An efficient interactive multi-label segmentation tool for 2D and 3D medical images using fully connected conditional random field
COMPUTER METHODS AND PROGRAMS IN BIOMEDICINE(2022)
摘要
Objective: Image segmentation is a crucial and fundamental step in many medical image analysis tasks, such as tumor measurement, surgery planning, disease diagnosis, etc. To ensure the quality of image segmentation, most of the current solutions require labor-intensive manual processes by tracing the boundaries of the objects. The workload increases tremendously for the case of three dimensional (3D) image with multiple objects to be segmented. Method: In this paper, we introduce our developed interactive image segmentation tool that provides efficient segmentation of multiple labels for both 2D and 3D medical images. The core segmentation method is based on a fast implementation of the fully connected conditional random field. The software also enables automatic recommendation of the next slice to be annotated in 3D, leading to a higher efficiency. Results: We have evaluated the tool on many 2D and 3D medical image modalities (e.g. CT, MRI, ultrasound, X-ray, etc.) and different objects of interest (abdominal organs, tumor, bones, etc.), in terms of segmentation accuracy, repeatability and computational time. Conclusion: In contrast to other interactive image segmentation tools, our software produces high quality image segmentation results without the requirement of parameter tuning for each application. Both the software and source code are freely available for research purpose(1. 1)Software and source code download: https://drive.google.com/file/d/1JIzWkT3M-X7jeB8tTwVcEw240TGbJAvj/view?usp=sharing. (C) 2021 Elsevier B.V. Allrights reserved.
更多查看译文
关键词
2D&3D Medical image segmentation, Conditional random filed
AI 理解论文
溯源树
样例
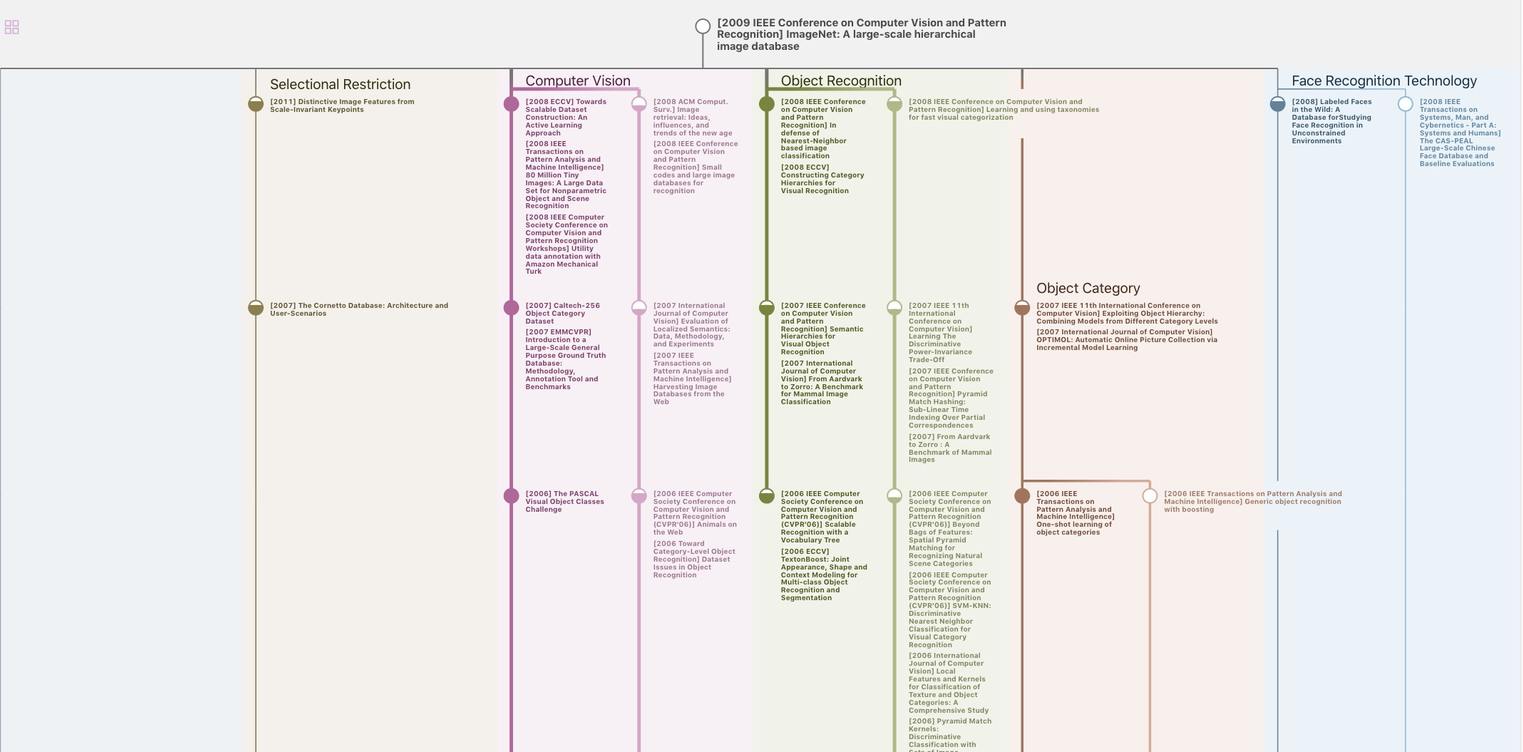
生成溯源树,研究论文发展脉络
Chat Paper
正在生成论文摘要