The Spectroscopic Binaries from the LAMOST Medium-resolution Survey. I. Searching for Double-lined Spectroscopic Binaries with a Convolutional Neural Network
ASTROPHYSICAL JOURNAL SUPPLEMENT SERIES(2022)
摘要
We developed a convolutional neural network model to distinguish the double-lined spectroscopic binaries (SB2s) from others based on single-exposure medium-resolution spectra (R similar to 7500). The training set consists of a large set of mock spectra of single stars and binaries synthesized based on the MIST stellar evolutionary model and ATLAS9 atmospheric model. Our model reaches a novel theoretic false-positive rate by adding a proper penalty on the negative sample (e.g., 0.12% and 0.16% for the blue/red arm when the penalty parameter ? = 16). Tests show that the performance is as expected and favors FGK-type main-sequence (MS) binaries with high mass ratio (q >= 0.7) and large radial velocity separation (Delta v >= 50 km s(-1)). Although the real false-positive rate cannot be estimated reliably, validating on eclipsing binaries identified from Kepler light curves indicates that our model predicts low binary probabilities at eclipsing phases (0, 0.5, and 1.0) as expected. The color-magnitude diagram also helps illustrate its feasibility and capability of identifying FGK MS binaries from spectra. We conclude that this model is reasonably reliable and can provide an automatic approach to identify SB2s with period less than or similar to 10 days. This work yields a catalog of binary probabilities for over 5 million spectra of 1 million sources from the LAMOST medium-resolution survey (MRS) and a catalog of 2198 SB2 candidates whose physical properties will be analyzed in a follow-up paper. Data products are made publicly available online, as well as our Github website.
更多查看译文
AI 理解论文
溯源树
样例
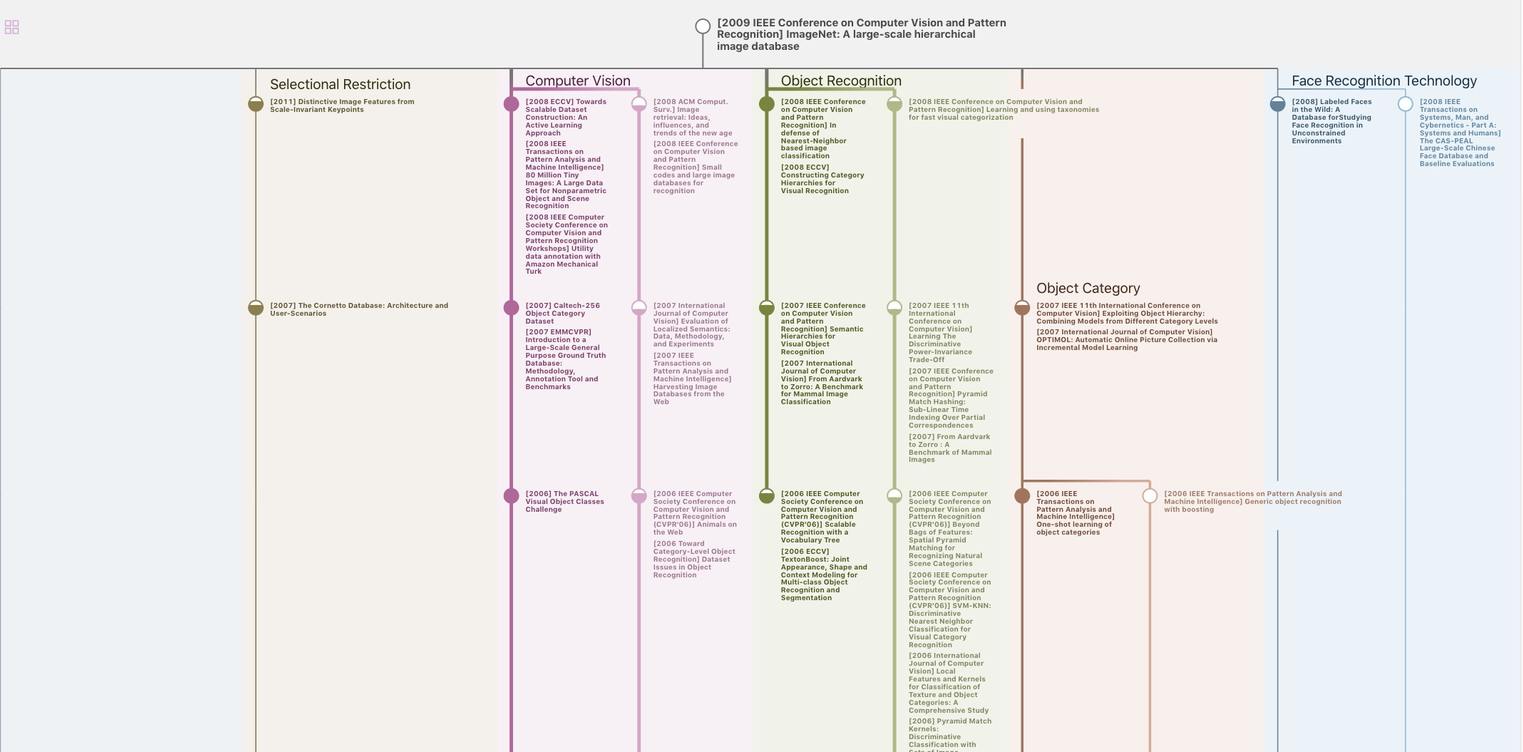
生成溯源树,研究论文发展脉络
Chat Paper
正在生成论文摘要