Identifying correlations driven by influential observations in large datasets
BRIEFINGS IN BIOINFORMATICS(2022)
摘要
Although high-throughput data allow researchers to interrogate thousands of variables simultaneously, it can also introduce a significant number of spurious results. Here we demonstrate that correlation analysis of large datasets can yield numerous false positives due to the presence of outliers that canonical methods fail to identify. We present Correlations Under The InfluencE (CUTIE), an open-source jackknifing-based method to detect such cases with both parametric and non-parametric correlation measures, and which can also uniquely rescue correlations not originally deemed significant or with incorrect sign. Our approach can additionally be used to identify variables or samples that induce these false correlations in high proportion. A meta-analysis of various omics datasets using CUTIE reveals that this issue is pervasive across different domains, although microbiome data are particularly susceptible to it. Although the significance of a correlation eventually depends on the thresholds used, our approach provides an efficient way to automatically identify those that warrant closer examination in very large datasets.
更多查看译文
关键词
correlation analysis, statistics, microbiome, multiomic analysis
AI 理解论文
溯源树
样例
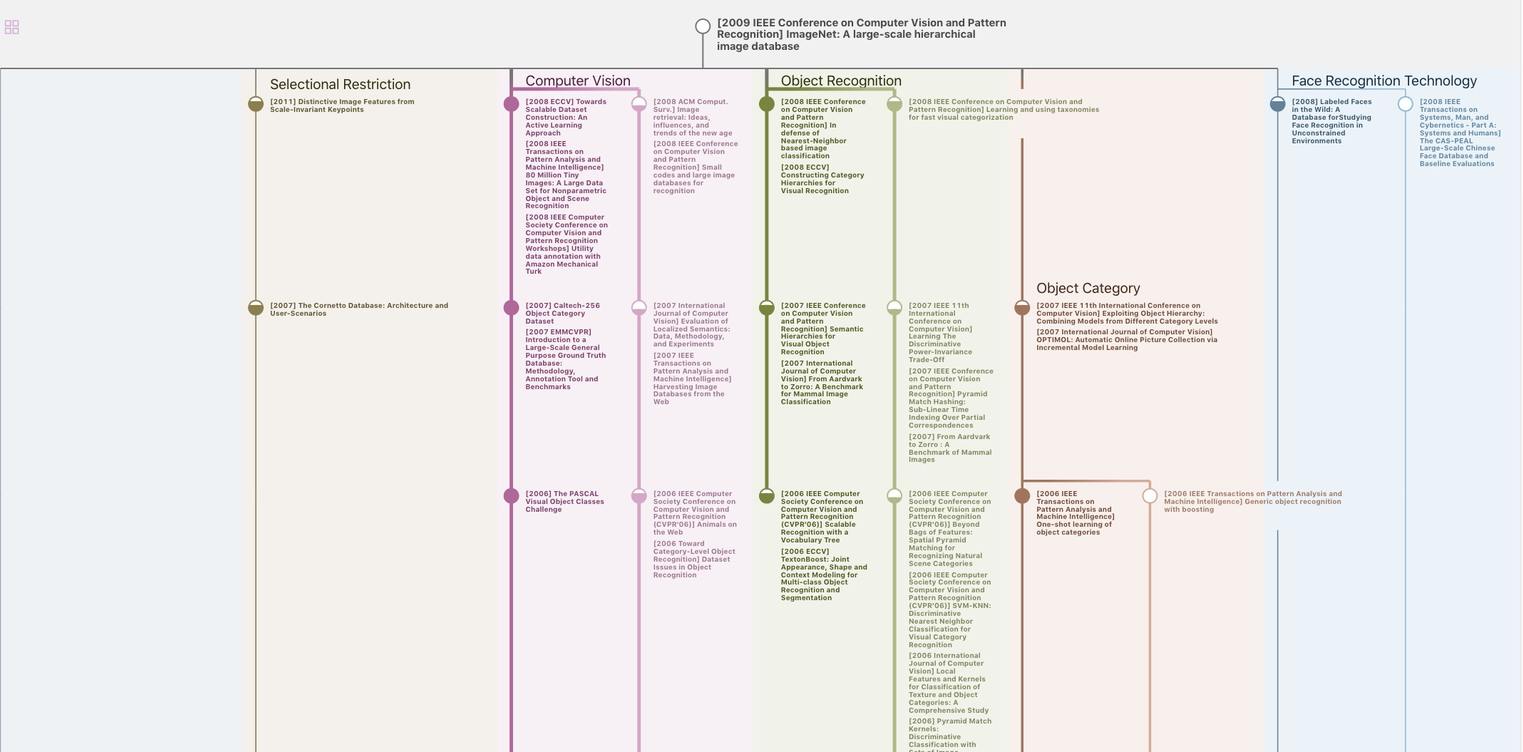
生成溯源树,研究论文发展脉络
Chat Paper
正在生成论文摘要