Evaluating Temporal Bias in Time Series Event Detection Methods.
J. Inf. Data Manag.(2021)
摘要
The detection of events in time series is an important task in several areas of knowledge where operations monitoring is essential. Experts often have to deal with choosing the most appropriate event detection method for a time series, which can be a complex task. There is a demand for benchmarking different methods in order to guide this choice. For this, standard classification accuracy metrics are usually adopted. However, they are insufficient for a qualitative analysis of the tendency of a method to precede or delay event detections. Such analysis is interesting for applications in which tolerance for "close" detections is important rather than focusing only on accurate ones. In this context, this paper proposes a more comprehensive event detection benchmark process, including an analysis of temporal bias of detection methods. For that, metrics based on the time distance between event detections and identified events (detection delay) are adopted. Computational experiments were conducted using real-world and synthetic datasets from Yahoo Labs and resources from the Harbinger framework for event detection. Adopting the proposed detection delay-based metrics helped obtain a complete overview of the performance and general behavior of detection methods.
更多查看译文
关键词
temporal bias,event,detection,time
AI 理解论文
溯源树
样例
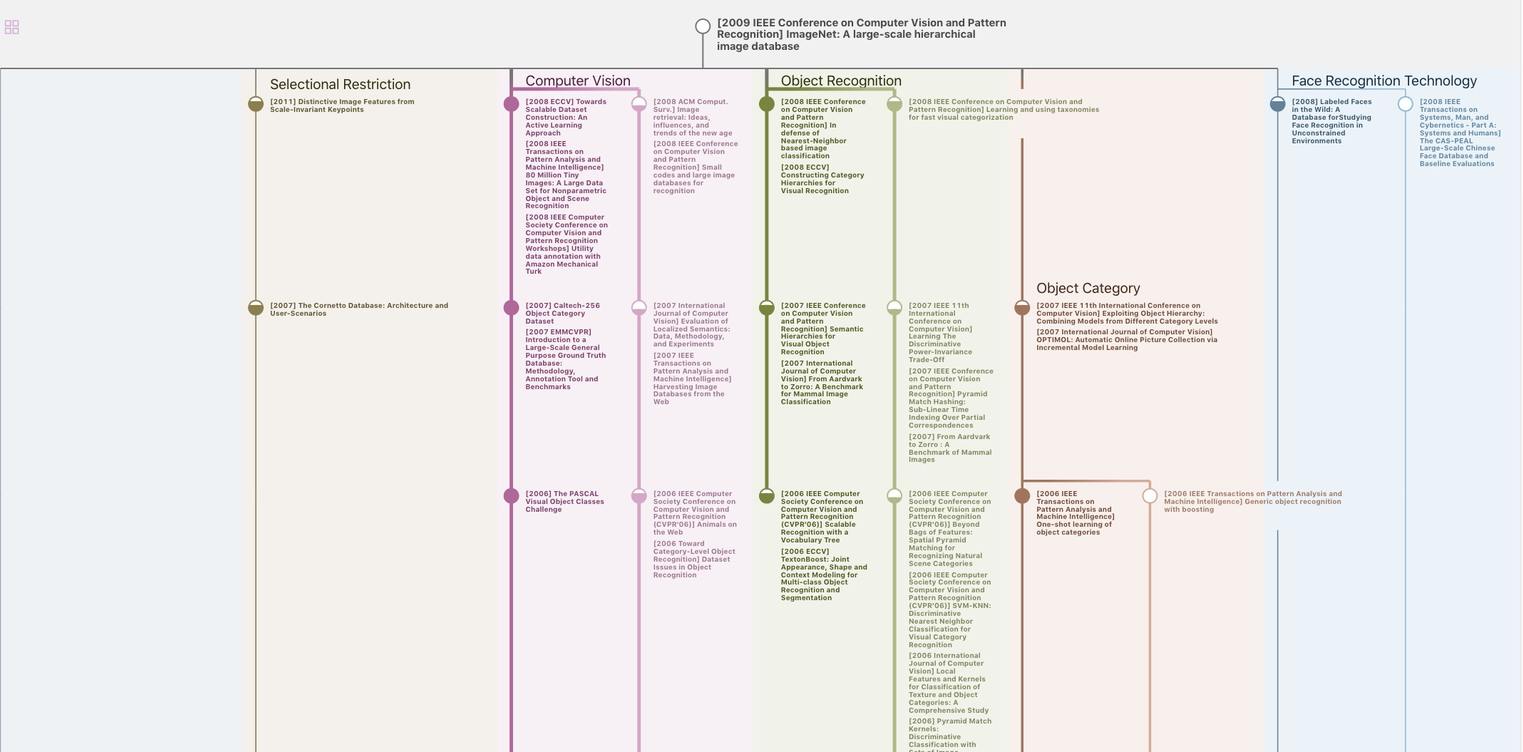
生成溯源树,研究论文发展脉络
Chat Paper
正在生成论文摘要