Blockchain for federated learning toward secure distributed machine learning systems: a systemic survey
Soft Computing(2021)
摘要
Federated learning ( FL ) is a promising decentralized deep learning technology, which allows users to update models cooperatively without sharing their data. FL is reshaping existing industry paradigms for mathematical modeling and analysis, enabling an increasing number of industries to build privacy-preserving, secure distributed machine learning models. However, the inherent characteristics of FL have led to problems such as privacy protection, communication cost, systems heterogeneity, and unreliability model upload in actual operation. Interestingly, the integration with Blockchain technology provides an opportunity to further improve the FL security and performance, besides increasing its scope of applications. Therefore, we denote this integration of Blockchain and FL as the Blockchain-based federated learning ( BCFL ) framework. This paper introduces an in-depth survey of BCFL and discusses the insights of such a new paradigm. In particular, we first briefly introduce the FL technology and discuss the challenges faced by such technology. Then, we summarize the Blockchain ecosystem. Next, we highlight the structural design and platform of BCFL . Furthermore, we present the attempts ins improving FL performance with Blockchain and several combined applications of incentive mechanisms in FL . Finally, we summarize the industrial application scenarios of BCFL .
更多查看译文
关键词
Blockchain,Federated learning,Smart Contract,Incentive mechanism,Industrial Applications
AI 理解论文
溯源树
样例
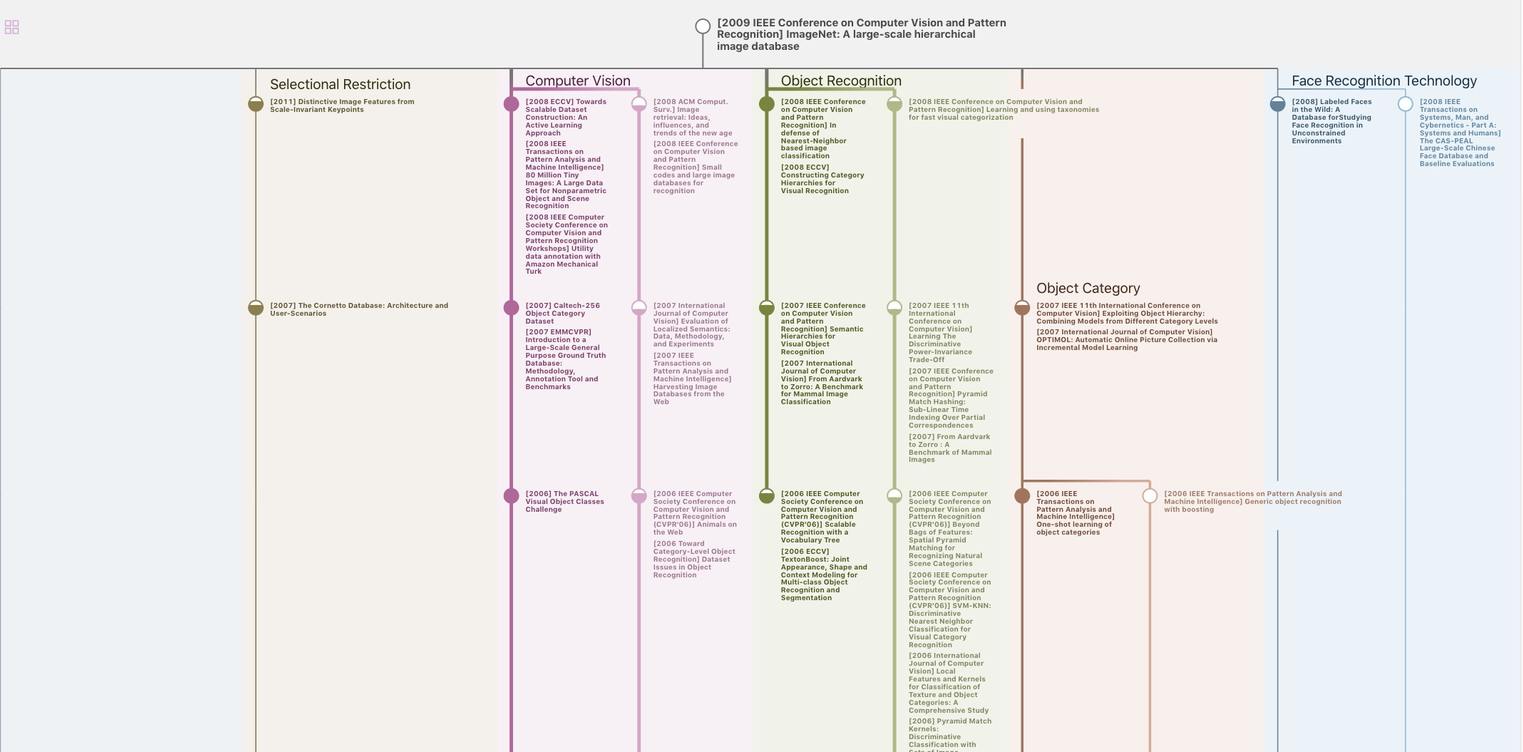
生成溯源树,研究论文发展脉络
Chat Paper
正在生成论文摘要