Bayesian calibration of a stochastic, multiscale agent-based model for predicting in vitro tumor growth
PLOS COMPUTATIONAL BIOLOGY(2021)
摘要
Hybrid multiscale agent-based models (ABMs) are unique in their ability to simulate individual cell interactions and microenvironmental dynamics. Unfortunately, the high computational cost of modeling individual cells, the inherent stochasticity of cell dynamics, and numerous model parameters are fundamental limitations of applying such models to predict tumor dynamics. To overcome these challenges, we have developed a coarse-grained two-scale ABM (cgABM) with a reduced parameter space that allows for an accurate and efficient calibration using a set of time-resolved microscopy measurements of cancer cells grown with different initial conditions. The multiscale model consists of a reaction-diffusion type model capturing the spatio-temporal evolution of glucose and growth factors in the tumor microenvironment (at tissue scale), coupled with a lattice-free ABM to simulate individual cell dynamics (at cellular scale). The experimental data consists of BT474 human breast carcinoma cells initialized with different glucose concentrations and tumor cell confluences. The confluence of live and dead cells was measured every three hours over four days. Given this model, we perform a time-dependent global sensitivity analysis to identify the relative importance of the model parameters. The subsequent cgABM is calibrated within a Bayesian framework to the experimental data to estimate model parameters, which are then used to predict the temporal evolution of the living and dead cell populations. To this end, a moment-based Bayesian inference is proposed to account for the stochasticity of the cgABM while quantifying uncertainties due to limited temporal observational data. The cgABM reduces the computational time of ABM simulations by 93% to 97% while staying within a 3% difference in prediction compared to ABM. Additionally, the cgABM can reliably predict the temporal evolution of breast cancer cells observed by the microscopy data with an average error and standard deviation for live and dead cells being 7.61 +/- 2.01 and 5.78 +/- 1.13, respectively. Author summaryThe calibration of agent-based models of tumor cell growth to experimental data remains a challenge in computational oncology. Besides the computational cost of modeling thousands of agents, the model's intrinsic stochasticity demands numerous realizations of the simulations to accurately represent the statistical features of the model predictions. We developed a hybrid, multiscale, coarse-grain, agent-based model that captures the growth and decline of human breast carcinoma cells under different initial conditions. We determined the effects of coarse-graining the ABM on the multiscale model output and the number of repetitions necessary to capture the stochastic transitions present in the model. We identified the most influential parameters on the model prediction through a sensitivity analysis and selected which parameters can be fixed and which ones should be calibrated. Using Bayesian calibration, we show that the model can accurately represent the experimental data. The validation step indicates that our model can reliably predict the in vitro temporal data, depending on the choice of the training (calibration data) sets.
更多查看译文
关键词
bayesian calibration,tumor growth,stochastic,agent-based
AI 理解论文
溯源树
样例
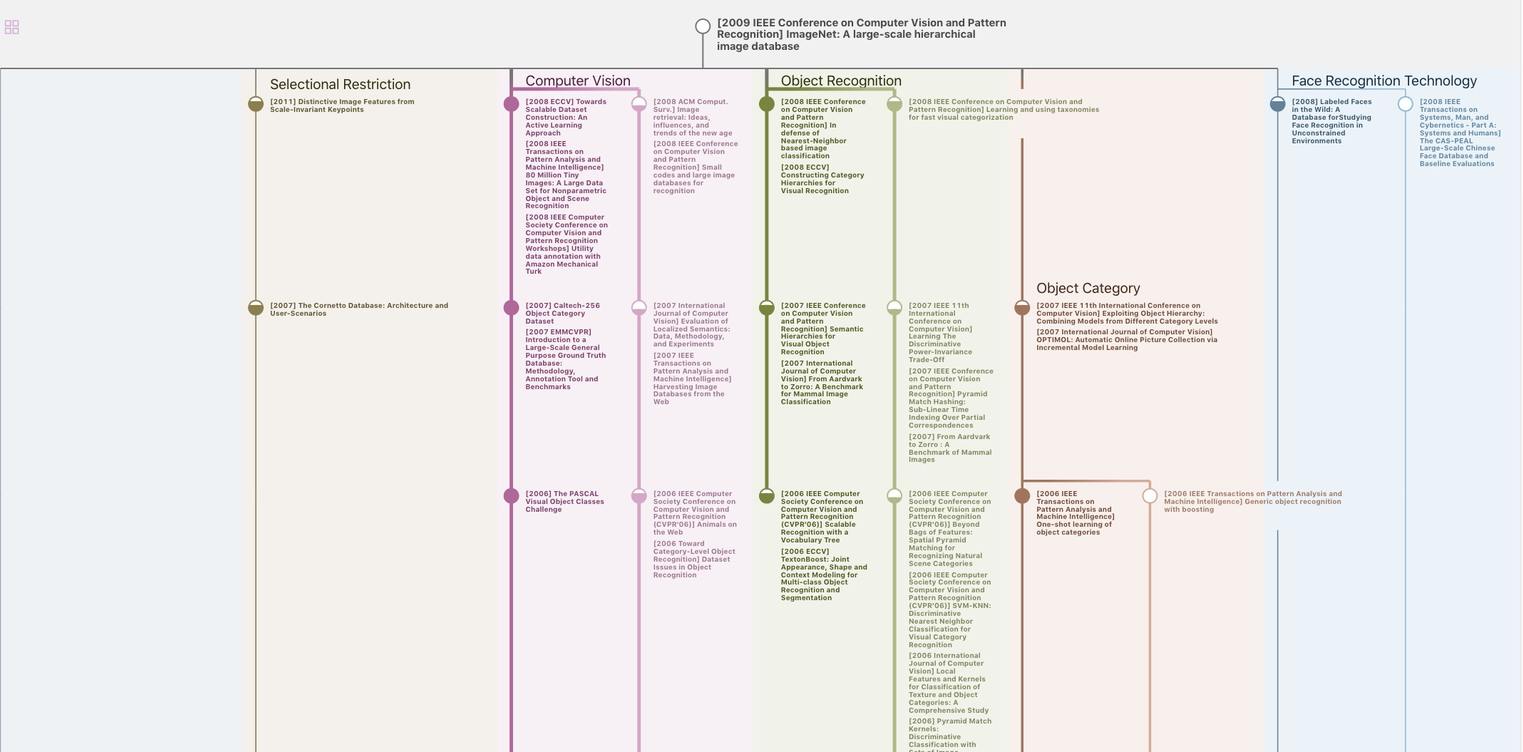
生成溯源树,研究论文发展脉络
Chat Paper
正在生成论文摘要