An adversarial discriminative temporal convolutional network for EEG-based cross-domain emotion recognition.
Computers in biology and medicine(2021)
摘要
Domain adaptation (DA) tackles the problem where data from the source domain and target domain have different underlying distributions. In cross-domain (cross-subject or cross-dataset) emotion recognition based on EEG signals, traditional classification methods lack domain adaptation capabilities and have low performance. To address this problem, we proposed a novel domain adaptation strategy called adversarial discriminative-temporal convolutional networks (AD-TCNs) in this study, which can ensure the invariance of the representation of feature graphs in different domains and fill in the differences between different domains. For EEG data with specific temporal attributes, the temporal model TCN is used as the feature encoder. In the cross-subject experiment, our AD-TCN method achieved the highest accuracies of the valence and arousal dimensions in both the DREAMER and DEAP datasets. In the cross-dataset experiment, two of the eight task groups showed accuracies of 62.65% and 62.36%. Compared with the state-of-the-art performance in the same protocol, experimental results demonstrated that our method is an effective extension to realize EEG-based cross-domain emotion recognition.
更多查看译文
AI 理解论文
溯源树
样例
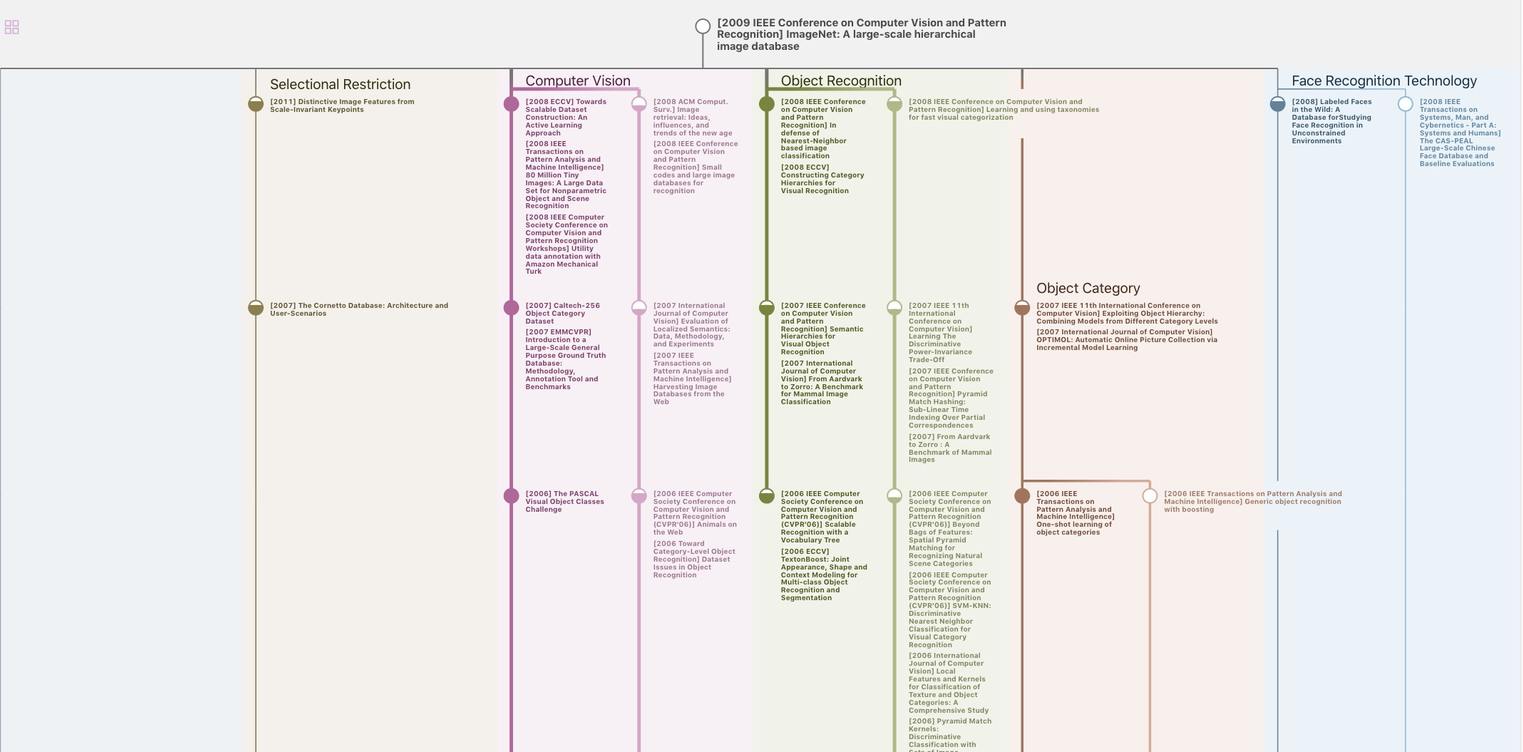
生成溯源树,研究论文发展脉络
Chat Paper
正在生成论文摘要