Autoencoder-based feature construction for IoT attacks clustering
Future Generation Computer Systems(2022)
Abstract
Variations in commands executed as part of the attack process can be used to determine the behavioural patterns of IoT attacks. Existing approaches rely on the domain knowledge of security experts to identify the behavioural patterns, categorise and classify cyber attacks. We proposed an Autoencoder (AE)-based feature construction approach to remove the dependency of manually correlating commands and generate an efficient representation by automatically learning the semantic similarity between input features extracted through commands data. We applied three clustering algorithms, i.e., K-means, Gaussian Mixture Models and Density-based spatial clustering of applications with noise, on our data set of AE features. We discussed the clustering arrangements for understanding the impact of changes in commands on behavioural patterns of attacks and how attacks are grouped in the same or different clusters. Evaluation of our feature construction approach shows that the clustering algorithm grouped attacks with more common features values compared to clustering with original features. Moreover, we performed a comparative analysis of two existing feature extraction approaches on our data set considering the type of analysis in the process, generalisability of applying features, coverage to the data set and clustering arrangements. We found that challenges identified in applying existing approaches can be addressed with our proposed approach and improving features with AE resulted in providing meaningful clustering interpretations.
MoreTranslated text
Key words
IoT attacks,Feature construction,Autoencoder,Clustering
AI Read Science
Must-Reading Tree
Example
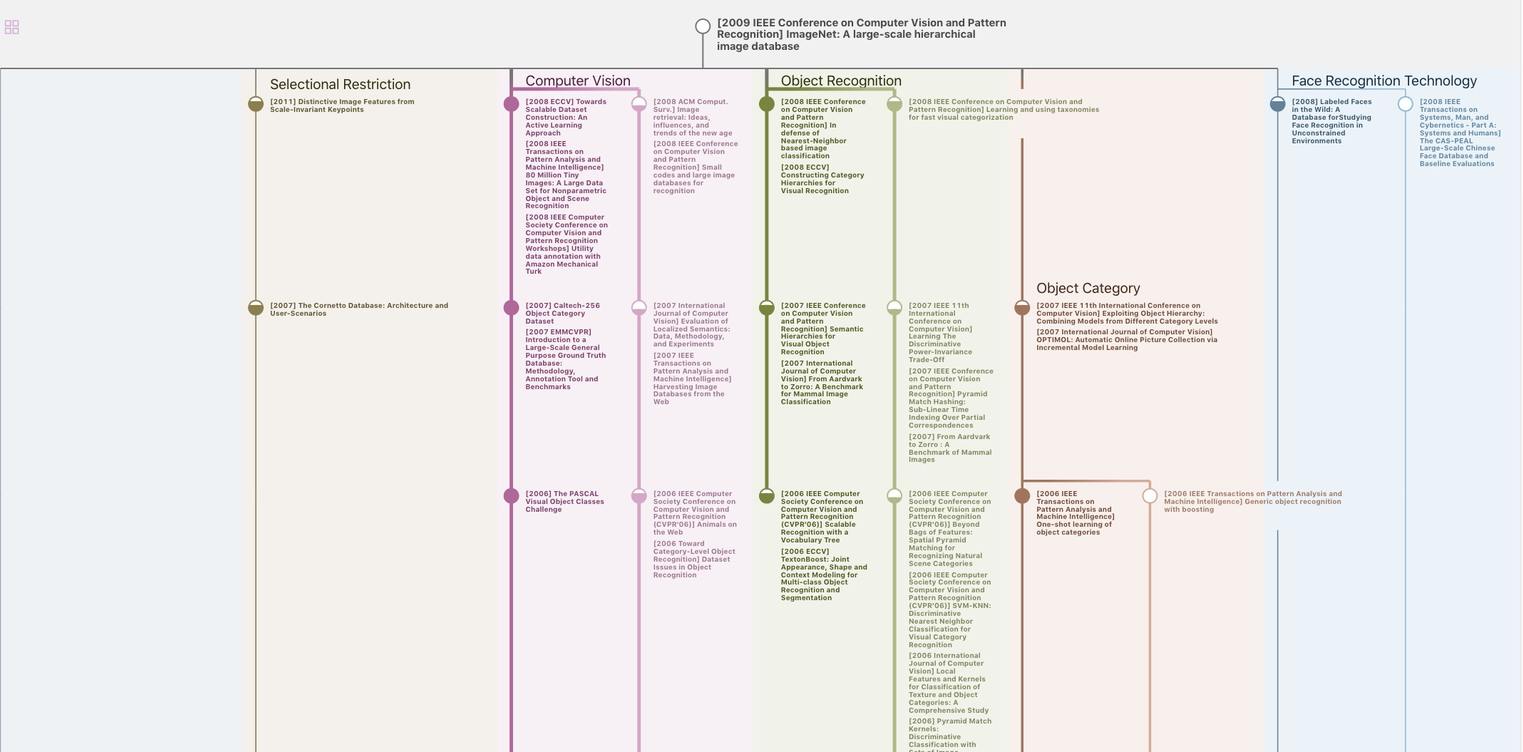
Generate MRT to find the research sequence of this paper
Chat Paper
Summary is being generated by the instructions you defined