SGNNMD: signed graph neural network for predicting deregulation types of miRNA-disease associations
BRIEFINGS IN BIOINFORMATICS(2022)
摘要
MiRNAs are a class of small non-coding RNA molecules that play an important role in many biological processes, and determining miRNA-disease associations can benefit drug development and clinical diagnosis. Although great efforts have been made to develop miRNA-disease association prediction methods, few attention has been paid to in-depth classification of miRNA-disease associations, e.g. up/down-regulation of miRNAs in diseases. In this paper, we regard known miRNA-disease associations as a signed bipartite network, which has miRNA nodes, disease nodes and two types of edges representing up/down-regulation of miRNAs in diseases, and propose a signed graph neural network method (SGNNMD) for predicting deregulation types of miRNA-disease associations. SGNNMD extracts subgraphs around miRNA-disease pairs from the signed bipartite network and learns structural features of subgraphs via a labeling algorithm and a neural network, and then combines them with biological features (i.e. miRNA-miRNA functional similarity and disease-disease semantic similarity) to build the prediction model. In the computational experiments, SGNNMD achieves highly competitive performance when compared with several baselines, including the signed graph link prediction methods, multi-relation prediction methods and one existing deregulation type prediction method. Moreover, SGNNMD has good inductive capability and can generalize to miRNAs/diseases unseen during the training.
更多查看译文
关键词
miRNA-disease associations, subgraph, graph convolutional network, signed network
AI 理解论文
溯源树
样例
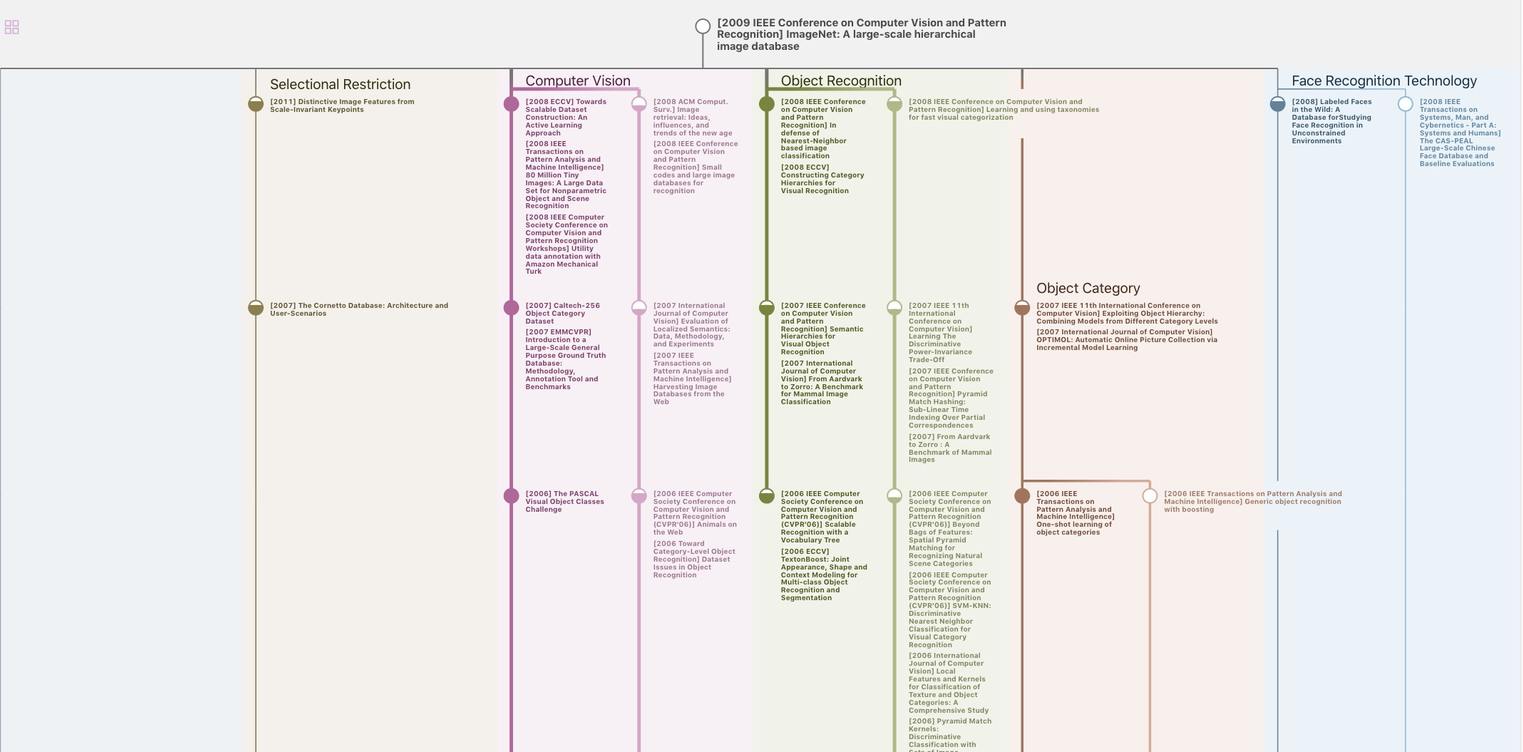
生成溯源树,研究论文发展脉络
Chat Paper
正在生成论文摘要