IMRecoNet: Learn to Detect in Index Modulation Aided MIMO Systems With Complex Valued Neural Networks
IEEE Transactions on Vehicular Technology(2023)
摘要
Index modulation (IM) reduces the power consumption and hardware cost of the multiple-input multiple-output (MIMO) system by activating part of the antennas for data transmission. However, IM significantly increases the complexity of the receiver and needs accurate channel estimation to guarantee its performance. To tackle these challenges, in this paper, we design a deep learning (DL) based detector for the IM aided MIMO (IM-MIMO) systems. We first formulate the detection process as a sparse reconstruction problem by utilizing the inherent attributes of IM. Then, based on greedy strategy, we design a DL based detector, called IMRecoNet, to realize this sparse reconstruction process. Different from the general neural networks, we introduce complex value operations to adapt the complex signals in communication systems. To the best of our knowledge, this is the first attempt that introduce complex valued neural network to the design of detector for the IM-MIMO systems. Finally, to verify the adaptability and robustness of the proposed detector, simulations are carried out with consideration of inaccurate channel state information (CSI) and correlated MIMO channels. The simulation results demonstrate that the proposed detector outperforms existing algorithms in terms of antenna recognition accuracy and bit error rate under various scenarios.
更多查看译文
关键词
Complex-valued neural network,index modu-lation,multiple-input multiple-output,signal detection,sparse reconstruction
AI 理解论文
溯源树
样例
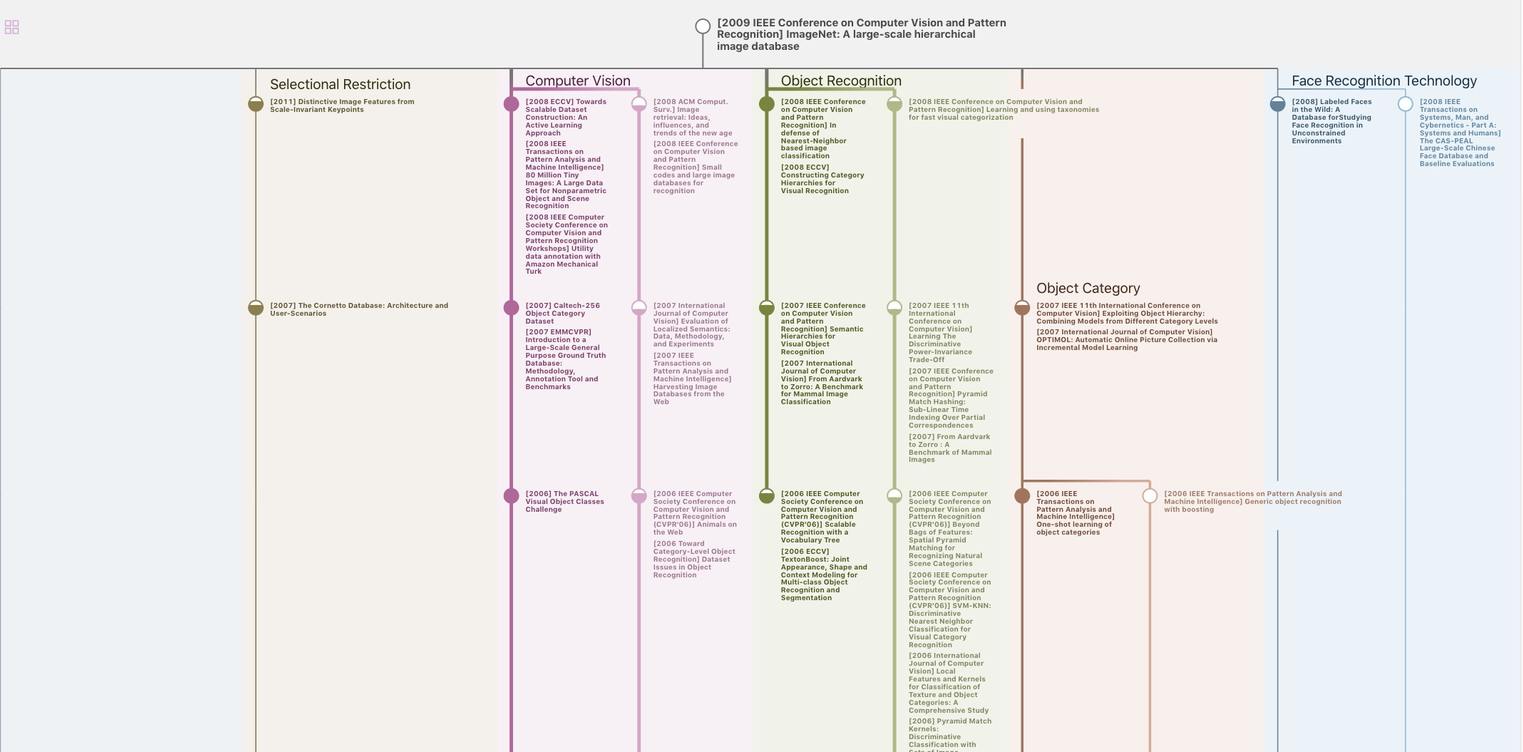
生成溯源树,研究论文发展脉络
Chat Paper
正在生成论文摘要