Accuracy of CREST Guideline in Management of Cellulitis in Emergency Department; a Systematic Review and Meta-analysis
ARCHIVES OF ACADEMIC EMERGENCY MEDICINE(2021)
摘要
Introduction: Skin and soft tissue infections are important causes of outpatient visits to medical clinics or hospitals. This study aimed to review the literature for the accuracy of Clinical Resource Efficiency Support Team (CREST) guideline in management of cellulitis in emergency department. Methods: Studies that had evaluated cellulitis patients using the CREST guideline were quarried in Scopus, Web of Science, and PubMed database, from 2005 to the end of 2020. The quality of the studies was evaluated using Scottish Intercollegiate Guideline Network (SIGN) checklist for cohort studies. Pooled area under the receiver operating characteristic curve (AUROC) of CREST guideline regarding the rate of hospital stay more than 24 hours, rate of revisit, and appropriateness of antimicrobial treatment in management of cellulitis in emergency department was evaluated. Results: Seven studies evaluating a total of 1640 adult cellulitis patients were finally entered to the study. In evaluation of the rate of the appropriate treatment versus over-treatment, the pooled AUROC was estimated to be 0.38 (95% confidence interval (CI): 0.06 - 0.82), indicating low accuracy (AUROC lower than 0.5) of guideline for antimicrobial choice. CREST II patients had a significantly lower odds ratio (OR) of revisiting the Emergency Department, OR=0.21 (95% CI: 0.009 - 0.47). Pooled AUROC value of 0.86 (CI95%: 0.84 - 0.89) showed accuracy of the CREST classification in prediction of being hospitalized more or less than 24 hours. Conclusion: CREST classification shows good accuracy in determining the duration of hospitalization or observation in ED but it could lead to inevitable over/under treatment with empirical antimicrobial agents.
更多查看译文
关键词
Cellulitis, emergency service, hospital, systematic review, skin diseases, bacterial, anti-bacterial agents
AI 理解论文
溯源树
样例
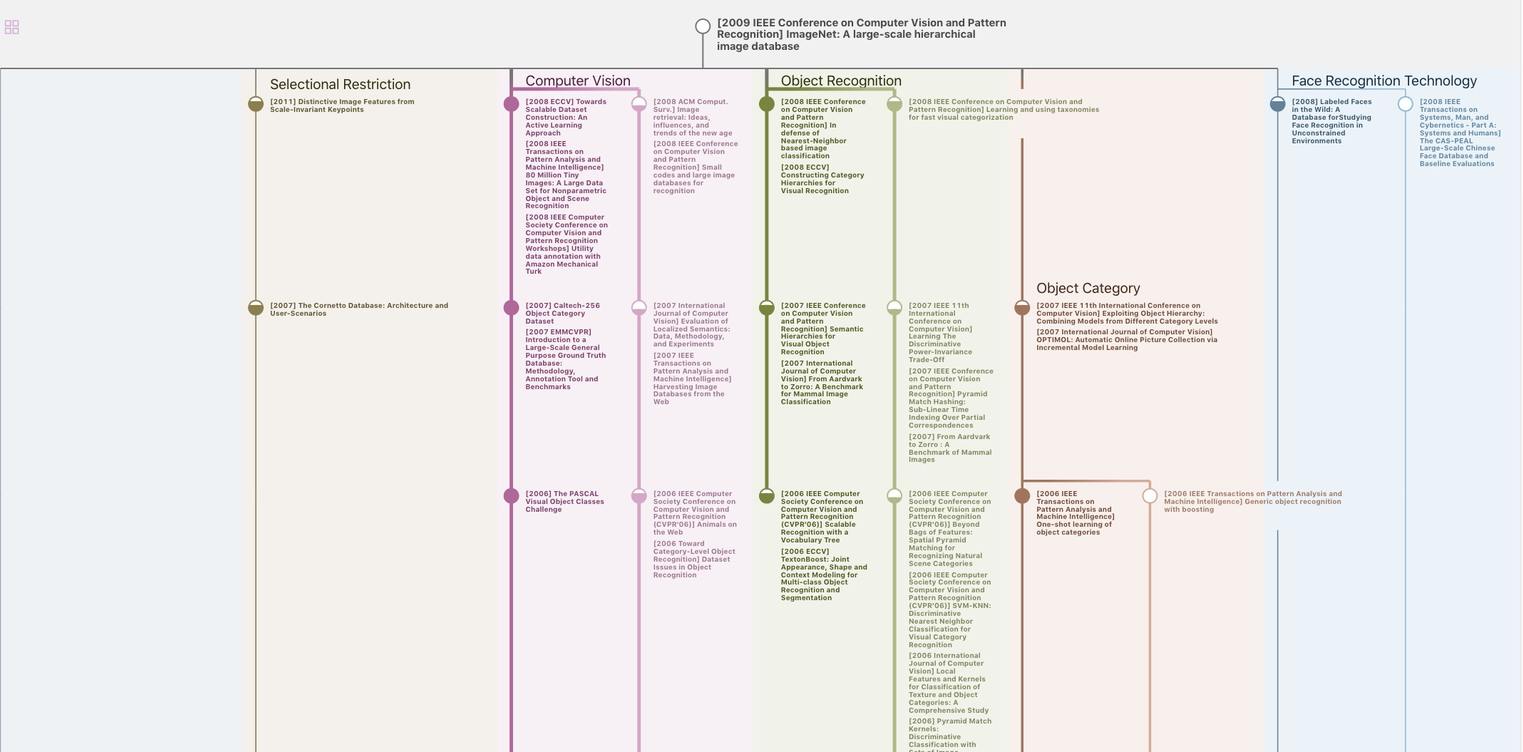
生成溯源树,研究论文发展脉络
Chat Paper
正在生成论文摘要