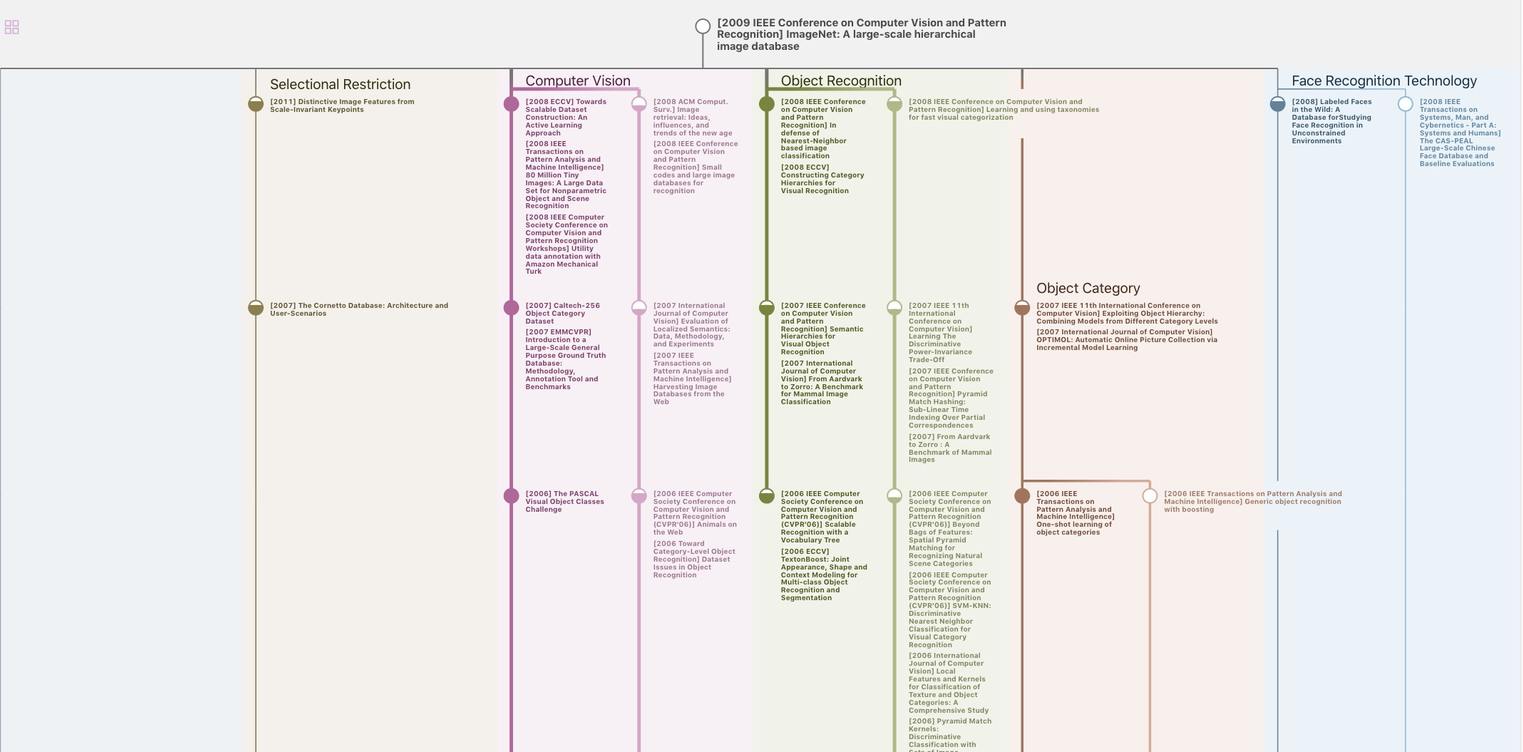
生成溯源树,研究论文发展脉络
Chat Paper
正在生成论文摘要