Morphological Cross Entropy Loss for Improved Semantic Segmentation of Small and Thin Objects
KNOWLEDGE-BASED AND INTELLIGENT INFORMATION & ENGINEERING SYSTEMS (KSE 2021)(2021)
摘要
Image segmentation often faces a trade-off between using higher resolution images to detect fine details, such as the edges of objects or thin structures, and lower resolution images which is more suitable for accurate segmentation of massive objects. Because low resolution images require less resources, accurate detection of small objects is often less prioritized in trying to achieve the highest accuracy. In this paper, we propose to improve the segmentation of small and thin objects by convolutional neural networks by adding a morphological element to the loss function used for training the segmentation network. The approach is tested on a traffic sign segmentation problem using the Cityscapes dataset with a training set of 2 979 images and is shown to have an advantage over the popular cross-entropy (CE) and a ground truth (GT) affinity map weighted CE as it yields higher global IoU and, more importantly, an IoU gain among the smaller traffic signs with no additional computational resources. (C) 2021 The Authors. Published by Elsevier B.V. This is an open access article under the CC BY-NC-ND license (https://crativecommons.org/licenses/by-nc-nd/4.0) Peer-review under responsibility of the scientific committee of KES International.
更多查看译文
关键词
Semantic segmentation, Traffic signs, Loss function, Convolutional neural network
AI 理解论文
溯源树
样例
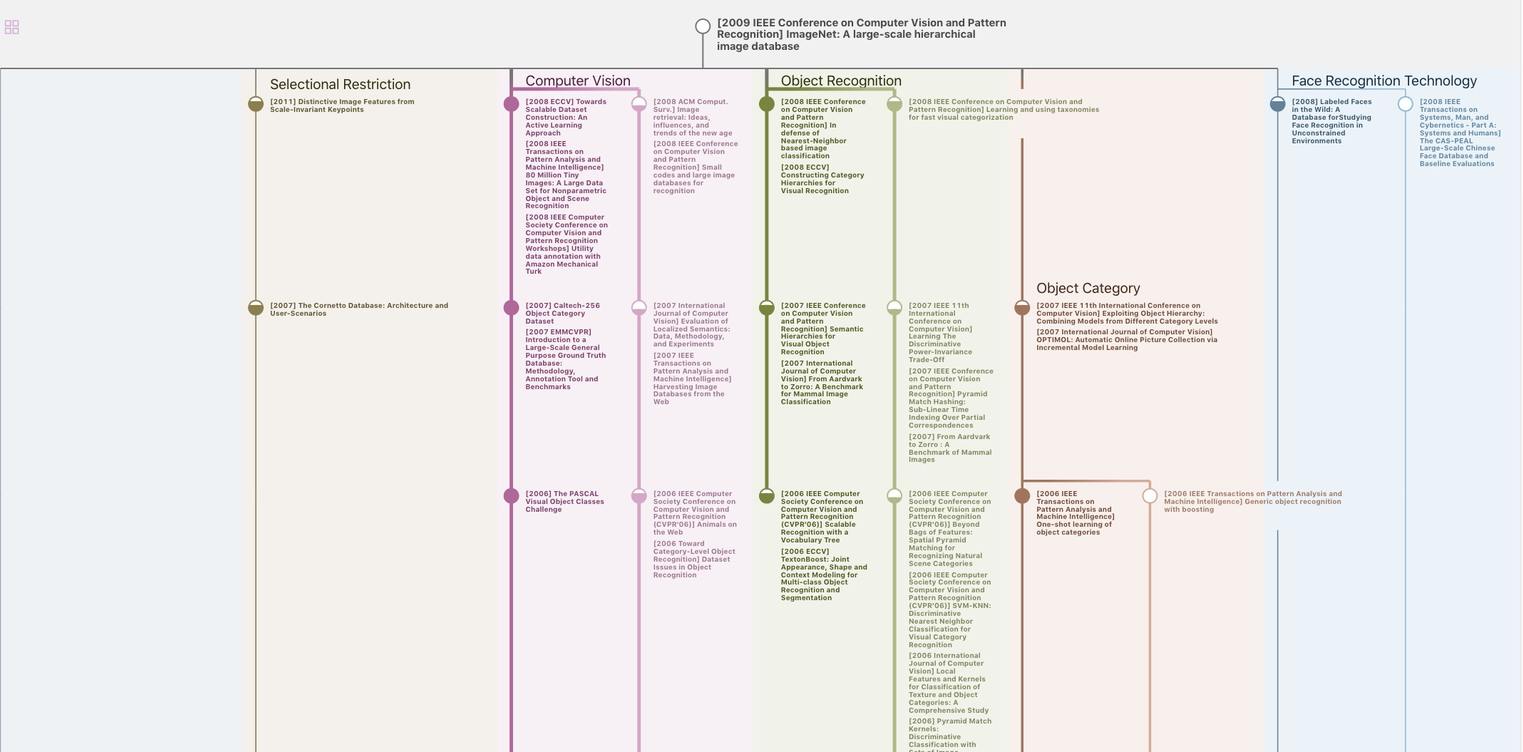
生成溯源树,研究论文发展脉络
Chat Paper
正在生成论文摘要