Zero-Shot Recommendation as Language Modeling
ADVANCES IN INFORMATION RETRIEVAL, PT II(2022)
摘要
Recommendation is the task of ranking items (e.g. movies or products) according to individual user needs. Current systems rely on collaborative filtering and content-based techniques, which both require structured training data. We propose a framework for recommendation with off-the-shelf pretrained language models (LM) that only used unstructured text corpora as training data. If a user u liked Matrix and Inception, we construct a textual prompt, e.g. "Movies like Matrix, Inception, " to estimate the affinity between u and m with LM likelihood. We motivate our idea with a corpus analysis, evaluate several prompt structures, and we compare LM-based recommendation with standard matrix factorization trained on different data regimes. The code for our experiments is publicly available (https://colabsesearch.google.com/drive/...?usp=sharing).
更多查看译文
关键词
language,zero-shot
AI 理解论文
溯源树
样例
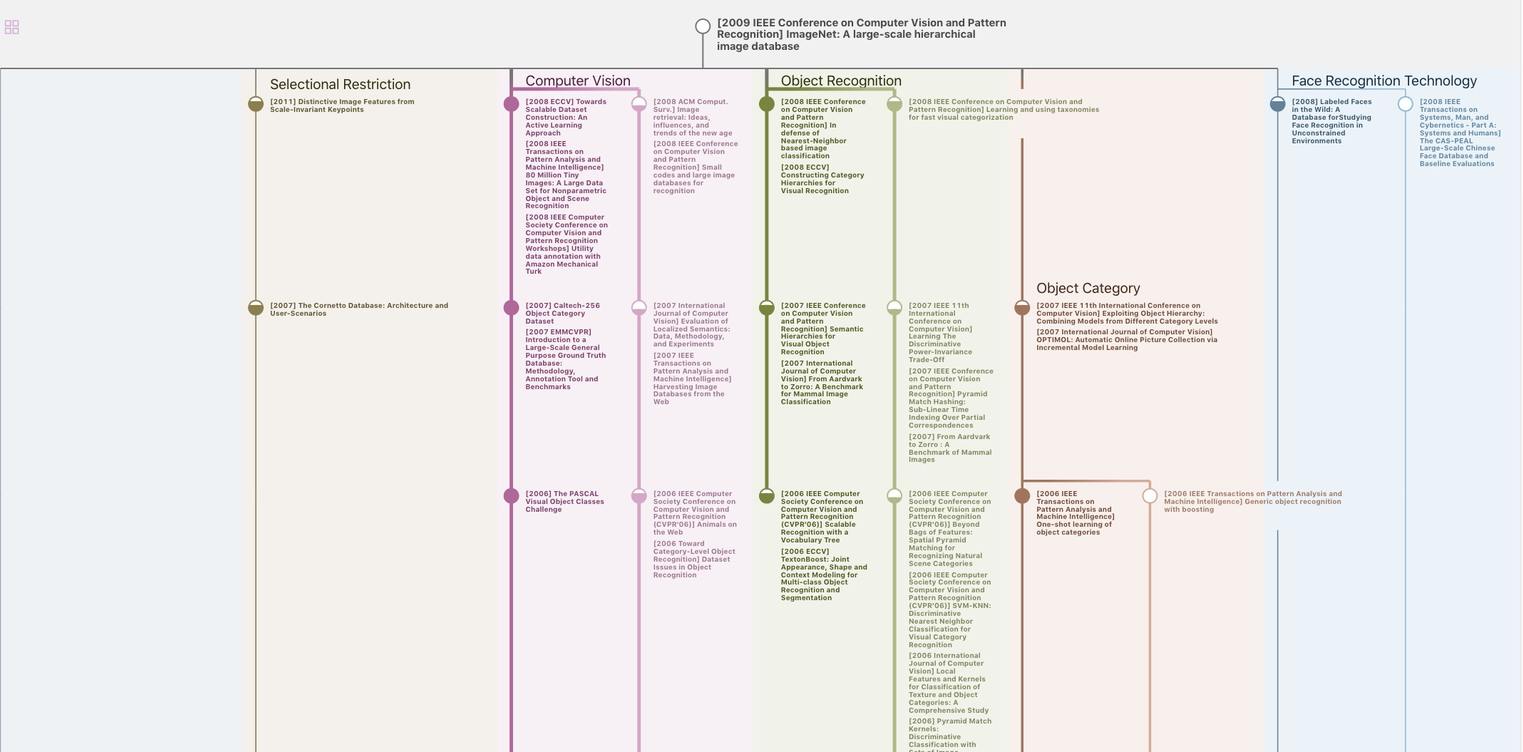
生成溯源树,研究论文发展脉络
Chat Paper
正在生成论文摘要