Rank-Constrained Least-Squares: Prediction and Inference
arXiv (Cornell University)(2021)
摘要
In this work, we focus on the high-dimensional trace regression model with a low-rank coefficient matrix. We establish a nearly optimal in-sample prediction risk bound for the rank-constrained least-squares estimator under no assumptions on the design matrix. Lying at the heart of the proof is a covering number bound for the family of projection operators corresponding to the subspaces spanned by the design. By leveraging this complexity result, we perform a power analysis for a permutation test on the existence of a low-rank signal under the high-dimensional trace regression model. Finally, we use alternating minimization to approximately solve the rank-constrained least-squares problem to evaluate its empirical in-sample prediction risk and power of the resulting permutation test in our numerical study.
更多查看译文
关键词
prediction,inference,rank-constrained,least-squares
AI 理解论文
溯源树
样例
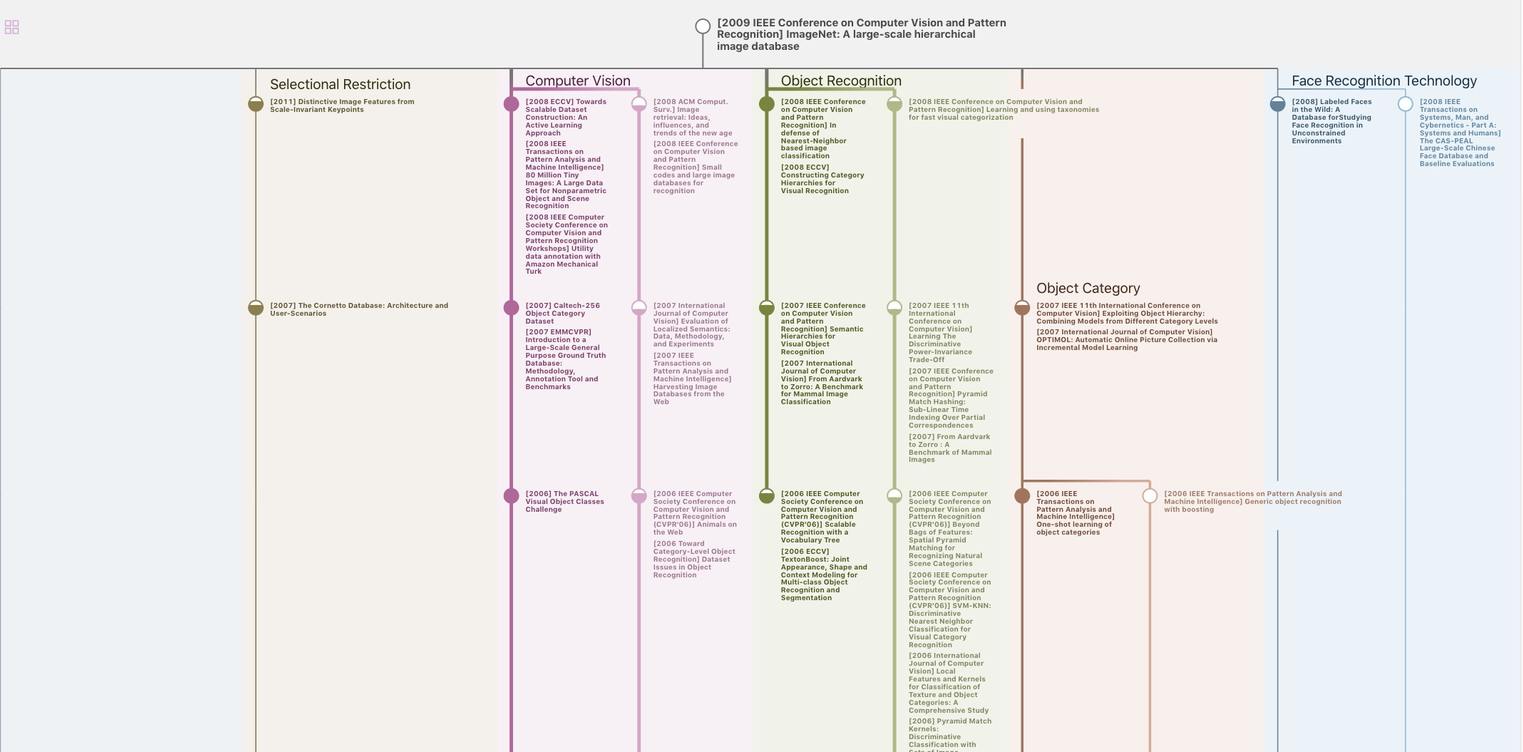
生成溯源树,研究论文发展脉络
Chat Paper
正在生成论文摘要