Aerial Images Meet Crowdsourced Trajectories: A New Approach to Robust Road Extraction.
IEEE transactions on neural networks and learning systems(2022)
摘要
Land remote-sensing analysis is a crucial research in earth science. In this work, we focus on a challenging task of land analysis, i.e., automatic extraction of traffic roads from remote-sensing data, which has widespread applications in urban development and expansion estimation. Nevertheless, conventional methods either only utilized the limited information of aerial images, or simply fused multimodal information (e.g., vehicle trajectories), thus cannot well recognize unconstrained roads. To facilitate this problem, we introduce a novel neural network framework termed cross-modal message propagation network (CMMPNet), which fully benefits the complementary different modal data (i.e., aerial images and crowdsourced trajectories). Specifically, CMMPNet is composed of two deep autoencoders for modality-specific representation learning and a tailor-designed dual enhancement module for cross-modal representation refinement. In particular, the complementary information of each modality is comprehensively extracted and dynamically propagated to enhance the representation of another modality. Extensive experiments on three real-world benchmarks demonstrate the effectiveness of our CMMPNet for robust road extraction benefiting from blending different modal data, either using image and trajectory data or image and light detection and ranging (LiDAR) data. From the experimental results, we observe that the proposed approach outperforms current state-of-the-art methods by large margins. Our source code is resealed on the project page http://lingboliu.com/multimodal_road_extraction.html.
更多查看译文
关键词
Roads,Trajectory,Feature extraction,Data mining,Remote sensing,Geoscience,Visualization,Aerial images,crowdsourced trajectories,land remote sensing,road network extraction
AI 理解论文
溯源树
样例
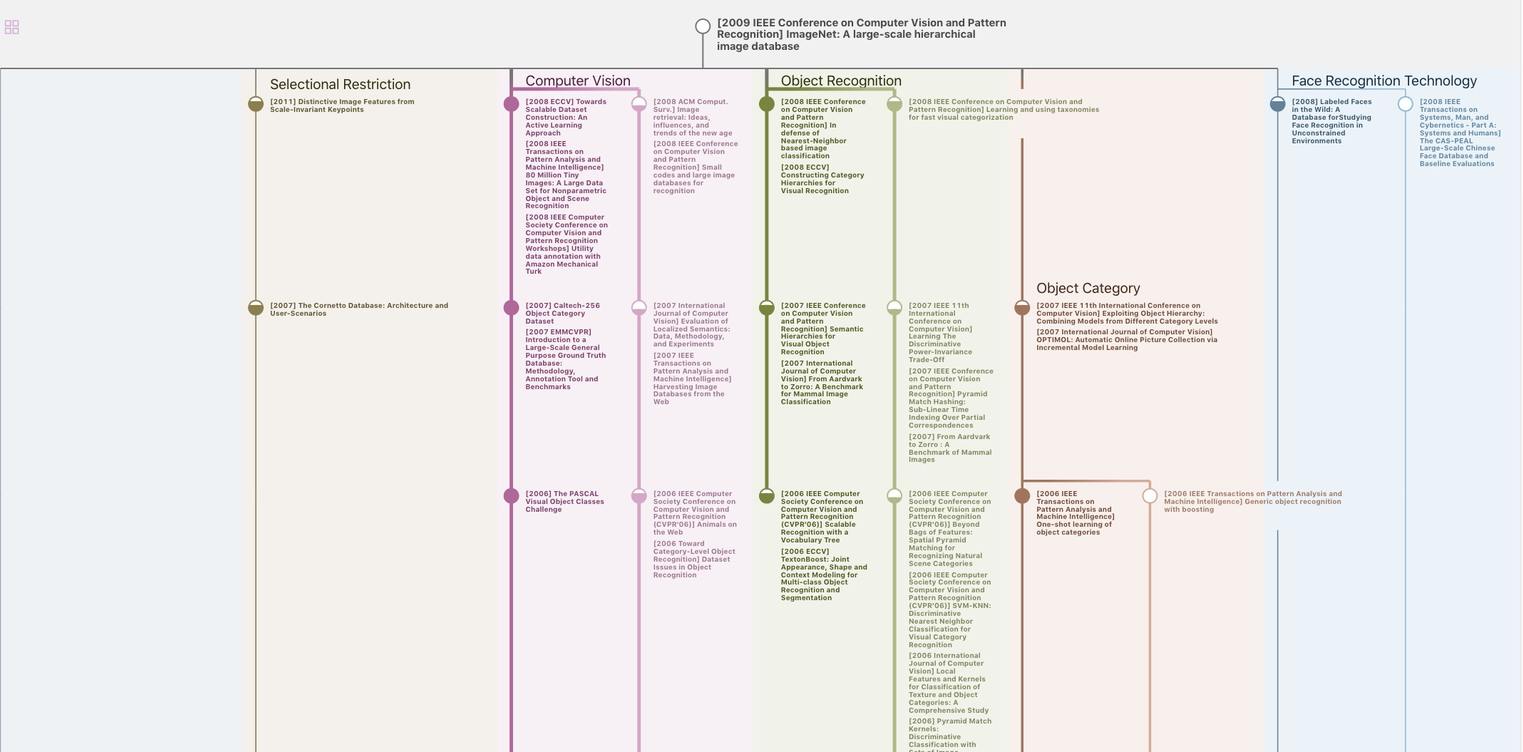
生成溯源树,研究论文发展脉络
Chat Paper
正在生成论文摘要