Exploring the vertical dimension of street view image based on deep learning: a case study on lowest floor elevation estimation
INTERNATIONAL JOURNAL OF GEOGRAPHICAL INFORMATION SCIENCE(2022)
摘要
Street view imagery such as Google Street View is widely used in people's daily lives. Many studies have been conducted to detect and map objects such as traffic signs and sidewalks for urban built-up environment analysis. While mapping objects in the horizontal dimension is common in those studies, automatic vertical measuring in large areas is underexploited. Vertical information from street view imagery can benefit a variety of studies. One notable application is estimating the lowest floor elevation, which is critical for building flood vulnerability assessment and insurance premium calculation. In this article, we explored the vertical measurement in street view imagery using the principle of tacheometric surveying. In the case study of lowest floor elevation estimation using Google Street View images, we trained a neural network (YOLO-v5) for door detection and used the fixed height of doors to measure doors' elevation. The results suggest that the average error of estimated elevation is 0.218 m. The depthmaps of Google Street View were utilized to traverse the elevation from the roadway surface to target objects. The proposed pipeline provides a novel approach for automatic elevation estimation from street view imagery and is expected to benefit future terrain-related studies for large areas.
更多查看译文
关键词
Street view, image, lowest floor elevation, vertical dimension, deep learning
AI 理解论文
溯源树
样例
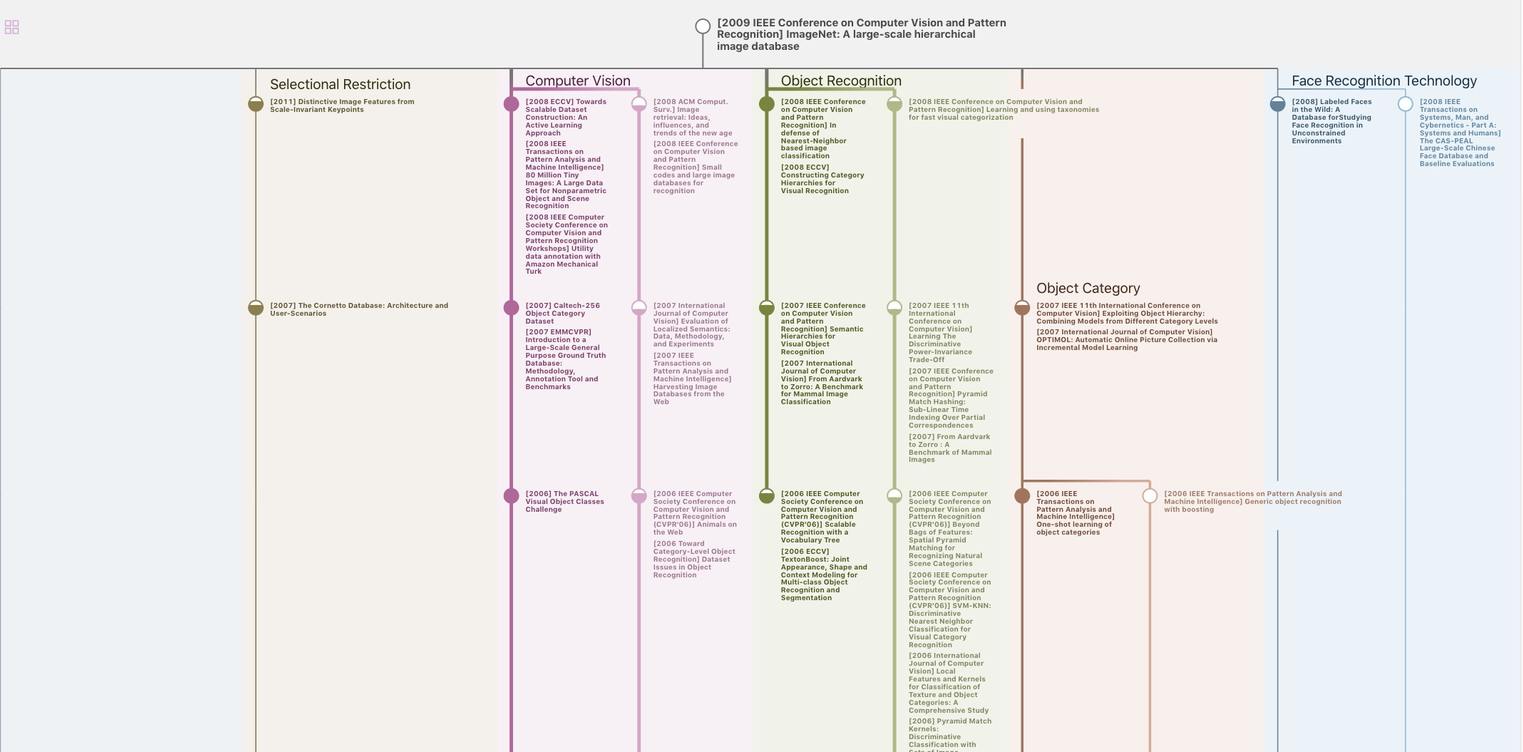
生成溯源树,研究论文发展脉络
Chat Paper
正在生成论文摘要