Trap of Feature Diversity in the Learning of MLPs
ArXiv(2021)
摘要
In this paper, we discover a two-phase phenomenon in the learning of multi-layer perceptrons (MLPs). I.e., in the first phase, the training loss does not decrease significantly, but the similarity of features between different samples keeps increasing, which hurts the feature diversity. We explain such a two-phase phenomenon in terms of the learning dynamics of the MLP. Furthermore, we propose two normalization operations to eliminate the two-phase phenomenon, which avoids the decrease of the feature diversity and speeds up the training process.
更多查看译文
关键词
feature diversity,mlps,learning
AI 理解论文
溯源树
样例
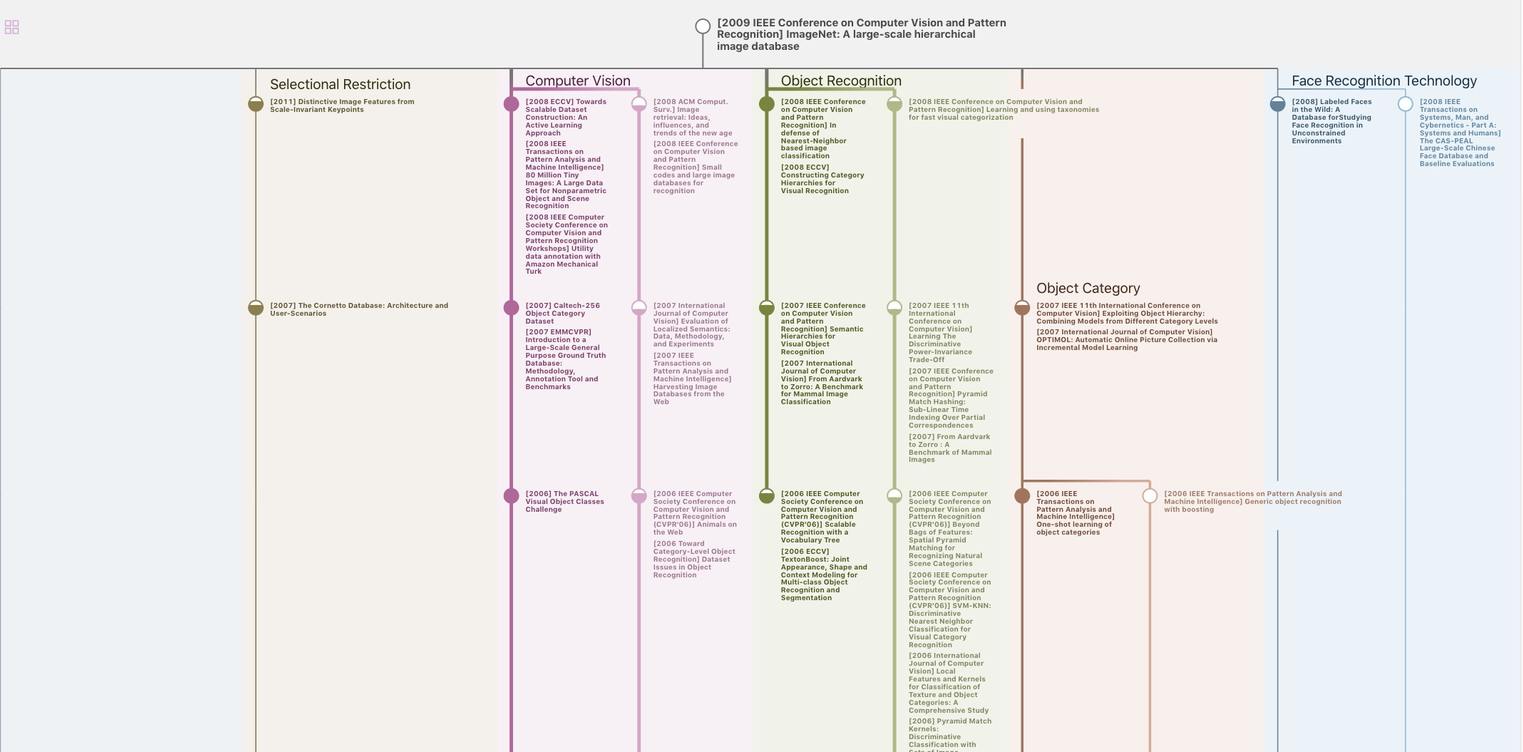
生成溯源树,研究论文发展脉络
Chat Paper
正在生成论文摘要