A data-driven framework to identify human-critical autonomous vehicle testing and deployment zones
GIS(2021)
摘要
ABSTRACTWe proposed a data-driven framework that leverages machine learning and econometric modeling techniques to investigate autonomous vehicle (AV) involved crashes and maps human-critical safety factors to operational design domains. The goal is to assist the infrastructure owner-operators in identifying human-critical AV-testing-and-deployment zones based on data-driven insights from both AV-testing data (e.g., California Department of Motor Vehicle AV crash reports) and historical crash data involving only human drivers. First, we analyzed AV crash data collected from the CA DMV website for May 2018 to December 2020 using ML-based and econometric models incorporating attributes such as weather, lighting condition, road surface condition, vehicle miles traveled, and collision type. Later we use the findings to demonstrate the framework's applicability for New York City crash data at the Zip Code level (2012--2021).
更多查看译文
AI 理解论文
溯源树
样例
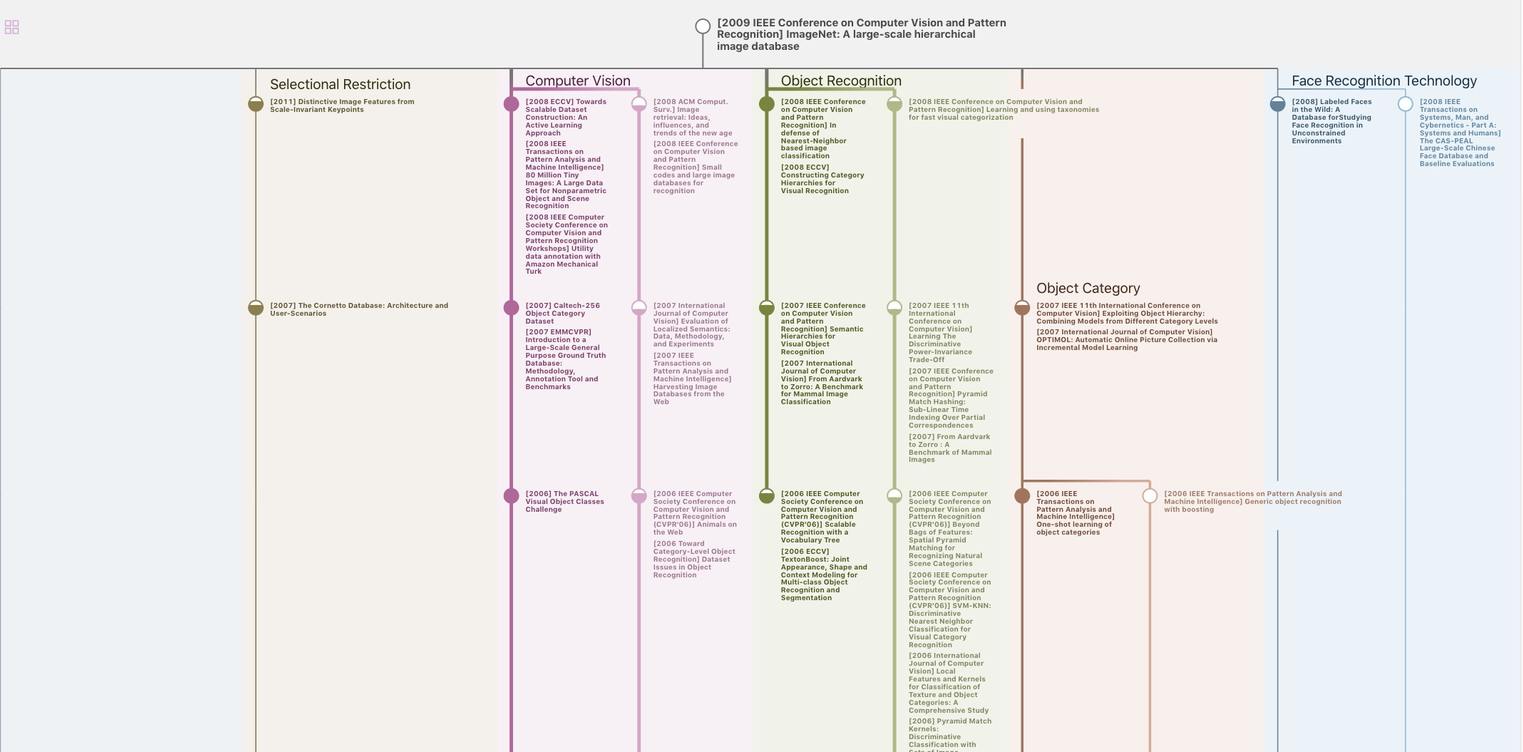
生成溯源树,研究论文发展脉络
Chat Paper
正在生成论文摘要