Structured connectivity in the cerebellum enables noise-resilient pattern separation
Nature(2021)
摘要
The cerebellum is thought to detect and correct errors between intended and executed commands[1][1]–[3][2] and is critical for social behaviors, cognition and emotion[4][3]–[6][4]. Computations for motor control must be performed quickly to correct errors in real time and should be sensitive to small differences between patterns for fine error correction while being resilient to noise[7][5]. Influential theories of cerebellar information processing have largely assumed random network connectivity, which increases the encoding capacity of the network’s first layer[8][6]–[13][7]. However, maximizing encoding capacity reduces resiliency to noise[7][5]. To understand how neuronal circuits address this fundamental tradeoff, we mapped the feedforward connectivity in the mouse cerebellar cortex using automated large-scale transmission electron microscopy (EM) and convolutional neural network-based image segmentation. We found that both the input and output layers of the circuit exhibit redundant and selective connectivity motifs, which contrast with prevailing models. Numerical simulations suggest these redundant, non-random connectivity motifs increase discriminability of similar input patterns at a minimal cost to the network’s overall encoding capacity. This work reveals how neuronal network structure can balance encoding capacity and redundancy, unveiling principles of biological network architecture with implications for artificial neural network design.
### Competing Interest Statement
W.C.A.L. and D.G.C.H. declare the following competing interest: Harvard University filed a patent application regarding GridTape (WO2017184621A1) on behalf of the inventors including W.C.A.L and D.G.C.H., and negotiated licensing agreements with interested partners.
[1]: #ref-1
[2]: #ref-3
[3]: #ref-4
[4]: #ref-6
[5]: #ref-7
[6]: #ref-8
[7]: #ref-13
更多查看译文
AI 理解论文
溯源树
样例
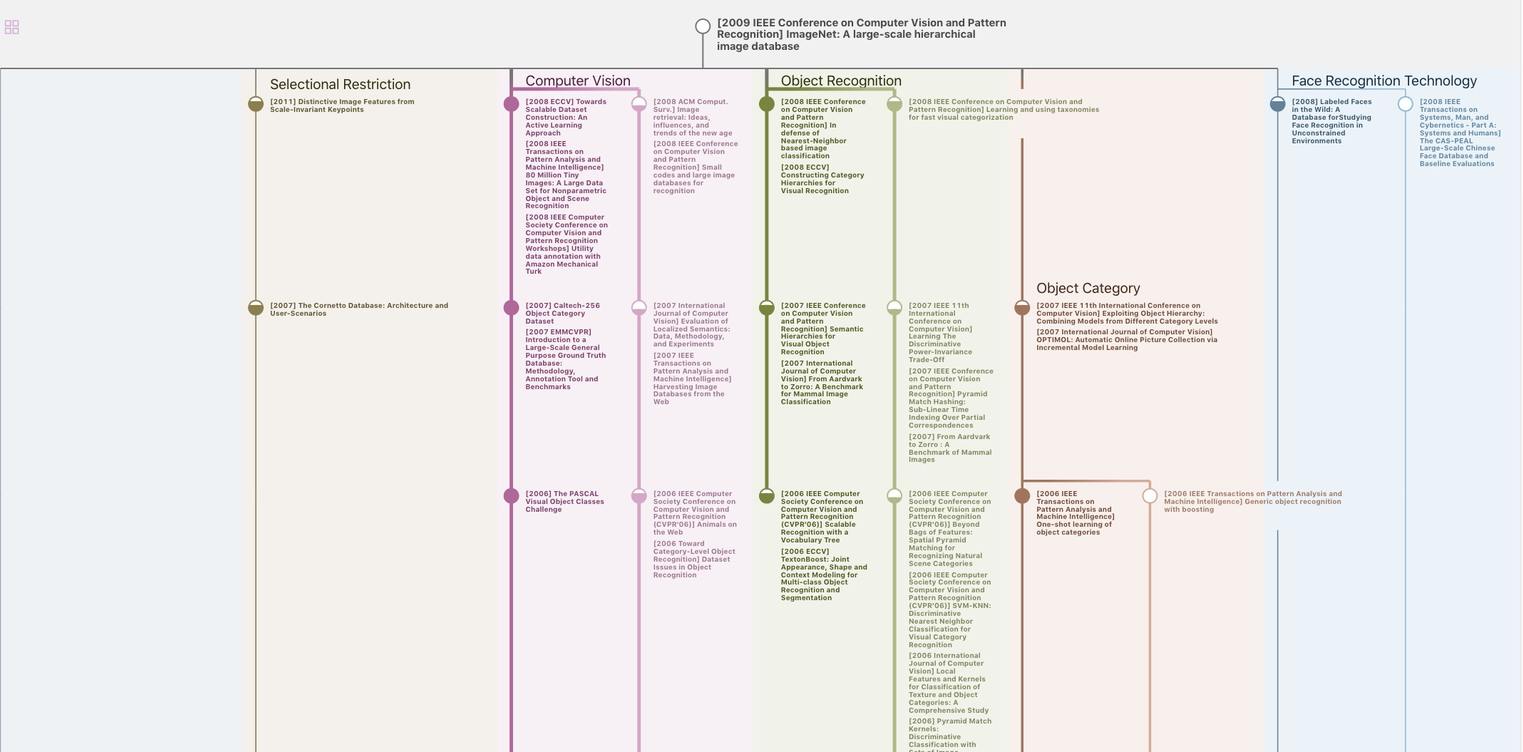
生成溯源树,研究论文发展脉络
Chat Paper
正在生成论文摘要