A Quantum Annealing Approach to Reduce Covid-19 Spread on College Campuses
arXiv (Cornell University)(2021)
摘要
Disruptions of university campuses caused by COVID-19 have motivated strategies to prevent the spread of infectious diseases while maintaining some level of in person learning. In response, the proposed approach recursively applied a quantum annealing algorithm for Max-Cut optimization on D-Wave Systems, which grouped students into cohorts such that the number of possible infection events via shared classrooms was minimized. To test this approach, available coursework data was used to generate highly clustered course enrollment networks representing students and the classes they share. The algorithm was then recursively called on these networks to group students, and a disease model was applied to forecast disease spread. Simulation results showed that under some assumptions on disease statistics and methods of spread, the quantum grouping method reduced both the total and peak percentage of infected students when compared against random groupings of students. Scaling to larger networks, it is possible that this quantum annealer-assisted grouping approach may provide practical advantage over classical approaches. This paper, however, is strictly a proof-of-concept demonstration of the approach and is not intended to argue for a quantum speedup.
更多查看译文
关键词
quantum annealing approach,campuses
AI 理解论文
溯源树
样例
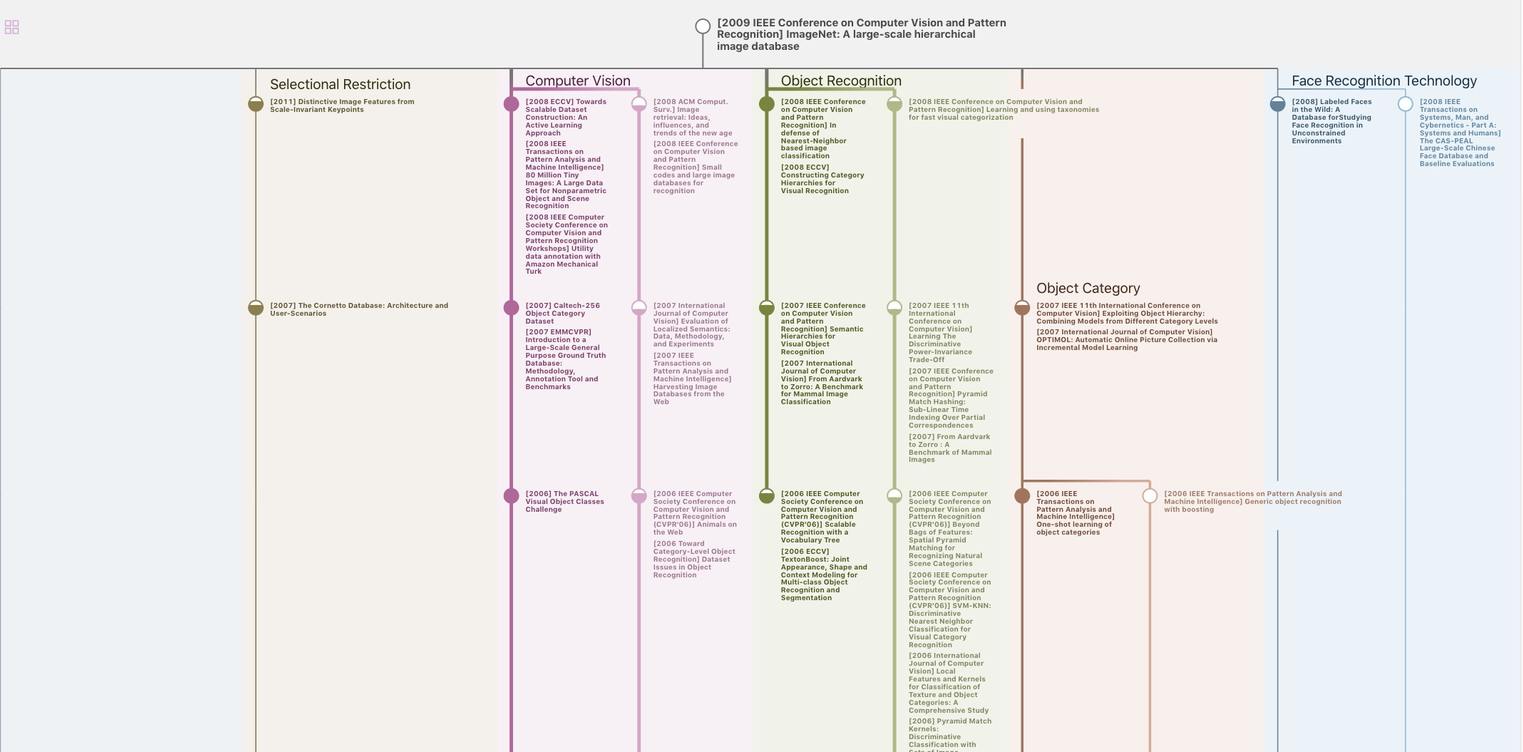
生成溯源树,研究论文发展脉络
Chat Paper
正在生成论文摘要