Exploring Low-Cost Transformer Model Compression for Large-Scale Commercial Reply Suggestions
CoRR(2021)
Abstract
Fine-tuning pre-trained language models improves the quality of commercial reply suggestion systems, but at the cost of unsustainable training times. Popular training time reduction approaches are resource intensive, thus we explore low-cost model compression techniques like Layer Dropping and Layer Freezing. We demonstrate the efficacy of these techniques in large-data scenarios, enabling the training time reduction for a commercial email reply suggestion system by 42%, without affecting the model relevance or user engagement. We further study the robustness of these techniques to pre-trained model and dataset size ablation, and share several insights and recommendations for commercial applications.
MoreTranslated text
Key words
low-cost,large-scale
AI Read Science
Must-Reading Tree
Example
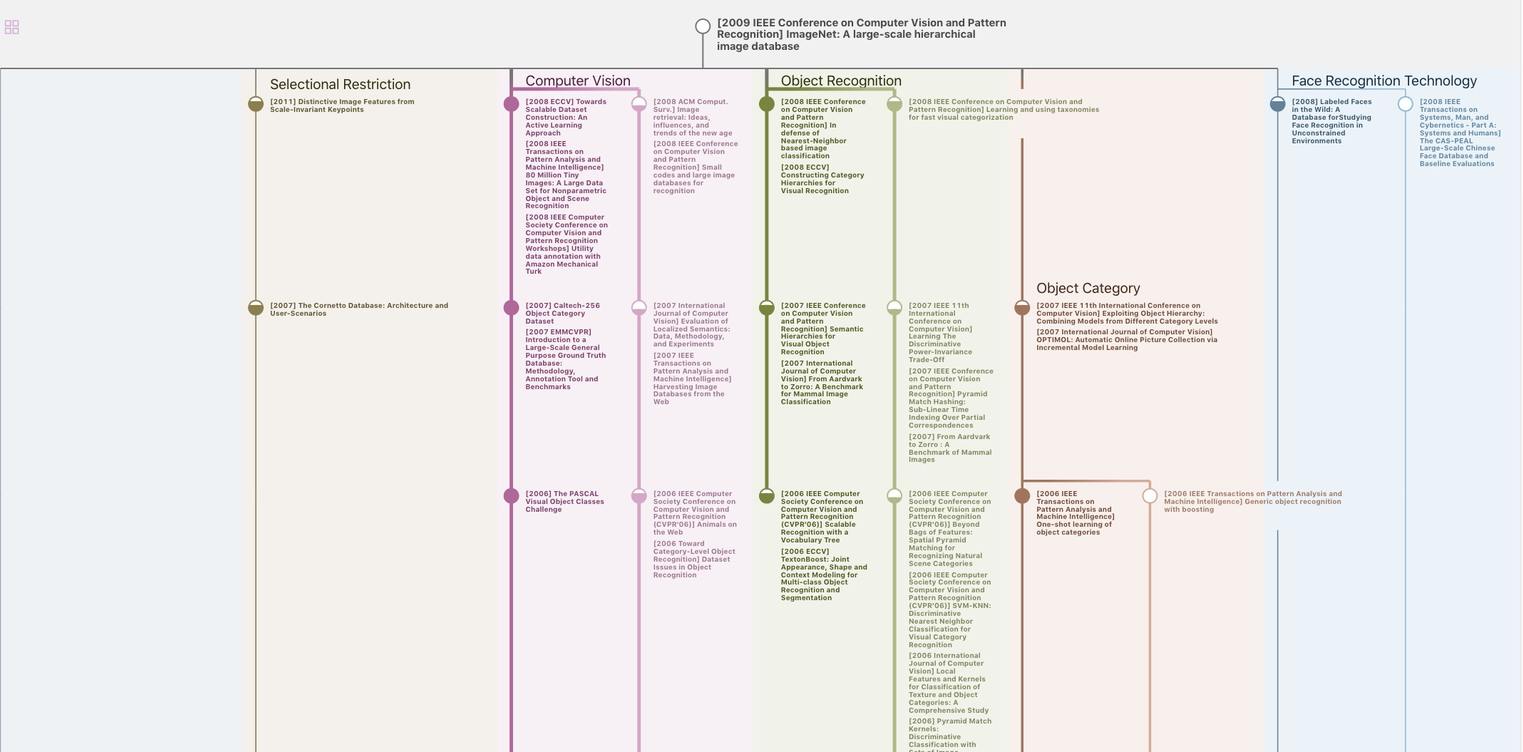
Generate MRT to find the research sequence of this paper
Chat Paper
Summary is being generated by the instructions you defined