Segmentation of male pelvic organs on computed tomography with a deep neural network fine-tuned by a level-set method
COMPUTERS IN BIOLOGY AND MEDICINE(2022)
摘要
Computed Tomography (CT) imaging is used in Radiation Therapy planning, where the treatment is carefully tailored to each patient in order to maximize radiation dose to the target while decreasing adverse effects to nearby healthy tissues. A crucial step in this process is manual organ contouring, which if performed automatically could considerably decrease the time to starting treatment and improve outcomes. Computerized segmentation of male pelvic organs has been studied for decades and deep learning models have brought considerable advances to the field, but improvements are still demanded. A two-step framework for automatic segmentation of the prostate, bladder and rectum is presented: a convolutional neural network enhanced with attention gates performs an initial segmentation, followed by a region-based active contour model to fine-tune the segmentations to each patient's specific anatomy. The framework was evaluated on a large collection of planning CTs of patients who had Radiation Therapy for prostate cancer. The Surface Dice Coefficient improved from 79.41 to 81.00% on segmentation of the prostate, 94.03-95.36% on the bladder and 82.17-83.68% on the rectum, comparing the proposed framework with the baseline convolutional neural network. This study shows that traditional image segmentation algorithms can help improve the immense gains that deep learning models have brought to the medical imaging segmentation field.
更多查看译文
关键词
Deep learning, Convolutional neural networks, Deformable model, Radiation therapy, Prostate cancer, Computed tomography imaging
AI 理解论文
溯源树
样例
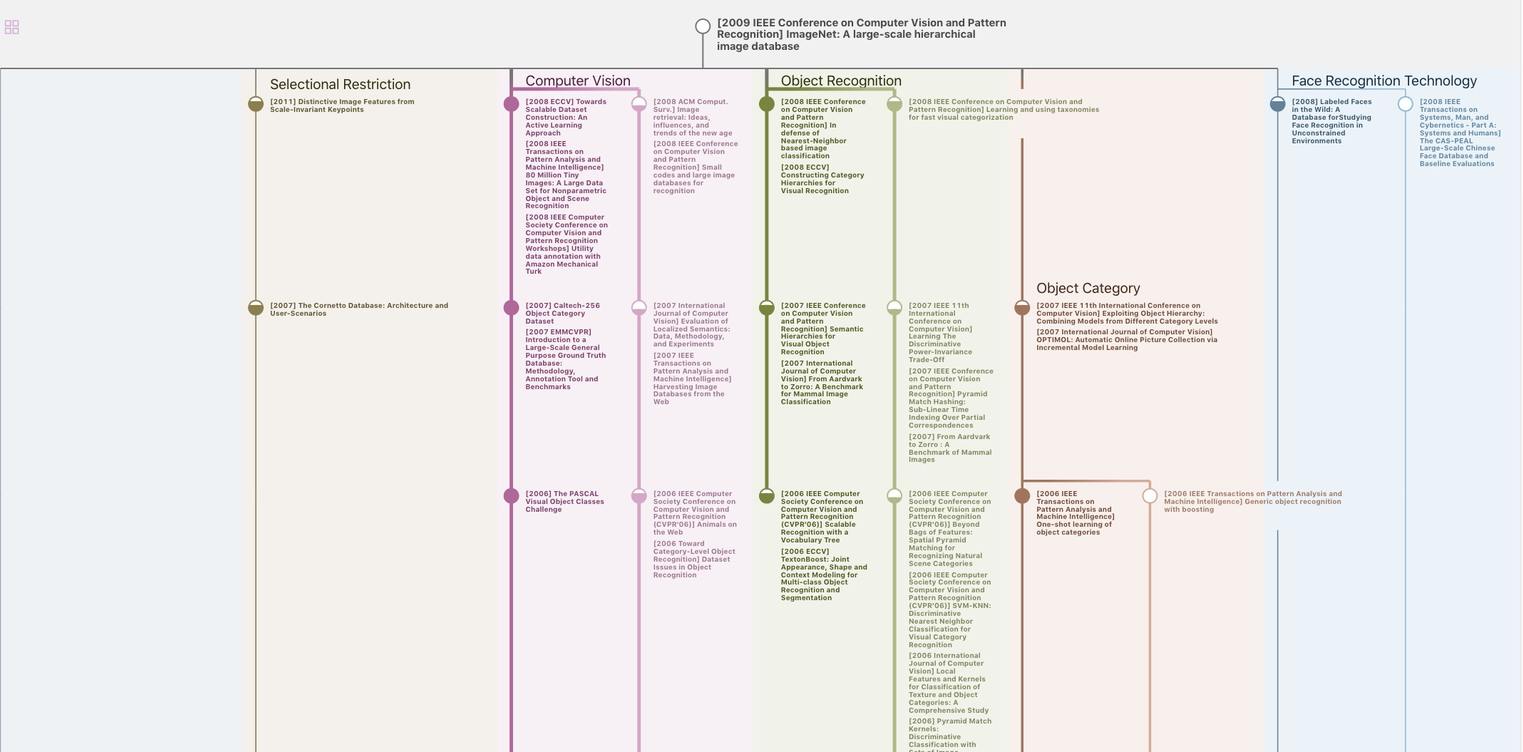
生成溯源树,研究论文发展脉络
Chat Paper
正在生成论文摘要