Interpreting Deep Machine Learning Models: An Easy Guide for Oncologists
IEEE Reviews in Biomedical Engineering(2023)
摘要
Healthcare agents, in particular in the oncology field, are currently collecting vast amounts of diverse patient data. In this context, some decision-support systems, mostly based on deep learning techniques, have already been approved for clinical purposes. Despite all the efforts in introducing artificial intelligence methods in the workflow of clinicians, its lack of interpretability - understand how the methods make decisions - still inhibits their dissemination in clinical practice. The aim of this article is to present an easy guide for oncologists explaining how these methods make decisions and illustrating the strategies to explain them. Theoretical concepts were illustrated based on oncological examples and a literature review of research works was performed from PubMed between January 2014 to September 2020, using “deep learning techniques,” “interpretability” and “oncology” as keywords. Overall, more than 60% are related to breast, skin or brain cancers and the majority focused on explaining the importance of tumor characteristics (e.g. dimension, shape) in the predictions. The most used computational methods are multilayer perceptrons and convolutional neural networks. Nevertheless, despite being successfully applied in different cancers scenarios, endowing deep learning techniques with interpretability, while maintaining their performance, continues to be one of the greatest challenges of artificial intelligence.
更多查看译文
关键词
Big Data,interpretability,deep learning,decision-support systems,oncology
AI 理解论文
溯源树
样例
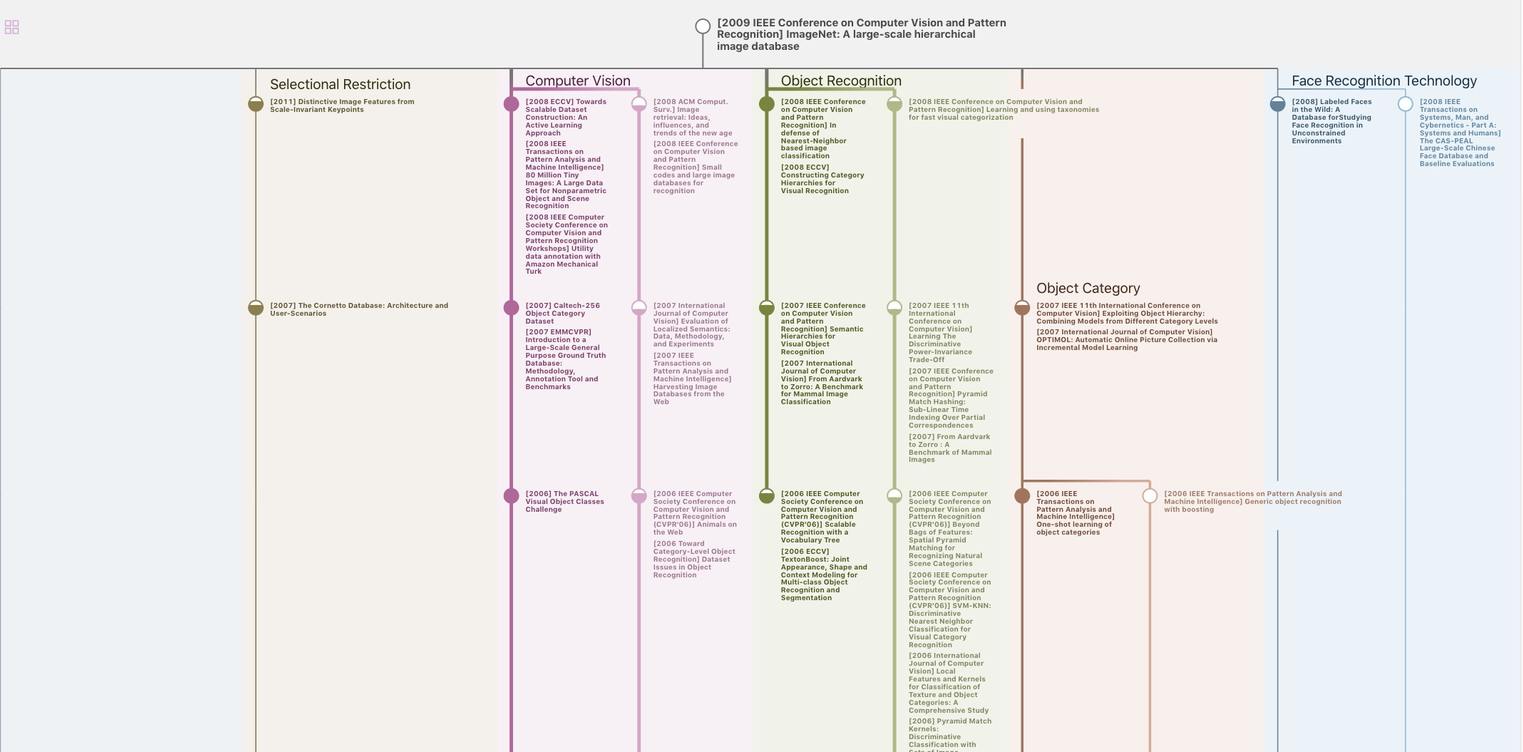
生成溯源树,研究论文发展脉络
Chat Paper
正在生成论文摘要